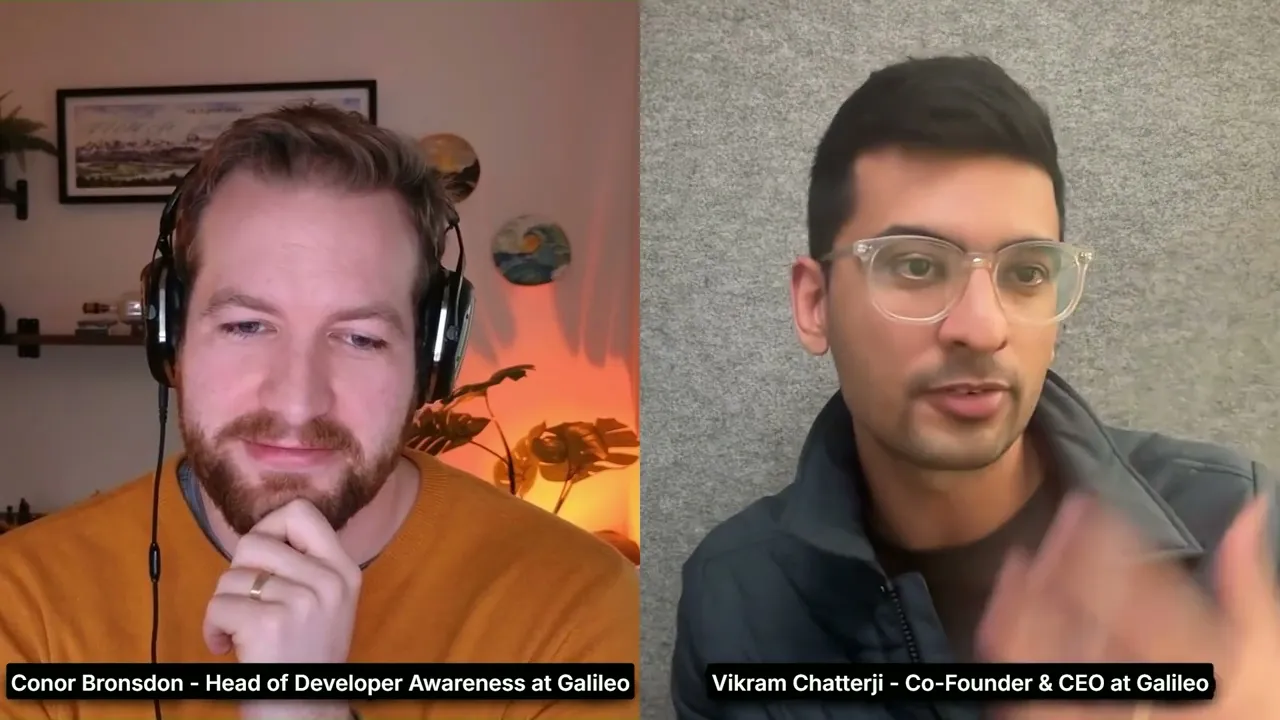
Apr 3, 2025
Comprehensive AI Evaluation: A Step-By-Step Approach to Maximize AI Potential


Conor Bronsdon
Head of Developer Awareness
Conor Bronsdon
Head of Developer Awareness


AI evaluation has become the make-or-break factor in AI implementation success. This rings especially true for generative AI, where traditional metrics simply can't capture the full picture. The stakes? Massive. Over 80% of AI projects crash and burn—twice the failure rate of non-AI IT projects.
This article explores step-by-step what it takes to properly evaluate AI systems and get them working successfully.
We recently explored this topic on our Chain of Thought podcast, where industry experts shared practical insights and real-world implementation strategies.
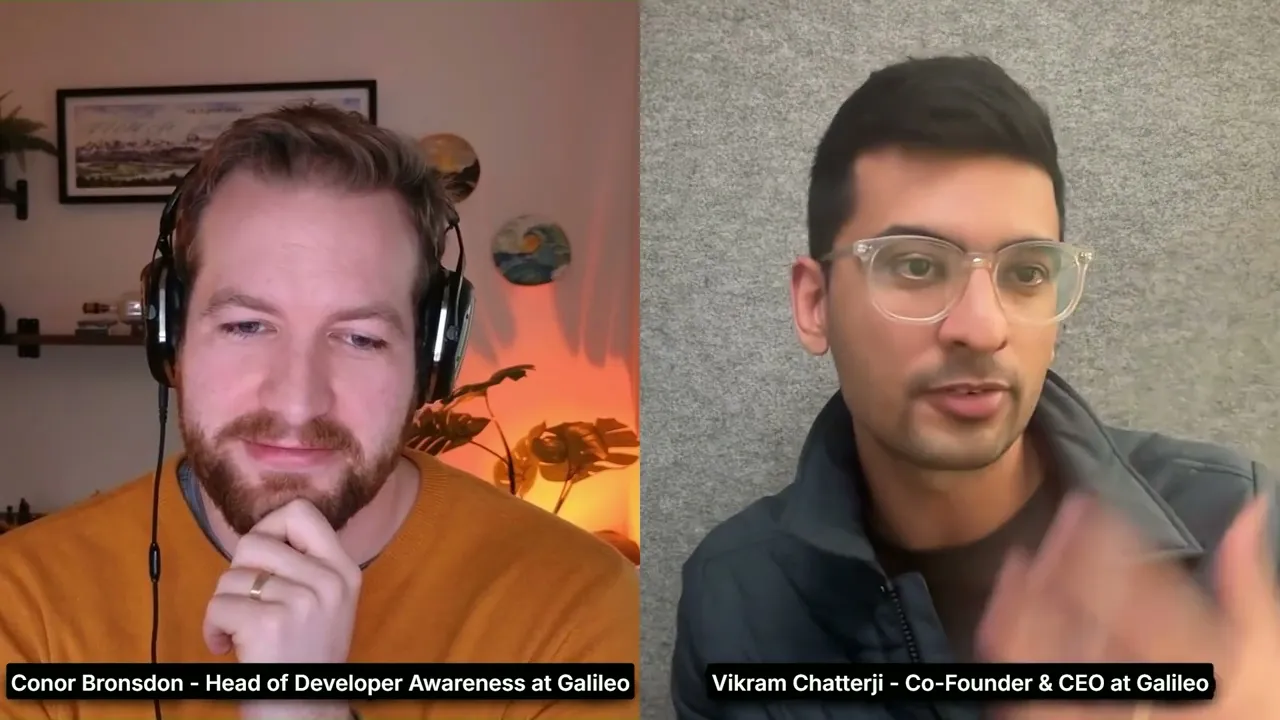
What is AI Evaluation?
AI evaluation is the systematic assessment of artificial intelligence systems to measure their performance, reliability, and alignment with intended objectives. Traditional ML models produce consistent outputs for identical inputs, but generative AI requires analyzing probabilistic outputs that can vary significantly even with the same prompts.
Traditional ML evaluation focuses on quantitative metrics like accuracy, precision, and recall using confusion matrices measured against ground truth. Generative AI demands a multidimensional approach examining output quality, creativity, ethical considerations, and alignment with human values.
Technical evaluation aims to identify model limitations, detect biases, measure performance across diverse tasks, and ensure safety. Business objectives focus on validating real-world problem-solving capabilities, measuring ROI, and ensuring regulatory compliance.
Modern evaluation combines computation-based metrics (ROUGE, BLEU) with model-based metrics that use judge models. This hybrid approach assesses both objective qualities and subjective dimensions like coherence and relevance.
The Importance of AI Evaluation in Enterprise Applications
Good evaluation is a crucial part of AI risk management, helping organizations avoid costly failures by catching potential risks early—data privacy violations, biased outputs, or hallucinations that could damage reputation or create legal liability.
Companies deploying financial advisor AI must rigorously check models for accuracy and regulatory compliance to prevent spreading misleading investment advice.
Beyond risk management, thorough AI evaluation creates competitive advantage. Organizations that properly evaluate their AI can optimize performance for specific use cases, driving higher adoption, better user satisfaction, and stronger ROI.
Effectively measuring AI ROI is crucial in regulated industries like healthcare and finance, where evaluation requirements are stricter and failure consequences more severe.
Evolution of AI Evaluation Methods
AI evaluation has transformed from simple accuracy metrics for early machine learning to sophisticated multi-dimensional frameworks for today's generative systems. Initially, evaluation relied on deterministic measures like precision, recall, and F1 scores comparing outputs against definitive ground truths.
The rise of large language models forced a shift toward probabilistic methods. Anthropic researchers pioneered innovative approaches like self-evaluation, where AI models participate in their own assessment, creating evaluations in minutes instead of days or months.
Constitutional AI (CAI) represents a milestone in this evolution, replacing human red teaming with model-based alternatives to improve output safety. This approach shows how AI evaluation has become integrated with training, creating a continuous feedback loop that drives improvement rather than just serving as a verification step.
These evaluation advancements set the foundation for the structured step-by-step approach detailed in the following steps, guiding teams through implementing comprehensive AI evaluation frameworks that address technical performance and business impact considerations.

AI Evaluation Step #1: Align AI Evaluation with Stakeholder Requirements
Effective AI evaluation requires balancing diverse priorities across your organization:
Start with methodical stakeholder interviews to capture these perspectives. Using approaches from implementation science, engage people across organizational levels for comprehensive insights. Structure your questions to be accessible while aligning with your evaluation framework.
Next, map requirements to create a unified view of evaluation needs. Document each stakeholder's priorities in a framework to identify where they overlap and conflict. This process often reveals tensions between technical, business, and ethical considerations.
When competing interests arise, try quantifying stakeholder priorities. Tools like the Analytic Hierarchy Process (AHP) can help assign relative importance to different evaluation criteria. This numerical approach clarifies necessary trade-offs and creates transparent decision-making processes.
Galileo streamlines this alignment with a unified evaluation platform supporting diverse perspectives. Its customizable dashboards translate complex AI metrics into actionable insights for everyone from engineers troubleshooting models to executives making strategic decisions.
Galileo further enables comprehensive requirement documentation through customizable evaluation templates that connect technical metrics with business objectives. Multi-level reporting features translate evaluation results into appropriate formats for different stakeholders, ensuring everyone gets the insights they need.
AI Evaluation Step #2: Implement Ground Truth Alternatives
Evaluating generative AI presents a fundamental challenge: unlike traditional ML models, there's often no definitive "correct answer" to compare outputs against. This absence of ground truth makes conventional metrics insufficient. We need practical alternatives to reliably assess AI-generated content quality.
Model-based evaluation, as part of an LLM evaluation framework, provides one powerful approach. When you use consensus methods, you can gather judgments from multiple models or evaluators to approximate ground truth. By looking at responses from various model architectures or prompting strategies, you'll find high-agreement areas that likely represent correct outputs. This approach helps reduce individual model biases, though it requires careful coordination.
With autonomous evaluation, you can implement self-consistency checks within your evaluation pipeline. Constitutional AI shows how model-based approaches can replace human red-teaming to improve harmlessness. You can check whether outputs are internally consistent, factually plausible, and follow predefined constraints—without needing external reference data.
Reference-augmented evaluation combines model reasoning with verified external information. Try prompting the model to evaluate itself by comparing outputs against trusted knowledge bases, creating a synthetic ground truth. This bridges the gap between pure model-based approaches and traditional reference-based evaluation.
To get the most robust results, orchestrate multiple evaluation strategies in parallel. Galileo automates this orchestration, letting you implement several alternatives simultaneously and integrate their results into a comprehensive evaluation framework.
AI Evaluation Step #3: Implement Evaluation Metrics
Selecting appropriate metrics forms the foundation of effective AI evaluation. Your metrics must align with your application context, risk profile, and business objectives. Technical performance metrics alone won't cut it—you need a comprehensive framework addressing all dimensions of your AI system's behavior.
Galileo offers a robust set of AI evaluation metrics across several key dimensions:
Quality and safety metrics go beyond raw performance. Ethical evaluation frameworks like the Foundation Model Transparency Index and IBM's AIX360 assess fairness, bias, and transparency—increasingly critical as AI makes consequential decisions and faces regulatory scrutiny.
Understanding trade-offs between competing metrics is essential. Better precision often comes at the cost of worse recall, and optimizing short-term performance might sacrifice long-term safety. Your validation strategy should reflect how the model will operate in production and what risks it poses.
Be clear about which metrics are non-negotiable versus flexible. This determination should flow directly from your earlier business requirements, regulatory constraints, and ethical commitments. Document these priorities clearly to guide development and evaluation cycles.
As AI systems evolve, tracking metrics consistently across experiments grows increasingly complex. Galileo provides a unified framework for defining, measuring, and monitoring diverse evaluation metrics throughout the AI lifecycle, keeping your systems aligned with your standards and goals while adapting to emerging challenges.
AI Evaluation Step #4: Analyze Patterns and Take Action
With evaluation metrics data collected, you need to translate results into actionable insights. Examine trends across multiple evaluation runs rather than focusing on isolated incidents. Look for recurring issues in specific domains, with particular prompt structures, or under certain conditions to identify systematic weaknesses.
Root cause analysis uncovers why your AI system fails. Common failure points include misunderstanding the initial problem, inadequate training data, focusing on technology over solutions, insufficient infrastructure, and overestimating AI capabilities. Identifying which factors affect your system helps target improvement efforts when evaluating generative AI.
Apply structured analytical methods for deeper insights. Try conducting comparative analyses between model versions, trend analyses to identify performance shifts over time, and correlation analyses connecting specific behaviors with performance metrics. These approaches pinpoint exactly where interventions are needed.
Develop a targeted intervention strategy based on findings. For minor issues, prompt engineering may suffice—refining instructions or adding guardrails for specific failure modes. For bigger problems, consider dataset augmentation to address identified gaps or fine-tuning approaches focusing on problematic areas while preserving general capabilities.
Advanced intervention might involve model architecture changes or developing specialized models for challenging domains. Create a prioritized improvement roadmap balancing quick wins with longer-term solutions. Implement changes incrementally and maintain your AI evaluation framework to measure each intervention's impact.
Maintaining a closed feedback loop between evaluation, analysis, and improvement drives continuous enhancement. Galileo streamlines this process by automatically identifying performance patterns, highlighting potential failure modes, and providing actionable recommendations without extensive manual analysis.
AI Evaluation Step #5: Set Up Effective Guardrails
After thoroughly evaluating your AI system, establishing appropriate guardrails ensures safe, reliable operation. Guardrails act as boundaries within which your AI can function while preventing harmful outputs. Design them based on evaluation insights, focusing on known failure modes and potential risks identified during testing.
Technical implementation typically involves setting confidence thresholds, creating filtering mechanisms, and designing intervention protocols. You might implement validation layers checking output consistency before presenting results to users. Configure your system to flag or block outputs where certainty falls below predetermined levels, particularly for high-stakes applications in healthcare, finance, or security.
Context-aware warning systems work better than generic disclaimers. Rather than showing constant warnings users eventually ignore, effective systems display warnings only when relevant to specific outputs. This might include using AI-generated confidence scores to determine when to show contextual warnings about potential inaccuracies.
Balancing restrictiveness with utility remains challenging. Overly strict guardrails limit functionality and frustrate users, while insufficient boundaries pose safety risks. Achieving this balance may require focusing on AI explainability to make guardrails transparent to users.
This balance must be calibrated contextually—what works for content generation differs dramatically from what's needed for autonomous systems or financial advice tools.
Your guardrails directly impact trust in your AI system and are a key component of trustworthy AI governance. Robust safety measures based on ISO/IEC standards ensure your system "does not under defined conditions, lead to a state in which human life, health, property, or the environment is endangered."
Galileo strengthens guardrail implementation through automatic detection of potential harmful outputs, drift monitoring systems that identify when AI responses deviate from safety parameters, and detailed feedback on which guardrails are triggered and why, enabling continuous refinement of protection mechanisms.
AI Evaluation Step #6: Establish Continuous Evaluation Workflows
AI models aren't "set it and forget it" solutions. Point-in-time evaluations quickly become outdated as data distributions shift, user behavior changes, and new edge cases emerge. Continuous evaluation throughout the AI lifecycle maintains model quality and reliability.
Implement continuous evaluation by integrating testing into your CI/CD pipelines. Automate model evaluation whenever code changes, new data arrives, or models are retrained. Performance degradation happens gradually, making it hard to detect without proper monitoring.
Data drift detection systems alert you when input distributions change significantly, while performance metrics tracking identifies subtle declines in accuracy, precision, recall, or latency. These should trigger automated evaluations when thresholds are crossed.
A/B testing lets you run two model versions in parallel and compare results, enabling data-driven update decisions without risking your entire system. This approach allows real-time performance comparison and incremental improvements.
Your continuous evaluation architecture should include automated retraining pipelines incorporating new data and user feedback. This creates a closed feedback loop where models constantly improve based on real-world performance. User feedback provides valuable insights into model performance and reveals opportunities for improving satisfaction and accuracy.
Galileo takes continuous AI evaluation further with comprehensive monitoring tools that integrate with existing workflows. You can automate evaluation triggers based on model updates or data shifts, track performance metrics across iterations, and gain actionable insights to guide your next development cycle.
Get Started With Galileo’s Comprehensive Platform for AI Evaluation
Effective AI evaluation requires addressing multiple challenges, including system complexity, standardized metrics, and ethical considerations. A structured approach is essential for deploying reliable, high-performing AI solutions. Galileo offers a comprehensive platform designed to address these evaluation requirements with precision and efficiency.
Galileo's platform provides end-to-end AI evaluation capabilities that help teams tackle the multifaceted challenges of AI assessment:
Get started with Galileo today to ensure your AI solutions meet the highest standards of performance, reliability, and ethical compliance.
AI evaluation has become the make-or-break factor in AI implementation success. This rings especially true for generative AI, where traditional metrics simply can't capture the full picture. The stakes? Massive. Over 80% of AI projects crash and burn—twice the failure rate of non-AI IT projects.
This article explores step-by-step what it takes to properly evaluate AI systems and get them working successfully.
We recently explored this topic on our Chain of Thought podcast, where industry experts shared practical insights and real-world implementation strategies.
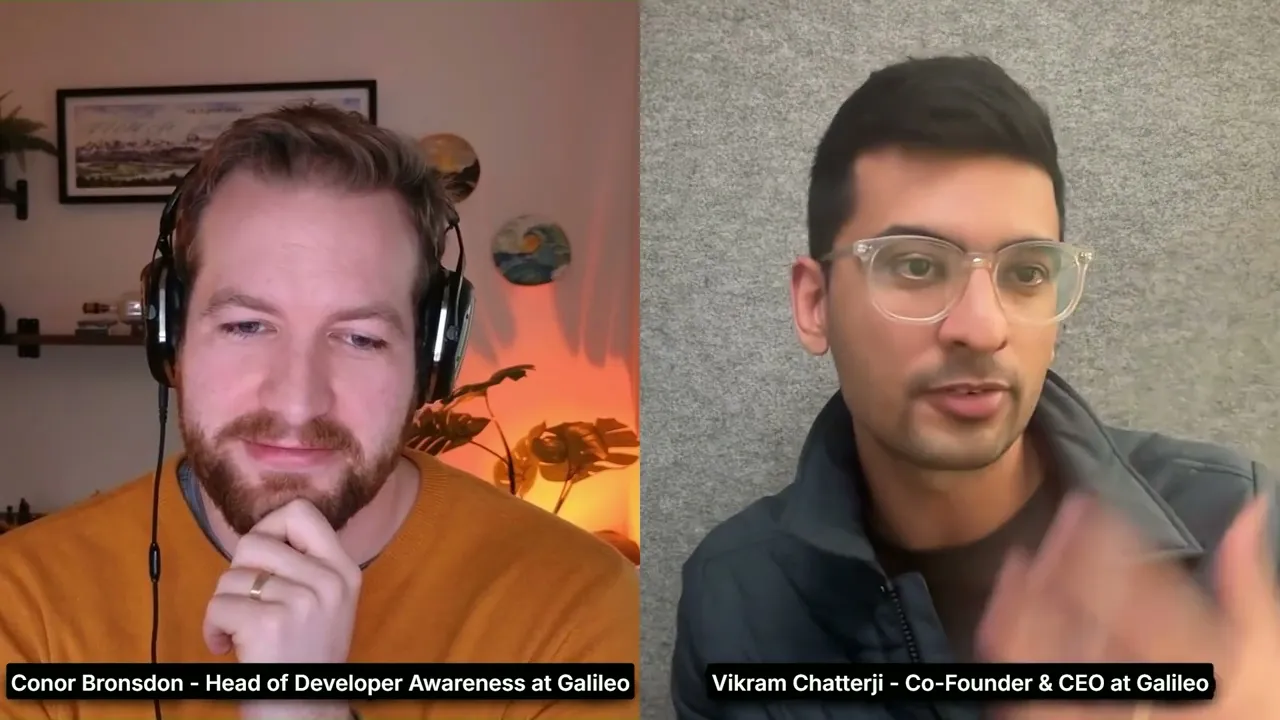
What is AI Evaluation?
AI evaluation is the systematic assessment of artificial intelligence systems to measure their performance, reliability, and alignment with intended objectives. Traditional ML models produce consistent outputs for identical inputs, but generative AI requires analyzing probabilistic outputs that can vary significantly even with the same prompts.
Traditional ML evaluation focuses on quantitative metrics like accuracy, precision, and recall using confusion matrices measured against ground truth. Generative AI demands a multidimensional approach examining output quality, creativity, ethical considerations, and alignment with human values.
Technical evaluation aims to identify model limitations, detect biases, measure performance across diverse tasks, and ensure safety. Business objectives focus on validating real-world problem-solving capabilities, measuring ROI, and ensuring regulatory compliance.
Modern evaluation combines computation-based metrics (ROUGE, BLEU) with model-based metrics that use judge models. This hybrid approach assesses both objective qualities and subjective dimensions like coherence and relevance.
The Importance of AI Evaluation in Enterprise Applications
Good evaluation is a crucial part of AI risk management, helping organizations avoid costly failures by catching potential risks early—data privacy violations, biased outputs, or hallucinations that could damage reputation or create legal liability.
Companies deploying financial advisor AI must rigorously check models for accuracy and regulatory compliance to prevent spreading misleading investment advice.
Beyond risk management, thorough AI evaluation creates competitive advantage. Organizations that properly evaluate their AI can optimize performance for specific use cases, driving higher adoption, better user satisfaction, and stronger ROI.
Effectively measuring AI ROI is crucial in regulated industries like healthcare and finance, where evaluation requirements are stricter and failure consequences more severe.
Evolution of AI Evaluation Methods
AI evaluation has transformed from simple accuracy metrics for early machine learning to sophisticated multi-dimensional frameworks for today's generative systems. Initially, evaluation relied on deterministic measures like precision, recall, and F1 scores comparing outputs against definitive ground truths.
The rise of large language models forced a shift toward probabilistic methods. Anthropic researchers pioneered innovative approaches like self-evaluation, where AI models participate in their own assessment, creating evaluations in minutes instead of days or months.
Constitutional AI (CAI) represents a milestone in this evolution, replacing human red teaming with model-based alternatives to improve output safety. This approach shows how AI evaluation has become integrated with training, creating a continuous feedback loop that drives improvement rather than just serving as a verification step.
These evaluation advancements set the foundation for the structured step-by-step approach detailed in the following steps, guiding teams through implementing comprehensive AI evaluation frameworks that address technical performance and business impact considerations.

AI Evaluation Step #1: Align AI Evaluation with Stakeholder Requirements
Effective AI evaluation requires balancing diverse priorities across your organization:
Start with methodical stakeholder interviews to capture these perspectives. Using approaches from implementation science, engage people across organizational levels for comprehensive insights. Structure your questions to be accessible while aligning with your evaluation framework.
Next, map requirements to create a unified view of evaluation needs. Document each stakeholder's priorities in a framework to identify where they overlap and conflict. This process often reveals tensions between technical, business, and ethical considerations.
When competing interests arise, try quantifying stakeholder priorities. Tools like the Analytic Hierarchy Process (AHP) can help assign relative importance to different evaluation criteria. This numerical approach clarifies necessary trade-offs and creates transparent decision-making processes.
Galileo streamlines this alignment with a unified evaluation platform supporting diverse perspectives. Its customizable dashboards translate complex AI metrics into actionable insights for everyone from engineers troubleshooting models to executives making strategic decisions.
Galileo further enables comprehensive requirement documentation through customizable evaluation templates that connect technical metrics with business objectives. Multi-level reporting features translate evaluation results into appropriate formats for different stakeholders, ensuring everyone gets the insights they need.
AI Evaluation Step #2: Implement Ground Truth Alternatives
Evaluating generative AI presents a fundamental challenge: unlike traditional ML models, there's often no definitive "correct answer" to compare outputs against. This absence of ground truth makes conventional metrics insufficient. We need practical alternatives to reliably assess AI-generated content quality.
Model-based evaluation, as part of an LLM evaluation framework, provides one powerful approach. When you use consensus methods, you can gather judgments from multiple models or evaluators to approximate ground truth. By looking at responses from various model architectures or prompting strategies, you'll find high-agreement areas that likely represent correct outputs. This approach helps reduce individual model biases, though it requires careful coordination.
With autonomous evaluation, you can implement self-consistency checks within your evaluation pipeline. Constitutional AI shows how model-based approaches can replace human red-teaming to improve harmlessness. You can check whether outputs are internally consistent, factually plausible, and follow predefined constraints—without needing external reference data.
Reference-augmented evaluation combines model reasoning with verified external information. Try prompting the model to evaluate itself by comparing outputs against trusted knowledge bases, creating a synthetic ground truth. This bridges the gap between pure model-based approaches and traditional reference-based evaluation.
To get the most robust results, orchestrate multiple evaluation strategies in parallel. Galileo automates this orchestration, letting you implement several alternatives simultaneously and integrate their results into a comprehensive evaluation framework.
AI Evaluation Step #3: Implement Evaluation Metrics
Selecting appropriate metrics forms the foundation of effective AI evaluation. Your metrics must align with your application context, risk profile, and business objectives. Technical performance metrics alone won't cut it—you need a comprehensive framework addressing all dimensions of your AI system's behavior.
Galileo offers a robust set of AI evaluation metrics across several key dimensions:
Quality and safety metrics go beyond raw performance. Ethical evaluation frameworks like the Foundation Model Transparency Index and IBM's AIX360 assess fairness, bias, and transparency—increasingly critical as AI makes consequential decisions and faces regulatory scrutiny.
Understanding trade-offs between competing metrics is essential. Better precision often comes at the cost of worse recall, and optimizing short-term performance might sacrifice long-term safety. Your validation strategy should reflect how the model will operate in production and what risks it poses.
Be clear about which metrics are non-negotiable versus flexible. This determination should flow directly from your earlier business requirements, regulatory constraints, and ethical commitments. Document these priorities clearly to guide development and evaluation cycles.
As AI systems evolve, tracking metrics consistently across experiments grows increasingly complex. Galileo provides a unified framework for defining, measuring, and monitoring diverse evaluation metrics throughout the AI lifecycle, keeping your systems aligned with your standards and goals while adapting to emerging challenges.
AI Evaluation Step #4: Analyze Patterns and Take Action
With evaluation metrics data collected, you need to translate results into actionable insights. Examine trends across multiple evaluation runs rather than focusing on isolated incidents. Look for recurring issues in specific domains, with particular prompt structures, or under certain conditions to identify systematic weaknesses.
Root cause analysis uncovers why your AI system fails. Common failure points include misunderstanding the initial problem, inadequate training data, focusing on technology over solutions, insufficient infrastructure, and overestimating AI capabilities. Identifying which factors affect your system helps target improvement efforts when evaluating generative AI.
Apply structured analytical methods for deeper insights. Try conducting comparative analyses between model versions, trend analyses to identify performance shifts over time, and correlation analyses connecting specific behaviors with performance metrics. These approaches pinpoint exactly where interventions are needed.
Develop a targeted intervention strategy based on findings. For minor issues, prompt engineering may suffice—refining instructions or adding guardrails for specific failure modes. For bigger problems, consider dataset augmentation to address identified gaps or fine-tuning approaches focusing on problematic areas while preserving general capabilities.
Advanced intervention might involve model architecture changes or developing specialized models for challenging domains. Create a prioritized improvement roadmap balancing quick wins with longer-term solutions. Implement changes incrementally and maintain your AI evaluation framework to measure each intervention's impact.
Maintaining a closed feedback loop between evaluation, analysis, and improvement drives continuous enhancement. Galileo streamlines this process by automatically identifying performance patterns, highlighting potential failure modes, and providing actionable recommendations without extensive manual analysis.
AI Evaluation Step #5: Set Up Effective Guardrails
After thoroughly evaluating your AI system, establishing appropriate guardrails ensures safe, reliable operation. Guardrails act as boundaries within which your AI can function while preventing harmful outputs. Design them based on evaluation insights, focusing on known failure modes and potential risks identified during testing.
Technical implementation typically involves setting confidence thresholds, creating filtering mechanisms, and designing intervention protocols. You might implement validation layers checking output consistency before presenting results to users. Configure your system to flag or block outputs where certainty falls below predetermined levels, particularly for high-stakes applications in healthcare, finance, or security.
Context-aware warning systems work better than generic disclaimers. Rather than showing constant warnings users eventually ignore, effective systems display warnings only when relevant to specific outputs. This might include using AI-generated confidence scores to determine when to show contextual warnings about potential inaccuracies.
Balancing restrictiveness with utility remains challenging. Overly strict guardrails limit functionality and frustrate users, while insufficient boundaries pose safety risks. Achieving this balance may require focusing on AI explainability to make guardrails transparent to users.
This balance must be calibrated contextually—what works for content generation differs dramatically from what's needed for autonomous systems or financial advice tools.
Your guardrails directly impact trust in your AI system and are a key component of trustworthy AI governance. Robust safety measures based on ISO/IEC standards ensure your system "does not under defined conditions, lead to a state in which human life, health, property, or the environment is endangered."
Galileo strengthens guardrail implementation through automatic detection of potential harmful outputs, drift monitoring systems that identify when AI responses deviate from safety parameters, and detailed feedback on which guardrails are triggered and why, enabling continuous refinement of protection mechanisms.
AI Evaluation Step #6: Establish Continuous Evaluation Workflows
AI models aren't "set it and forget it" solutions. Point-in-time evaluations quickly become outdated as data distributions shift, user behavior changes, and new edge cases emerge. Continuous evaluation throughout the AI lifecycle maintains model quality and reliability.
Implement continuous evaluation by integrating testing into your CI/CD pipelines. Automate model evaluation whenever code changes, new data arrives, or models are retrained. Performance degradation happens gradually, making it hard to detect without proper monitoring.
Data drift detection systems alert you when input distributions change significantly, while performance metrics tracking identifies subtle declines in accuracy, precision, recall, or latency. These should trigger automated evaluations when thresholds are crossed.
A/B testing lets you run two model versions in parallel and compare results, enabling data-driven update decisions without risking your entire system. This approach allows real-time performance comparison and incremental improvements.
Your continuous evaluation architecture should include automated retraining pipelines incorporating new data and user feedback. This creates a closed feedback loop where models constantly improve based on real-world performance. User feedback provides valuable insights into model performance and reveals opportunities for improving satisfaction and accuracy.
Galileo takes continuous AI evaluation further with comprehensive monitoring tools that integrate with existing workflows. You can automate evaluation triggers based on model updates or data shifts, track performance metrics across iterations, and gain actionable insights to guide your next development cycle.
Get Started With Galileo’s Comprehensive Platform for AI Evaluation
Effective AI evaluation requires addressing multiple challenges, including system complexity, standardized metrics, and ethical considerations. A structured approach is essential for deploying reliable, high-performing AI solutions. Galileo offers a comprehensive platform designed to address these evaluation requirements with precision and efficiency.
Galileo's platform provides end-to-end AI evaluation capabilities that help teams tackle the multifaceted challenges of AI assessment:
Get started with Galileo today to ensure your AI solutions meet the highest standards of performance, reliability, and ethical compliance.
AI evaluation has become the make-or-break factor in AI implementation success. This rings especially true for generative AI, where traditional metrics simply can't capture the full picture. The stakes? Massive. Over 80% of AI projects crash and burn—twice the failure rate of non-AI IT projects.
This article explores step-by-step what it takes to properly evaluate AI systems and get them working successfully.
We recently explored this topic on our Chain of Thought podcast, where industry experts shared practical insights and real-world implementation strategies.
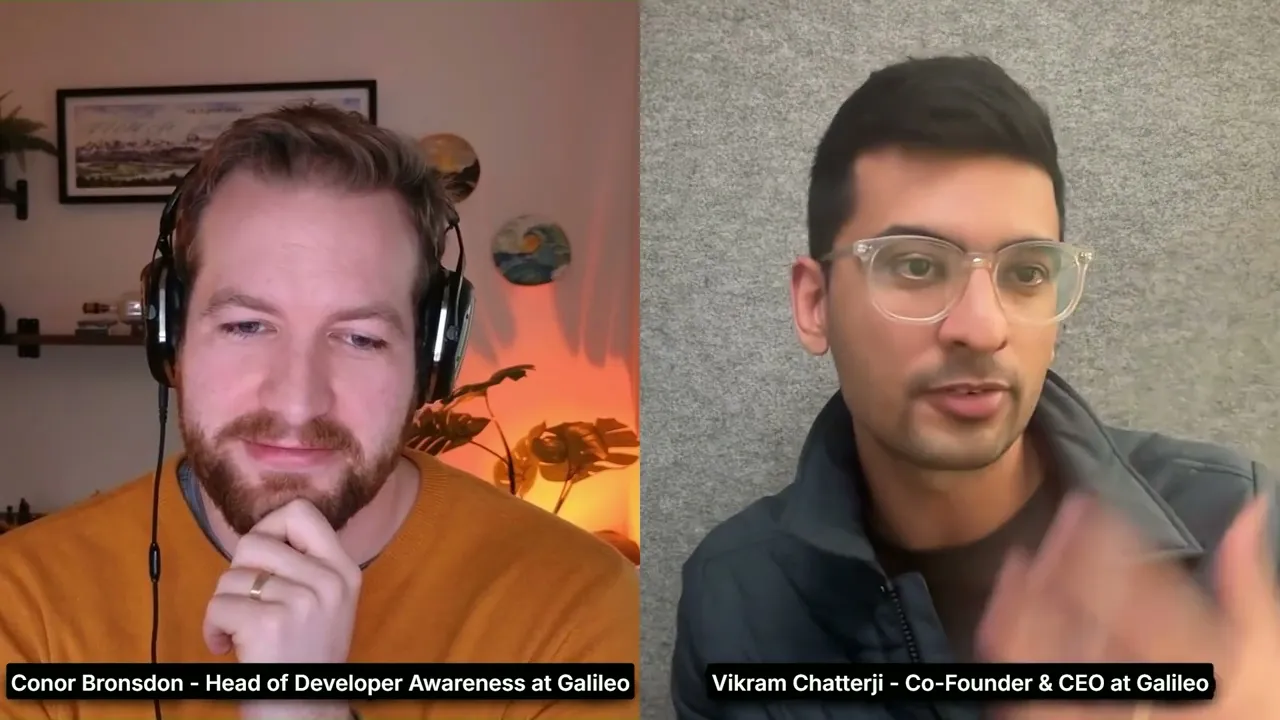
What is AI Evaluation?
AI evaluation is the systematic assessment of artificial intelligence systems to measure their performance, reliability, and alignment with intended objectives. Traditional ML models produce consistent outputs for identical inputs, but generative AI requires analyzing probabilistic outputs that can vary significantly even with the same prompts.
Traditional ML evaluation focuses on quantitative metrics like accuracy, precision, and recall using confusion matrices measured against ground truth. Generative AI demands a multidimensional approach examining output quality, creativity, ethical considerations, and alignment with human values.
Technical evaluation aims to identify model limitations, detect biases, measure performance across diverse tasks, and ensure safety. Business objectives focus on validating real-world problem-solving capabilities, measuring ROI, and ensuring regulatory compliance.
Modern evaluation combines computation-based metrics (ROUGE, BLEU) with model-based metrics that use judge models. This hybrid approach assesses both objective qualities and subjective dimensions like coherence and relevance.
The Importance of AI Evaluation in Enterprise Applications
Good evaluation is a crucial part of AI risk management, helping organizations avoid costly failures by catching potential risks early—data privacy violations, biased outputs, or hallucinations that could damage reputation or create legal liability.
Companies deploying financial advisor AI must rigorously check models for accuracy and regulatory compliance to prevent spreading misleading investment advice.
Beyond risk management, thorough AI evaluation creates competitive advantage. Organizations that properly evaluate their AI can optimize performance for specific use cases, driving higher adoption, better user satisfaction, and stronger ROI.
Effectively measuring AI ROI is crucial in regulated industries like healthcare and finance, where evaluation requirements are stricter and failure consequences more severe.
Evolution of AI Evaluation Methods
AI evaluation has transformed from simple accuracy metrics for early machine learning to sophisticated multi-dimensional frameworks for today's generative systems. Initially, evaluation relied on deterministic measures like precision, recall, and F1 scores comparing outputs against definitive ground truths.
The rise of large language models forced a shift toward probabilistic methods. Anthropic researchers pioneered innovative approaches like self-evaluation, where AI models participate in their own assessment, creating evaluations in minutes instead of days or months.
Constitutional AI (CAI) represents a milestone in this evolution, replacing human red teaming with model-based alternatives to improve output safety. This approach shows how AI evaluation has become integrated with training, creating a continuous feedback loop that drives improvement rather than just serving as a verification step.
These evaluation advancements set the foundation for the structured step-by-step approach detailed in the following steps, guiding teams through implementing comprehensive AI evaluation frameworks that address technical performance and business impact considerations.

AI Evaluation Step #1: Align AI Evaluation with Stakeholder Requirements
Effective AI evaluation requires balancing diverse priorities across your organization:
Start with methodical stakeholder interviews to capture these perspectives. Using approaches from implementation science, engage people across organizational levels for comprehensive insights. Structure your questions to be accessible while aligning with your evaluation framework.
Next, map requirements to create a unified view of evaluation needs. Document each stakeholder's priorities in a framework to identify where they overlap and conflict. This process often reveals tensions between technical, business, and ethical considerations.
When competing interests arise, try quantifying stakeholder priorities. Tools like the Analytic Hierarchy Process (AHP) can help assign relative importance to different evaluation criteria. This numerical approach clarifies necessary trade-offs and creates transparent decision-making processes.
Galileo streamlines this alignment with a unified evaluation platform supporting diverse perspectives. Its customizable dashboards translate complex AI metrics into actionable insights for everyone from engineers troubleshooting models to executives making strategic decisions.
Galileo further enables comprehensive requirement documentation through customizable evaluation templates that connect technical metrics with business objectives. Multi-level reporting features translate evaluation results into appropriate formats for different stakeholders, ensuring everyone gets the insights they need.
AI Evaluation Step #2: Implement Ground Truth Alternatives
Evaluating generative AI presents a fundamental challenge: unlike traditional ML models, there's often no definitive "correct answer" to compare outputs against. This absence of ground truth makes conventional metrics insufficient. We need practical alternatives to reliably assess AI-generated content quality.
Model-based evaluation, as part of an LLM evaluation framework, provides one powerful approach. When you use consensus methods, you can gather judgments from multiple models or evaluators to approximate ground truth. By looking at responses from various model architectures or prompting strategies, you'll find high-agreement areas that likely represent correct outputs. This approach helps reduce individual model biases, though it requires careful coordination.
With autonomous evaluation, you can implement self-consistency checks within your evaluation pipeline. Constitutional AI shows how model-based approaches can replace human red-teaming to improve harmlessness. You can check whether outputs are internally consistent, factually plausible, and follow predefined constraints—without needing external reference data.
Reference-augmented evaluation combines model reasoning with verified external information. Try prompting the model to evaluate itself by comparing outputs against trusted knowledge bases, creating a synthetic ground truth. This bridges the gap between pure model-based approaches and traditional reference-based evaluation.
To get the most robust results, orchestrate multiple evaluation strategies in parallel. Galileo automates this orchestration, letting you implement several alternatives simultaneously and integrate their results into a comprehensive evaluation framework.
AI Evaluation Step #3: Implement Evaluation Metrics
Selecting appropriate metrics forms the foundation of effective AI evaluation. Your metrics must align with your application context, risk profile, and business objectives. Technical performance metrics alone won't cut it—you need a comprehensive framework addressing all dimensions of your AI system's behavior.
Galileo offers a robust set of AI evaluation metrics across several key dimensions:
Quality and safety metrics go beyond raw performance. Ethical evaluation frameworks like the Foundation Model Transparency Index and IBM's AIX360 assess fairness, bias, and transparency—increasingly critical as AI makes consequential decisions and faces regulatory scrutiny.
Understanding trade-offs between competing metrics is essential. Better precision often comes at the cost of worse recall, and optimizing short-term performance might sacrifice long-term safety. Your validation strategy should reflect how the model will operate in production and what risks it poses.
Be clear about which metrics are non-negotiable versus flexible. This determination should flow directly from your earlier business requirements, regulatory constraints, and ethical commitments. Document these priorities clearly to guide development and evaluation cycles.
As AI systems evolve, tracking metrics consistently across experiments grows increasingly complex. Galileo provides a unified framework for defining, measuring, and monitoring diverse evaluation metrics throughout the AI lifecycle, keeping your systems aligned with your standards and goals while adapting to emerging challenges.
AI Evaluation Step #4: Analyze Patterns and Take Action
With evaluation metrics data collected, you need to translate results into actionable insights. Examine trends across multiple evaluation runs rather than focusing on isolated incidents. Look for recurring issues in specific domains, with particular prompt structures, or under certain conditions to identify systematic weaknesses.
Root cause analysis uncovers why your AI system fails. Common failure points include misunderstanding the initial problem, inadequate training data, focusing on technology over solutions, insufficient infrastructure, and overestimating AI capabilities. Identifying which factors affect your system helps target improvement efforts when evaluating generative AI.
Apply structured analytical methods for deeper insights. Try conducting comparative analyses between model versions, trend analyses to identify performance shifts over time, and correlation analyses connecting specific behaviors with performance metrics. These approaches pinpoint exactly where interventions are needed.
Develop a targeted intervention strategy based on findings. For minor issues, prompt engineering may suffice—refining instructions or adding guardrails for specific failure modes. For bigger problems, consider dataset augmentation to address identified gaps or fine-tuning approaches focusing on problematic areas while preserving general capabilities.
Advanced intervention might involve model architecture changes or developing specialized models for challenging domains. Create a prioritized improvement roadmap balancing quick wins with longer-term solutions. Implement changes incrementally and maintain your AI evaluation framework to measure each intervention's impact.
Maintaining a closed feedback loop between evaluation, analysis, and improvement drives continuous enhancement. Galileo streamlines this process by automatically identifying performance patterns, highlighting potential failure modes, and providing actionable recommendations without extensive manual analysis.
AI Evaluation Step #5: Set Up Effective Guardrails
After thoroughly evaluating your AI system, establishing appropriate guardrails ensures safe, reliable operation. Guardrails act as boundaries within which your AI can function while preventing harmful outputs. Design them based on evaluation insights, focusing on known failure modes and potential risks identified during testing.
Technical implementation typically involves setting confidence thresholds, creating filtering mechanisms, and designing intervention protocols. You might implement validation layers checking output consistency before presenting results to users. Configure your system to flag or block outputs where certainty falls below predetermined levels, particularly for high-stakes applications in healthcare, finance, or security.
Context-aware warning systems work better than generic disclaimers. Rather than showing constant warnings users eventually ignore, effective systems display warnings only when relevant to specific outputs. This might include using AI-generated confidence scores to determine when to show contextual warnings about potential inaccuracies.
Balancing restrictiveness with utility remains challenging. Overly strict guardrails limit functionality and frustrate users, while insufficient boundaries pose safety risks. Achieving this balance may require focusing on AI explainability to make guardrails transparent to users.
This balance must be calibrated contextually—what works for content generation differs dramatically from what's needed for autonomous systems or financial advice tools.
Your guardrails directly impact trust in your AI system and are a key component of trustworthy AI governance. Robust safety measures based on ISO/IEC standards ensure your system "does not under defined conditions, lead to a state in which human life, health, property, or the environment is endangered."
Galileo strengthens guardrail implementation through automatic detection of potential harmful outputs, drift monitoring systems that identify when AI responses deviate from safety parameters, and detailed feedback on which guardrails are triggered and why, enabling continuous refinement of protection mechanisms.
AI Evaluation Step #6: Establish Continuous Evaluation Workflows
AI models aren't "set it and forget it" solutions. Point-in-time evaluations quickly become outdated as data distributions shift, user behavior changes, and new edge cases emerge. Continuous evaluation throughout the AI lifecycle maintains model quality and reliability.
Implement continuous evaluation by integrating testing into your CI/CD pipelines. Automate model evaluation whenever code changes, new data arrives, or models are retrained. Performance degradation happens gradually, making it hard to detect without proper monitoring.
Data drift detection systems alert you when input distributions change significantly, while performance metrics tracking identifies subtle declines in accuracy, precision, recall, or latency. These should trigger automated evaluations when thresholds are crossed.
A/B testing lets you run two model versions in parallel and compare results, enabling data-driven update decisions without risking your entire system. This approach allows real-time performance comparison and incremental improvements.
Your continuous evaluation architecture should include automated retraining pipelines incorporating new data and user feedback. This creates a closed feedback loop where models constantly improve based on real-world performance. User feedback provides valuable insights into model performance and reveals opportunities for improving satisfaction and accuracy.
Galileo takes continuous AI evaluation further with comprehensive monitoring tools that integrate with existing workflows. You can automate evaluation triggers based on model updates or data shifts, track performance metrics across iterations, and gain actionable insights to guide your next development cycle.
Get Started With Galileo’s Comprehensive Platform for AI Evaluation
Effective AI evaluation requires addressing multiple challenges, including system complexity, standardized metrics, and ethical considerations. A structured approach is essential for deploying reliable, high-performing AI solutions. Galileo offers a comprehensive platform designed to address these evaluation requirements with precision and efficiency.
Galileo's platform provides end-to-end AI evaluation capabilities that help teams tackle the multifaceted challenges of AI assessment:
Get started with Galileo today to ensure your AI solutions meet the highest standards of performance, reliability, and ethical compliance.
AI evaluation has become the make-or-break factor in AI implementation success. This rings especially true for generative AI, where traditional metrics simply can't capture the full picture. The stakes? Massive. Over 80% of AI projects crash and burn—twice the failure rate of non-AI IT projects.
This article explores step-by-step what it takes to properly evaluate AI systems and get them working successfully.
We recently explored this topic on our Chain of Thought podcast, where industry experts shared practical insights and real-world implementation strategies.
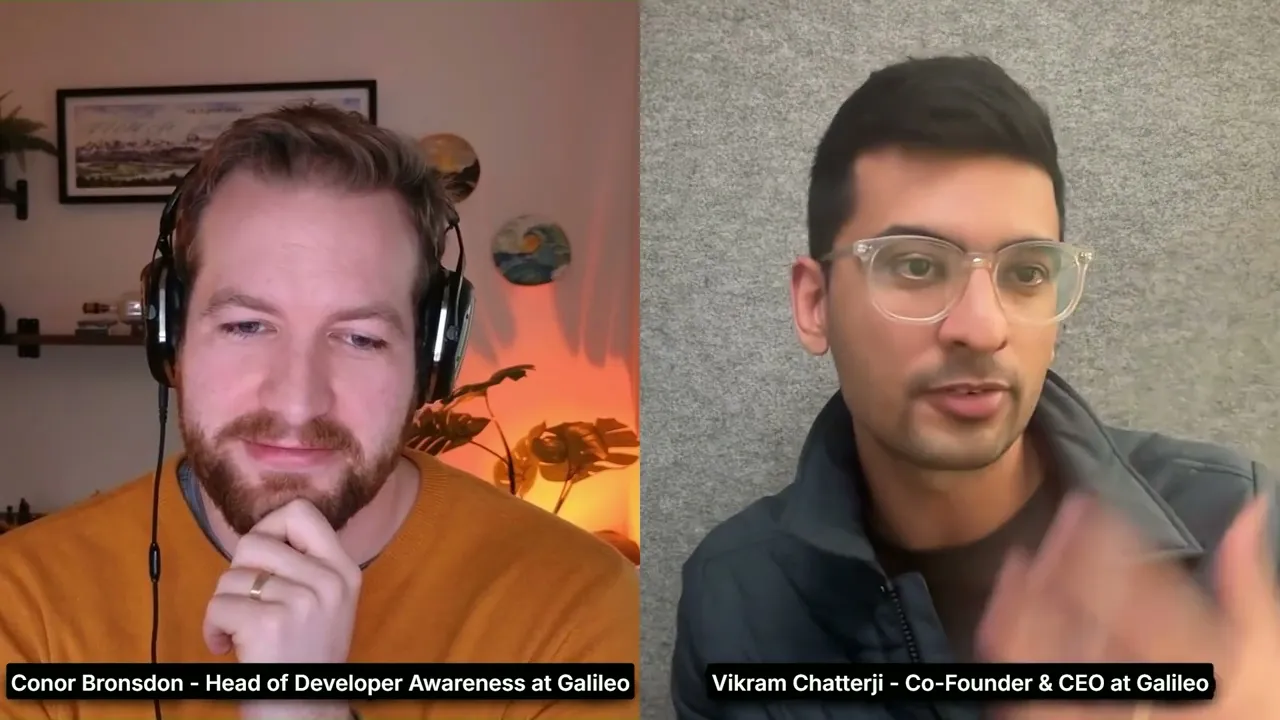
What is AI Evaluation?
AI evaluation is the systematic assessment of artificial intelligence systems to measure their performance, reliability, and alignment with intended objectives. Traditional ML models produce consistent outputs for identical inputs, but generative AI requires analyzing probabilistic outputs that can vary significantly even with the same prompts.
Traditional ML evaluation focuses on quantitative metrics like accuracy, precision, and recall using confusion matrices measured against ground truth. Generative AI demands a multidimensional approach examining output quality, creativity, ethical considerations, and alignment with human values.
Technical evaluation aims to identify model limitations, detect biases, measure performance across diverse tasks, and ensure safety. Business objectives focus on validating real-world problem-solving capabilities, measuring ROI, and ensuring regulatory compliance.
Modern evaluation combines computation-based metrics (ROUGE, BLEU) with model-based metrics that use judge models. This hybrid approach assesses both objective qualities and subjective dimensions like coherence and relevance.
The Importance of AI Evaluation in Enterprise Applications
Good evaluation is a crucial part of AI risk management, helping organizations avoid costly failures by catching potential risks early—data privacy violations, biased outputs, or hallucinations that could damage reputation or create legal liability.
Companies deploying financial advisor AI must rigorously check models for accuracy and regulatory compliance to prevent spreading misleading investment advice.
Beyond risk management, thorough AI evaluation creates competitive advantage. Organizations that properly evaluate their AI can optimize performance for specific use cases, driving higher adoption, better user satisfaction, and stronger ROI.
Effectively measuring AI ROI is crucial in regulated industries like healthcare and finance, where evaluation requirements are stricter and failure consequences more severe.
Evolution of AI Evaluation Methods
AI evaluation has transformed from simple accuracy metrics for early machine learning to sophisticated multi-dimensional frameworks for today's generative systems. Initially, evaluation relied on deterministic measures like precision, recall, and F1 scores comparing outputs against definitive ground truths.
The rise of large language models forced a shift toward probabilistic methods. Anthropic researchers pioneered innovative approaches like self-evaluation, where AI models participate in their own assessment, creating evaluations in minutes instead of days or months.
Constitutional AI (CAI) represents a milestone in this evolution, replacing human red teaming with model-based alternatives to improve output safety. This approach shows how AI evaluation has become integrated with training, creating a continuous feedback loop that drives improvement rather than just serving as a verification step.
These evaluation advancements set the foundation for the structured step-by-step approach detailed in the following steps, guiding teams through implementing comprehensive AI evaluation frameworks that address technical performance and business impact considerations.

AI Evaluation Step #1: Align AI Evaluation with Stakeholder Requirements
Effective AI evaluation requires balancing diverse priorities across your organization:
Start with methodical stakeholder interviews to capture these perspectives. Using approaches from implementation science, engage people across organizational levels for comprehensive insights. Structure your questions to be accessible while aligning with your evaluation framework.
Next, map requirements to create a unified view of evaluation needs. Document each stakeholder's priorities in a framework to identify where they overlap and conflict. This process often reveals tensions between technical, business, and ethical considerations.
When competing interests arise, try quantifying stakeholder priorities. Tools like the Analytic Hierarchy Process (AHP) can help assign relative importance to different evaluation criteria. This numerical approach clarifies necessary trade-offs and creates transparent decision-making processes.
Galileo streamlines this alignment with a unified evaluation platform supporting diverse perspectives. Its customizable dashboards translate complex AI metrics into actionable insights for everyone from engineers troubleshooting models to executives making strategic decisions.
Galileo further enables comprehensive requirement documentation through customizable evaluation templates that connect technical metrics with business objectives. Multi-level reporting features translate evaluation results into appropriate formats for different stakeholders, ensuring everyone gets the insights they need.
AI Evaluation Step #2: Implement Ground Truth Alternatives
Evaluating generative AI presents a fundamental challenge: unlike traditional ML models, there's often no definitive "correct answer" to compare outputs against. This absence of ground truth makes conventional metrics insufficient. We need practical alternatives to reliably assess AI-generated content quality.
Model-based evaluation, as part of an LLM evaluation framework, provides one powerful approach. When you use consensus methods, you can gather judgments from multiple models or evaluators to approximate ground truth. By looking at responses from various model architectures or prompting strategies, you'll find high-agreement areas that likely represent correct outputs. This approach helps reduce individual model biases, though it requires careful coordination.
With autonomous evaluation, you can implement self-consistency checks within your evaluation pipeline. Constitutional AI shows how model-based approaches can replace human red-teaming to improve harmlessness. You can check whether outputs are internally consistent, factually plausible, and follow predefined constraints—without needing external reference data.
Reference-augmented evaluation combines model reasoning with verified external information. Try prompting the model to evaluate itself by comparing outputs against trusted knowledge bases, creating a synthetic ground truth. This bridges the gap between pure model-based approaches and traditional reference-based evaluation.
To get the most robust results, orchestrate multiple evaluation strategies in parallel. Galileo automates this orchestration, letting you implement several alternatives simultaneously and integrate their results into a comprehensive evaluation framework.
AI Evaluation Step #3: Implement Evaluation Metrics
Selecting appropriate metrics forms the foundation of effective AI evaluation. Your metrics must align with your application context, risk profile, and business objectives. Technical performance metrics alone won't cut it—you need a comprehensive framework addressing all dimensions of your AI system's behavior.
Galileo offers a robust set of AI evaluation metrics across several key dimensions:
Quality and safety metrics go beyond raw performance. Ethical evaluation frameworks like the Foundation Model Transparency Index and IBM's AIX360 assess fairness, bias, and transparency—increasingly critical as AI makes consequential decisions and faces regulatory scrutiny.
Understanding trade-offs between competing metrics is essential. Better precision often comes at the cost of worse recall, and optimizing short-term performance might sacrifice long-term safety. Your validation strategy should reflect how the model will operate in production and what risks it poses.
Be clear about which metrics are non-negotiable versus flexible. This determination should flow directly from your earlier business requirements, regulatory constraints, and ethical commitments. Document these priorities clearly to guide development and evaluation cycles.
As AI systems evolve, tracking metrics consistently across experiments grows increasingly complex. Galileo provides a unified framework for defining, measuring, and monitoring diverse evaluation metrics throughout the AI lifecycle, keeping your systems aligned with your standards and goals while adapting to emerging challenges.
AI Evaluation Step #4: Analyze Patterns and Take Action
With evaluation metrics data collected, you need to translate results into actionable insights. Examine trends across multiple evaluation runs rather than focusing on isolated incidents. Look for recurring issues in specific domains, with particular prompt structures, or under certain conditions to identify systematic weaknesses.
Root cause analysis uncovers why your AI system fails. Common failure points include misunderstanding the initial problem, inadequate training data, focusing on technology over solutions, insufficient infrastructure, and overestimating AI capabilities. Identifying which factors affect your system helps target improvement efforts when evaluating generative AI.
Apply structured analytical methods for deeper insights. Try conducting comparative analyses between model versions, trend analyses to identify performance shifts over time, and correlation analyses connecting specific behaviors with performance metrics. These approaches pinpoint exactly where interventions are needed.
Develop a targeted intervention strategy based on findings. For minor issues, prompt engineering may suffice—refining instructions or adding guardrails for specific failure modes. For bigger problems, consider dataset augmentation to address identified gaps or fine-tuning approaches focusing on problematic areas while preserving general capabilities.
Advanced intervention might involve model architecture changes or developing specialized models for challenging domains. Create a prioritized improvement roadmap balancing quick wins with longer-term solutions. Implement changes incrementally and maintain your AI evaluation framework to measure each intervention's impact.
Maintaining a closed feedback loop between evaluation, analysis, and improvement drives continuous enhancement. Galileo streamlines this process by automatically identifying performance patterns, highlighting potential failure modes, and providing actionable recommendations without extensive manual analysis.
AI Evaluation Step #5: Set Up Effective Guardrails
After thoroughly evaluating your AI system, establishing appropriate guardrails ensures safe, reliable operation. Guardrails act as boundaries within which your AI can function while preventing harmful outputs. Design them based on evaluation insights, focusing on known failure modes and potential risks identified during testing.
Technical implementation typically involves setting confidence thresholds, creating filtering mechanisms, and designing intervention protocols. You might implement validation layers checking output consistency before presenting results to users. Configure your system to flag or block outputs where certainty falls below predetermined levels, particularly for high-stakes applications in healthcare, finance, or security.
Context-aware warning systems work better than generic disclaimers. Rather than showing constant warnings users eventually ignore, effective systems display warnings only when relevant to specific outputs. This might include using AI-generated confidence scores to determine when to show contextual warnings about potential inaccuracies.
Balancing restrictiveness with utility remains challenging. Overly strict guardrails limit functionality and frustrate users, while insufficient boundaries pose safety risks. Achieving this balance may require focusing on AI explainability to make guardrails transparent to users.
This balance must be calibrated contextually—what works for content generation differs dramatically from what's needed for autonomous systems or financial advice tools.
Your guardrails directly impact trust in your AI system and are a key component of trustworthy AI governance. Robust safety measures based on ISO/IEC standards ensure your system "does not under defined conditions, lead to a state in which human life, health, property, or the environment is endangered."
Galileo strengthens guardrail implementation through automatic detection of potential harmful outputs, drift monitoring systems that identify when AI responses deviate from safety parameters, and detailed feedback on which guardrails are triggered and why, enabling continuous refinement of protection mechanisms.
AI Evaluation Step #6: Establish Continuous Evaluation Workflows
AI models aren't "set it and forget it" solutions. Point-in-time evaluations quickly become outdated as data distributions shift, user behavior changes, and new edge cases emerge. Continuous evaluation throughout the AI lifecycle maintains model quality and reliability.
Implement continuous evaluation by integrating testing into your CI/CD pipelines. Automate model evaluation whenever code changes, new data arrives, or models are retrained. Performance degradation happens gradually, making it hard to detect without proper monitoring.
Data drift detection systems alert you when input distributions change significantly, while performance metrics tracking identifies subtle declines in accuracy, precision, recall, or latency. These should trigger automated evaluations when thresholds are crossed.
A/B testing lets you run two model versions in parallel and compare results, enabling data-driven update decisions without risking your entire system. This approach allows real-time performance comparison and incremental improvements.
Your continuous evaluation architecture should include automated retraining pipelines incorporating new data and user feedback. This creates a closed feedback loop where models constantly improve based on real-world performance. User feedback provides valuable insights into model performance and reveals opportunities for improving satisfaction and accuracy.
Galileo takes continuous AI evaluation further with comprehensive monitoring tools that integrate with existing workflows. You can automate evaluation triggers based on model updates or data shifts, track performance metrics across iterations, and gain actionable insights to guide your next development cycle.
Get Started With Galileo’s Comprehensive Platform for AI Evaluation
Effective AI evaluation requires addressing multiple challenges, including system complexity, standardized metrics, and ethical considerations. A structured approach is essential for deploying reliable, high-performing AI solutions. Galileo offers a comprehensive platform designed to address these evaluation requirements with precision and efficiency.
Galileo's platform provides end-to-end AI evaluation capabilities that help teams tackle the multifaceted challenges of AI assessment:
Get started with Galileo today to ensure your AI solutions meet the highest standards of performance, reliability, and ethical compliance.
Conor Bronsdon
Conor Bronsdon
Conor Bronsdon
Conor Bronsdon