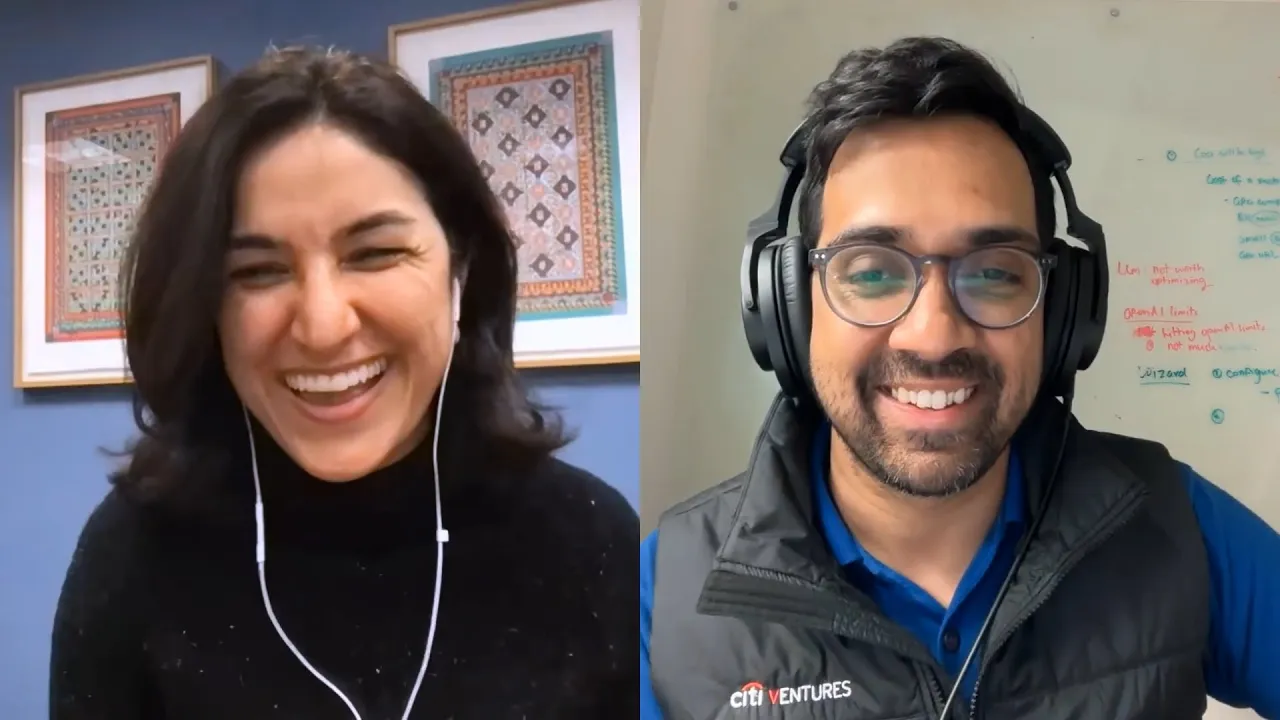
Apr 20, 2025
The 7-Step Framework for Effective AI Governance


Conor Bronsdon
Head of Developer Awareness
Conor Bronsdon
Head of Developer Awareness


A single AI system gone wrong can devastate many processes overnight. Without proper AI governance, companies face serious threats, from biased algorithms alienating customers to models leaking sensitive data, triggering penalties, and destroying trust.
Stakes are rising as enforcement tightens under frameworks like the EU AI Act, with fines reaching €35M or 7% of annual revenue. Organizations now face a clear challenge: unlock AI's benefits while ensuring responsible use. Without proper AI governance, AI systems can cause regulatory penalties, reputation damage, biased outcomes, and wasted resources.
This article explores seven key steps for organizations to implement effective, well-governed AI that delivers better results, accelerates innovation, and builds stakeholder confidence.
We recently explored this topic on our Chain of Thought podcast, where industry experts shared practical insights and real-world implementation strategies.
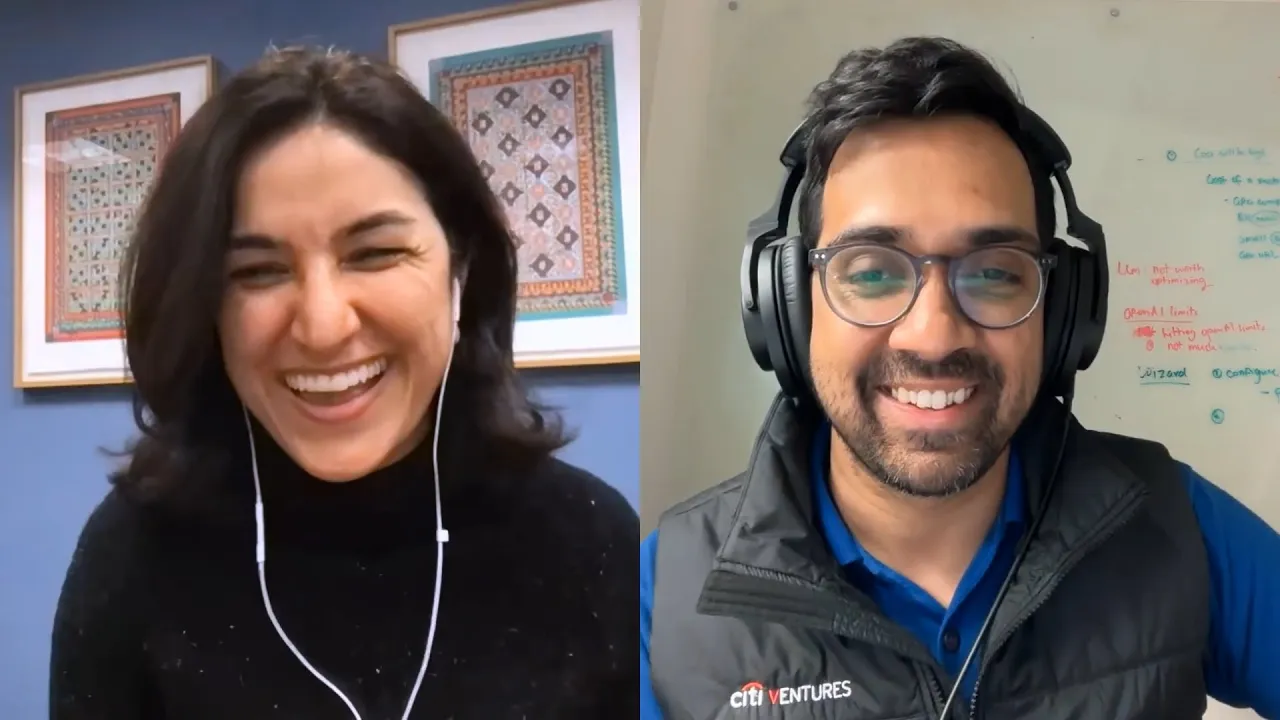
What is AI Governance?
AI governance is a structured framework of principles, best practices, and oversight mechanisms that enables organizations to maximize AI's potential while minimizing associated risks. It provides a comprehensive approach to managing artificial intelligence systems in alignment with organizational goals, regulatory requirements, and societal values.
The shift from traditional machine learning to generative AI has completely changed the AI governance landscape. Traditional ML models needed oversight for data accuracy and output validation, but generative AI demands stricter governance because it creates new content, shows unexpected behaviors, and interacts with users in unpredictable ways.
Implementing effective AI governance requires a systematic approach that addresses both technical requirements and organizational processes. Here is a seven-step framework that provides organizations with a practical roadmap for developing and maintaining robust AI governance in their AI implementations.

Step #1: Understand the Regulatory Landscape
The global AI regulatory picture is changing fast, with major regions taking different approaches. Knowing these differences is essential for creating your AI governance strategy.
The EU leads with the most thorough framework through the EU AI Act, which became law in August 2024. This regulation sorts AI systems by risk level and sets specific requirements for each category. Its influence extends beyond EU borders through the "Brussels Effect" as companies worldwide must comply to do business in European markets.
China takes a control-focused approach, requiring algorithm registration and content oversight. Their rules make companies register algorithms with the Cyberspace Administration of China (CAC) and emphasize information control, especially for technologies that shape public opinion.
Similarly, the United States has chosen a pro-innovation path with decentralized oversight, using mostly sectoral guidelines and voluntary frameworks instead of comprehensive laws. This creates a patchwork of state-level regulations that businesses must navigate.
For global organizations, these different approaches create significant compliance challenges. Your obligations vary dramatically based on where you operate and your industry, with healthcare, finance, and transportation facing the strictest requirements across all regions.
Understanding these differences isn't just about checking boxes—it's about future-proofing your AI governance strategy. Organizations that align their governance with regional requirements can navigate this complex landscape while maintaining room for innovation.
Step #2: Establish Your AI Governance Framework
To build a strong AI governance framework, start by defining clear organizational structures. Appoint AI ethics officers and create governance committees with members from different departments. These structures ensure consistent oversight across all AI projects and establish clear accountability for decisions at every level.
Your governance policies should match your organization's AI maturity. The NIST AI Risk Management Framework helps create approaches that grow with your capabilities. Begin with basic policies for high-risk systems, then expand as your AI matures and diversifies.
Documentation serves as the backbone of effective AI governance. Create standard templates for model cards, data lineage tracking, and risk assessments. Document when human oversight is needed and set approval thresholds based on potential impact. This documentation creates transparency and supports audits.
Make sure your framework addresses your industry's unique challenges. Financial institutions need strong bias monitoring for lending algorithms, while healthcare organizations must prioritize patient privacy and safety. Tailoring your framework to sector-specific requirements helps navigate complex regulations while maintaining innovation.
Galileo's platform offers specialized modules to put your AI governance framework into action, combining model documentation, risk assessment, and compliance monitoring into unified workflows. Galileo simplifies collaboration between technical teams and governance committees, providing complete visibility into AI systems while automating governance processes as your AI initiatives grow.
Step #3: Implement Effective Evaluation Systems
Evaluating generative AI outputs is challenging since there's often no "correct" answer for creative tasks. You need specialized approaches focused on consistency, coherence, and alignment with user intent rather than simple right/wrong metrics.
A/B testing lets you compare model variations by measuring user engagement, satisfaction, or task completion rates. Split traffic between different model versions and analyze performance differences across metrics that matter for your specific use case and business goals.
Nowadays, autonomous evaluation uses AI to evaluate AI, with evaluation models assessing outputs based on criteria like factual consistency or instruction adherence. This approach handles large volumes of outputs, but remember to verify that your evaluation models don't repeat the same biases as your production models.
In addition, benchmarking against standard datasets helps establish baseline performance, even for creative tasks. Create custom benchmarks for your specific use cases by developing test suites with diverse prompts that test different capabilities and potential failures of your AI system.
For creative outputs like marketing copy or design, evaluation becomes especially challenging. Technical solutions include developing multi-dimensional scoring systems that assess outputs for brand voice consistency, persuasiveness, and originality.
Additionally, being vigilant about LLM hallucinations ensures the content generated is both accurate and trustworthy. Evaluating multimodal AI systems presents unique challenges that require specialized multimodal AI evaluation methodologies. Consider using human review for a sample set to calibrate your automated metrics.
Companies like Anthropic demonstrate best practices by using comprehensive evaluation frameworks that combine human feedback, automated metrics, and red-teaming to test for vulnerabilities. Their approach tracks both improvements and regressions across multiple dimensions while protecting user privacy and system security.
Galileo further provides comprehensive evaluation capabilities without needing ground truth data, letting you assess model outputs across multiple dimensions, including factuality, coherence, and instruction following.
Galileo’s integrated framework combines automated metrics with customizable evaluation criteria, helping you maintain quality, catch regressions, and continuously improve your AI systems while meeting compliance requirements.
Step #4: Deploy Robust Monitoring and Observability
Continuous AI monitoring and observability are vital for effective AI governance, helping teams catch issues before they impact your business. Understanding the differences between monitoring and observability can further improve your strategies. Good monitoring systems identify model drift, performance problems, and potential bias that could create compliance or ethical risks.
Start by tracking metrics based on your specific risk profile and use cases. These typically include performance metrics (accuracy, precision, recall), fairness indicators across demographic groups, data drift measurements, and operational metrics like latency and throughput. Your monitoring framework should align with your AI risk assessments.
When implementing monitoring, connect it with your existing MLOps infrastructure to avoid creating new silos. Tools like Grafana can track model health and alert teams when metrics fall outside acceptable ranges. The key is automating this process to ensure vigilance.
Set clear performance thresholds based on your organization's risk tolerance and use case requirements. When creating alert mechanisms, ensure they reach the right people and include action plans, not just notifications.
To avoid building monitoring infrastructure from the ground up, Galileo provides real-time monitoring that simplifies compliance by automatically tracking model drift, bias, and performance issues. Galileo’s monitoring dashboard gives teams immediate visibility into potential governance issues, allowing for quick intervention before problems become regulatory concerns.
Step #5: Implement Comprehensive Protection and Risk Mitigation
Protecting your AI systems requires AI security practices and technical safeguards that address generative models' unique risks. Start by implementing robust input filtering to prevent prompt injection and other attacks. These filters should detect and block potentially harmful inputs before they reach your model, reducing the risk of exploitation.
Output verification is equally important. Deploy systems that check generated content against safety criteria before delivery to users. This verification layer should identify problematic content like misinformation, harmful advice, or biased responses that could damage trust and expose your organization to liability.
Different industries face unique AI risks requiring tailored approaches. In finance, follow established AI risk management frameworks that emphasize ongoing validation and monitoring. Banking regulators increasingly require comprehensive documentation of AI model behavior and performance metrics. Healthcare organizations must prioritize patient personal data protection with strict access controls and anonymization techniques.
The University of Technology Sydney provides an excellent example. They implemented collaborative AI governance by conducting detailed consultations with students and staff to establish policies aligned with their priorities. Their approach demonstrates how stakeholder engagement strengthens protection mechanisms and builds trust.
Galileo helps organizations implement these protection strategies with built-in guardrails metrics, bias detection, and monitoring tools. Galileo's customizable controls let you tailor risk mitigation to your specific industry requirements while maintaining visibility into how your models perform across diverse user populations and inputs.
Step #6: Build an AI Governance Culture and Cross-Functional Collaboration
Building a strong AI governance culture means addressing the human side of AI deployment. Develop AI governance literacy across your organization. Create targeted training programs that connect technical concepts with everyday applications, ensuring both developers and business teams understand their roles in maintaining compliance.
Similarly, cross-functional collaboration forms the backbone of effective AI governance. Form committees including legal, technical, and product teams to improve oversight. These groups should meet regularly to review model performance, discuss emerging risks, and align on governance priorities that balance innovation and compliance.
Executive support often determines whether AI governance efforts succeed. Secure leadership buy-in by showing the business value of governance through metrics like risk reduction, compliance efficiency, and improved customer trust.
Organizations like Telstra demonstrate how dedicated structures like their Risk Council of AI & Data establish clear ownership for oversight and decision-making. This approach creates accountability while preventing governance from becoming isolated within technical teams.
Galileo enhances cross-functional collaboration through intuitive interfaces and role-based access controls that make governance accessible to all stakeholders regardless of technical background. These capabilities ensure everyone can contribute effectively to responsible AI deployment, creating an integrated AI governance ecosystem.
Step #7: Future-Proof Your AI Governance Strategy
Technology changes rapidly, requiring AI governance frameworks that can evolve alongside emerging AI capabilities. Implement flexible policies that address fundamental principles rather than specific technologies so your framework adapts to new AI models without complete restructuring.
As you move from pilot projects to enterprise-wide AI deployment, establish scalable documentation processes and standardized risk assessment protocols. This prevents AI governance from becoming a bottleneck while maintaining necessary oversight. Consider automated monitoring tools that scale with your AI footprint while maintaining consistent oversight.
Multi-model systems and third-party AI components create unique challenges. Robust data lineage tracking becomes essential to maintain visibility across complex AI ecosystems. Successful organizations maintain comprehensive inventories of all AI components, including clear documentation of interdependencies and responsibilities.
To stay ahead of regulatory changes, establish dedicated teams responsible for monitoring evolving frameworks like the EU AI Act. The "Brussels Effect" means European regulations often influence global standards, making it prudent to align with the strictest requirements proactively rather than reactively.
Emerging trends point toward more collaborative AI governance models, including industry-specific coalitions and decentralized oversight. Consider joining these initiatives to share best practices and influence emerging standards. Galileo aligns with this forward-looking approach, providing the flexibility to adapt as AI technologies and regulatory requirements continue to evolve.
Transform Your AI Governance With Galileo
Good AI governance isn't just about checking regulatory boxes—it's a strategic advantage that drives innovation while managing risks. However, implementing strong AI governance requires the right tools. Galileo offers a complete solution that supports comprehensive AI governance implementation:
Explore how Galileo can help you implement a governance framework that balances innovation with responsibility, regardless of the complexity of your implementation.
A single AI system gone wrong can devastate many processes overnight. Without proper AI governance, companies face serious threats, from biased algorithms alienating customers to models leaking sensitive data, triggering penalties, and destroying trust.
Stakes are rising as enforcement tightens under frameworks like the EU AI Act, with fines reaching €35M or 7% of annual revenue. Organizations now face a clear challenge: unlock AI's benefits while ensuring responsible use. Without proper AI governance, AI systems can cause regulatory penalties, reputation damage, biased outcomes, and wasted resources.
This article explores seven key steps for organizations to implement effective, well-governed AI that delivers better results, accelerates innovation, and builds stakeholder confidence.
We recently explored this topic on our Chain of Thought podcast, where industry experts shared practical insights and real-world implementation strategies.
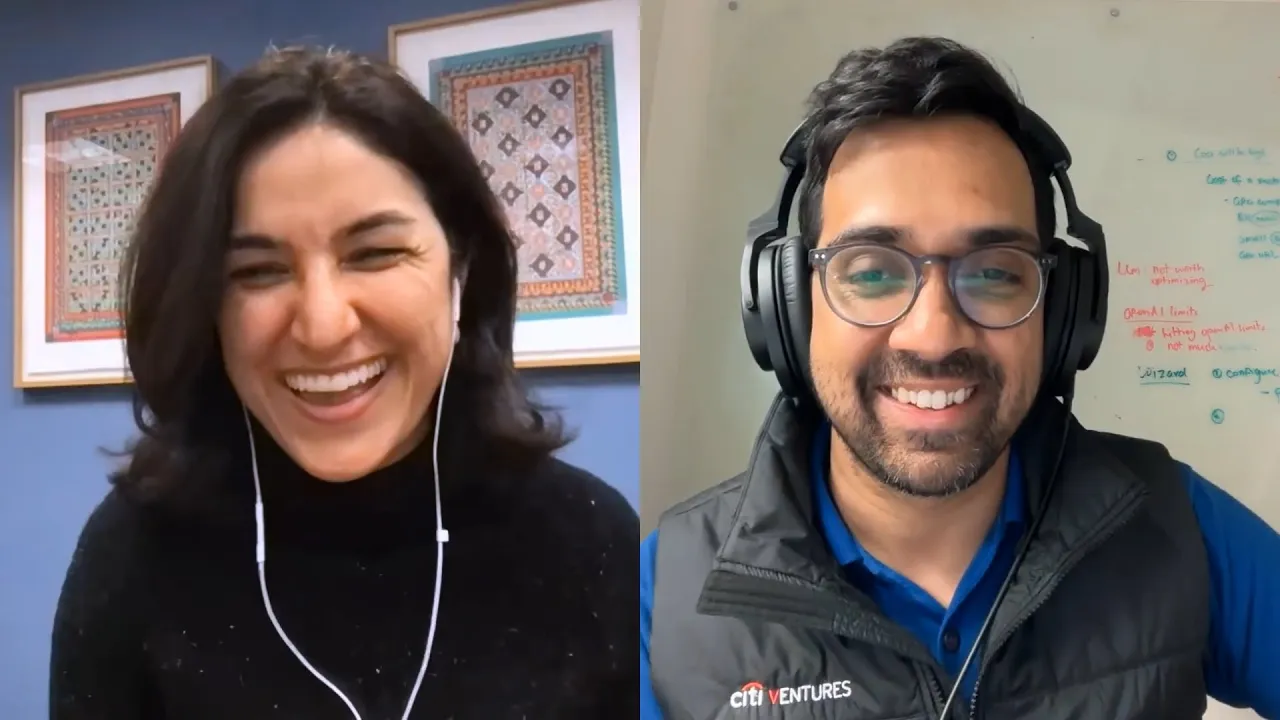
What is AI Governance?
AI governance is a structured framework of principles, best practices, and oversight mechanisms that enables organizations to maximize AI's potential while minimizing associated risks. It provides a comprehensive approach to managing artificial intelligence systems in alignment with organizational goals, regulatory requirements, and societal values.
The shift from traditional machine learning to generative AI has completely changed the AI governance landscape. Traditional ML models needed oversight for data accuracy and output validation, but generative AI demands stricter governance because it creates new content, shows unexpected behaviors, and interacts with users in unpredictable ways.
Implementing effective AI governance requires a systematic approach that addresses both technical requirements and organizational processes. Here is a seven-step framework that provides organizations with a practical roadmap for developing and maintaining robust AI governance in their AI implementations.

Step #1: Understand the Regulatory Landscape
The global AI regulatory picture is changing fast, with major regions taking different approaches. Knowing these differences is essential for creating your AI governance strategy.
The EU leads with the most thorough framework through the EU AI Act, which became law in August 2024. This regulation sorts AI systems by risk level and sets specific requirements for each category. Its influence extends beyond EU borders through the "Brussels Effect" as companies worldwide must comply to do business in European markets.
China takes a control-focused approach, requiring algorithm registration and content oversight. Their rules make companies register algorithms with the Cyberspace Administration of China (CAC) and emphasize information control, especially for technologies that shape public opinion.
Similarly, the United States has chosen a pro-innovation path with decentralized oversight, using mostly sectoral guidelines and voluntary frameworks instead of comprehensive laws. This creates a patchwork of state-level regulations that businesses must navigate.
For global organizations, these different approaches create significant compliance challenges. Your obligations vary dramatically based on where you operate and your industry, with healthcare, finance, and transportation facing the strictest requirements across all regions.
Understanding these differences isn't just about checking boxes—it's about future-proofing your AI governance strategy. Organizations that align their governance with regional requirements can navigate this complex landscape while maintaining room for innovation.
Step #2: Establish Your AI Governance Framework
To build a strong AI governance framework, start by defining clear organizational structures. Appoint AI ethics officers and create governance committees with members from different departments. These structures ensure consistent oversight across all AI projects and establish clear accountability for decisions at every level.
Your governance policies should match your organization's AI maturity. The NIST AI Risk Management Framework helps create approaches that grow with your capabilities. Begin with basic policies for high-risk systems, then expand as your AI matures and diversifies.
Documentation serves as the backbone of effective AI governance. Create standard templates for model cards, data lineage tracking, and risk assessments. Document when human oversight is needed and set approval thresholds based on potential impact. This documentation creates transparency and supports audits.
Make sure your framework addresses your industry's unique challenges. Financial institutions need strong bias monitoring for lending algorithms, while healthcare organizations must prioritize patient privacy and safety. Tailoring your framework to sector-specific requirements helps navigate complex regulations while maintaining innovation.
Galileo's platform offers specialized modules to put your AI governance framework into action, combining model documentation, risk assessment, and compliance monitoring into unified workflows. Galileo simplifies collaboration between technical teams and governance committees, providing complete visibility into AI systems while automating governance processes as your AI initiatives grow.
Step #3: Implement Effective Evaluation Systems
Evaluating generative AI outputs is challenging since there's often no "correct" answer for creative tasks. You need specialized approaches focused on consistency, coherence, and alignment with user intent rather than simple right/wrong metrics.
A/B testing lets you compare model variations by measuring user engagement, satisfaction, or task completion rates. Split traffic between different model versions and analyze performance differences across metrics that matter for your specific use case and business goals.
Nowadays, autonomous evaluation uses AI to evaluate AI, with evaluation models assessing outputs based on criteria like factual consistency or instruction adherence. This approach handles large volumes of outputs, but remember to verify that your evaluation models don't repeat the same biases as your production models.
In addition, benchmarking against standard datasets helps establish baseline performance, even for creative tasks. Create custom benchmarks for your specific use cases by developing test suites with diverse prompts that test different capabilities and potential failures of your AI system.
For creative outputs like marketing copy or design, evaluation becomes especially challenging. Technical solutions include developing multi-dimensional scoring systems that assess outputs for brand voice consistency, persuasiveness, and originality.
Additionally, being vigilant about LLM hallucinations ensures the content generated is both accurate and trustworthy. Evaluating multimodal AI systems presents unique challenges that require specialized multimodal AI evaluation methodologies. Consider using human review for a sample set to calibrate your automated metrics.
Companies like Anthropic demonstrate best practices by using comprehensive evaluation frameworks that combine human feedback, automated metrics, and red-teaming to test for vulnerabilities. Their approach tracks both improvements and regressions across multiple dimensions while protecting user privacy and system security.
Galileo further provides comprehensive evaluation capabilities without needing ground truth data, letting you assess model outputs across multiple dimensions, including factuality, coherence, and instruction following.
Galileo’s integrated framework combines automated metrics with customizable evaluation criteria, helping you maintain quality, catch regressions, and continuously improve your AI systems while meeting compliance requirements.
Step #4: Deploy Robust Monitoring and Observability
Continuous AI monitoring and observability are vital for effective AI governance, helping teams catch issues before they impact your business. Understanding the differences between monitoring and observability can further improve your strategies. Good monitoring systems identify model drift, performance problems, and potential bias that could create compliance or ethical risks.
Start by tracking metrics based on your specific risk profile and use cases. These typically include performance metrics (accuracy, precision, recall), fairness indicators across demographic groups, data drift measurements, and operational metrics like latency and throughput. Your monitoring framework should align with your AI risk assessments.
When implementing monitoring, connect it with your existing MLOps infrastructure to avoid creating new silos. Tools like Grafana can track model health and alert teams when metrics fall outside acceptable ranges. The key is automating this process to ensure vigilance.
Set clear performance thresholds based on your organization's risk tolerance and use case requirements. When creating alert mechanisms, ensure they reach the right people and include action plans, not just notifications.
To avoid building monitoring infrastructure from the ground up, Galileo provides real-time monitoring that simplifies compliance by automatically tracking model drift, bias, and performance issues. Galileo’s monitoring dashboard gives teams immediate visibility into potential governance issues, allowing for quick intervention before problems become regulatory concerns.
Step #5: Implement Comprehensive Protection and Risk Mitigation
Protecting your AI systems requires AI security practices and technical safeguards that address generative models' unique risks. Start by implementing robust input filtering to prevent prompt injection and other attacks. These filters should detect and block potentially harmful inputs before they reach your model, reducing the risk of exploitation.
Output verification is equally important. Deploy systems that check generated content against safety criteria before delivery to users. This verification layer should identify problematic content like misinformation, harmful advice, or biased responses that could damage trust and expose your organization to liability.
Different industries face unique AI risks requiring tailored approaches. In finance, follow established AI risk management frameworks that emphasize ongoing validation and monitoring. Banking regulators increasingly require comprehensive documentation of AI model behavior and performance metrics. Healthcare organizations must prioritize patient personal data protection with strict access controls and anonymization techniques.
The University of Technology Sydney provides an excellent example. They implemented collaborative AI governance by conducting detailed consultations with students and staff to establish policies aligned with their priorities. Their approach demonstrates how stakeholder engagement strengthens protection mechanisms and builds trust.
Galileo helps organizations implement these protection strategies with built-in guardrails metrics, bias detection, and monitoring tools. Galileo's customizable controls let you tailor risk mitigation to your specific industry requirements while maintaining visibility into how your models perform across diverse user populations and inputs.
Step #6: Build an AI Governance Culture and Cross-Functional Collaboration
Building a strong AI governance culture means addressing the human side of AI deployment. Develop AI governance literacy across your organization. Create targeted training programs that connect technical concepts with everyday applications, ensuring both developers and business teams understand their roles in maintaining compliance.
Similarly, cross-functional collaboration forms the backbone of effective AI governance. Form committees including legal, technical, and product teams to improve oversight. These groups should meet regularly to review model performance, discuss emerging risks, and align on governance priorities that balance innovation and compliance.
Executive support often determines whether AI governance efforts succeed. Secure leadership buy-in by showing the business value of governance through metrics like risk reduction, compliance efficiency, and improved customer trust.
Organizations like Telstra demonstrate how dedicated structures like their Risk Council of AI & Data establish clear ownership for oversight and decision-making. This approach creates accountability while preventing governance from becoming isolated within technical teams.
Galileo enhances cross-functional collaboration through intuitive interfaces and role-based access controls that make governance accessible to all stakeholders regardless of technical background. These capabilities ensure everyone can contribute effectively to responsible AI deployment, creating an integrated AI governance ecosystem.
Step #7: Future-Proof Your AI Governance Strategy
Technology changes rapidly, requiring AI governance frameworks that can evolve alongside emerging AI capabilities. Implement flexible policies that address fundamental principles rather than specific technologies so your framework adapts to new AI models without complete restructuring.
As you move from pilot projects to enterprise-wide AI deployment, establish scalable documentation processes and standardized risk assessment protocols. This prevents AI governance from becoming a bottleneck while maintaining necessary oversight. Consider automated monitoring tools that scale with your AI footprint while maintaining consistent oversight.
Multi-model systems and third-party AI components create unique challenges. Robust data lineage tracking becomes essential to maintain visibility across complex AI ecosystems. Successful organizations maintain comprehensive inventories of all AI components, including clear documentation of interdependencies and responsibilities.
To stay ahead of regulatory changes, establish dedicated teams responsible for monitoring evolving frameworks like the EU AI Act. The "Brussels Effect" means European regulations often influence global standards, making it prudent to align with the strictest requirements proactively rather than reactively.
Emerging trends point toward more collaborative AI governance models, including industry-specific coalitions and decentralized oversight. Consider joining these initiatives to share best practices and influence emerging standards. Galileo aligns with this forward-looking approach, providing the flexibility to adapt as AI technologies and regulatory requirements continue to evolve.
Transform Your AI Governance With Galileo
Good AI governance isn't just about checking regulatory boxes—it's a strategic advantage that drives innovation while managing risks. However, implementing strong AI governance requires the right tools. Galileo offers a complete solution that supports comprehensive AI governance implementation:
Explore how Galileo can help you implement a governance framework that balances innovation with responsibility, regardless of the complexity of your implementation.
A single AI system gone wrong can devastate many processes overnight. Without proper AI governance, companies face serious threats, from biased algorithms alienating customers to models leaking sensitive data, triggering penalties, and destroying trust.
Stakes are rising as enforcement tightens under frameworks like the EU AI Act, with fines reaching €35M or 7% of annual revenue. Organizations now face a clear challenge: unlock AI's benefits while ensuring responsible use. Without proper AI governance, AI systems can cause regulatory penalties, reputation damage, biased outcomes, and wasted resources.
This article explores seven key steps for organizations to implement effective, well-governed AI that delivers better results, accelerates innovation, and builds stakeholder confidence.
We recently explored this topic on our Chain of Thought podcast, where industry experts shared practical insights and real-world implementation strategies.
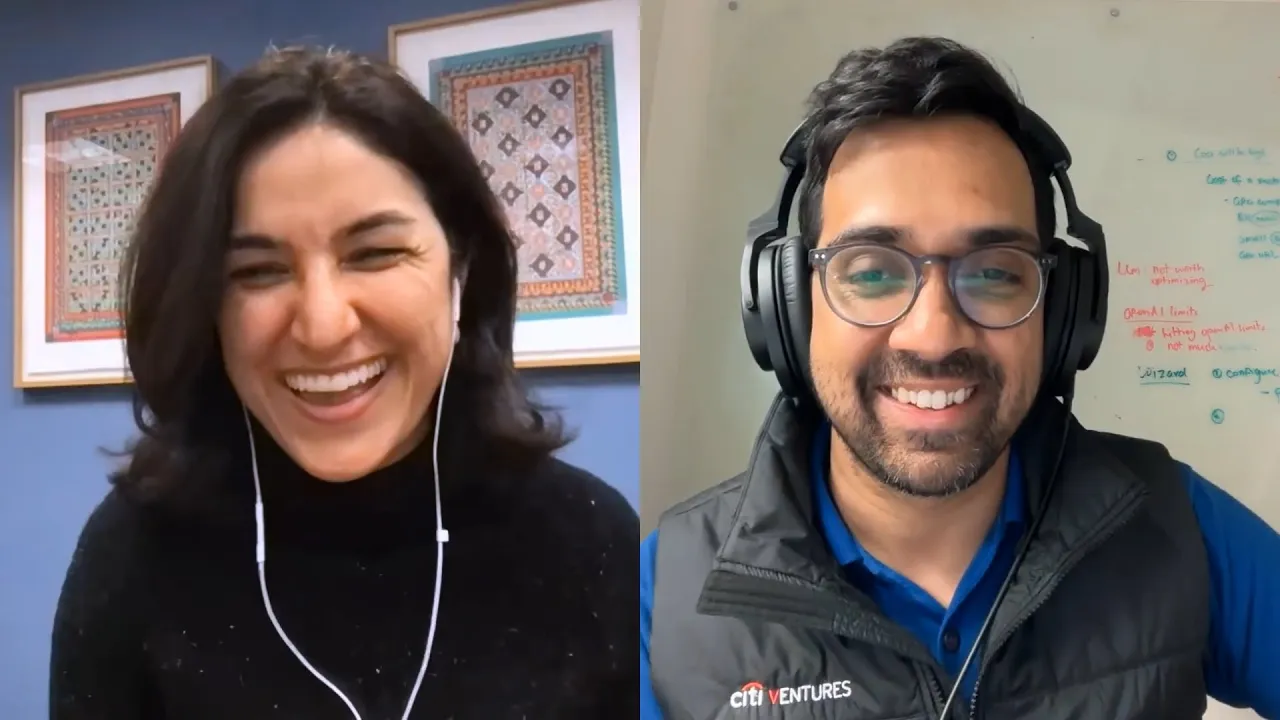
What is AI Governance?
AI governance is a structured framework of principles, best practices, and oversight mechanisms that enables organizations to maximize AI's potential while minimizing associated risks. It provides a comprehensive approach to managing artificial intelligence systems in alignment with organizational goals, regulatory requirements, and societal values.
The shift from traditional machine learning to generative AI has completely changed the AI governance landscape. Traditional ML models needed oversight for data accuracy and output validation, but generative AI demands stricter governance because it creates new content, shows unexpected behaviors, and interacts with users in unpredictable ways.
Implementing effective AI governance requires a systematic approach that addresses both technical requirements and organizational processes. Here is a seven-step framework that provides organizations with a practical roadmap for developing and maintaining robust AI governance in their AI implementations.

Step #1: Understand the Regulatory Landscape
The global AI regulatory picture is changing fast, with major regions taking different approaches. Knowing these differences is essential for creating your AI governance strategy.
The EU leads with the most thorough framework through the EU AI Act, which became law in August 2024. This regulation sorts AI systems by risk level and sets specific requirements for each category. Its influence extends beyond EU borders through the "Brussels Effect" as companies worldwide must comply to do business in European markets.
China takes a control-focused approach, requiring algorithm registration and content oversight. Their rules make companies register algorithms with the Cyberspace Administration of China (CAC) and emphasize information control, especially for technologies that shape public opinion.
Similarly, the United States has chosen a pro-innovation path with decentralized oversight, using mostly sectoral guidelines and voluntary frameworks instead of comprehensive laws. This creates a patchwork of state-level regulations that businesses must navigate.
For global organizations, these different approaches create significant compliance challenges. Your obligations vary dramatically based on where you operate and your industry, with healthcare, finance, and transportation facing the strictest requirements across all regions.
Understanding these differences isn't just about checking boxes—it's about future-proofing your AI governance strategy. Organizations that align their governance with regional requirements can navigate this complex landscape while maintaining room for innovation.
Step #2: Establish Your AI Governance Framework
To build a strong AI governance framework, start by defining clear organizational structures. Appoint AI ethics officers and create governance committees with members from different departments. These structures ensure consistent oversight across all AI projects and establish clear accountability for decisions at every level.
Your governance policies should match your organization's AI maturity. The NIST AI Risk Management Framework helps create approaches that grow with your capabilities. Begin with basic policies for high-risk systems, then expand as your AI matures and diversifies.
Documentation serves as the backbone of effective AI governance. Create standard templates for model cards, data lineage tracking, and risk assessments. Document when human oversight is needed and set approval thresholds based on potential impact. This documentation creates transparency and supports audits.
Make sure your framework addresses your industry's unique challenges. Financial institutions need strong bias monitoring for lending algorithms, while healthcare organizations must prioritize patient privacy and safety. Tailoring your framework to sector-specific requirements helps navigate complex regulations while maintaining innovation.
Galileo's platform offers specialized modules to put your AI governance framework into action, combining model documentation, risk assessment, and compliance monitoring into unified workflows. Galileo simplifies collaboration between technical teams and governance committees, providing complete visibility into AI systems while automating governance processes as your AI initiatives grow.
Step #3: Implement Effective Evaluation Systems
Evaluating generative AI outputs is challenging since there's often no "correct" answer for creative tasks. You need specialized approaches focused on consistency, coherence, and alignment with user intent rather than simple right/wrong metrics.
A/B testing lets you compare model variations by measuring user engagement, satisfaction, or task completion rates. Split traffic between different model versions and analyze performance differences across metrics that matter for your specific use case and business goals.
Nowadays, autonomous evaluation uses AI to evaluate AI, with evaluation models assessing outputs based on criteria like factual consistency or instruction adherence. This approach handles large volumes of outputs, but remember to verify that your evaluation models don't repeat the same biases as your production models.
In addition, benchmarking against standard datasets helps establish baseline performance, even for creative tasks. Create custom benchmarks for your specific use cases by developing test suites with diverse prompts that test different capabilities and potential failures of your AI system.
For creative outputs like marketing copy or design, evaluation becomes especially challenging. Technical solutions include developing multi-dimensional scoring systems that assess outputs for brand voice consistency, persuasiveness, and originality.
Additionally, being vigilant about LLM hallucinations ensures the content generated is both accurate and trustworthy. Evaluating multimodal AI systems presents unique challenges that require specialized multimodal AI evaluation methodologies. Consider using human review for a sample set to calibrate your automated metrics.
Companies like Anthropic demonstrate best practices by using comprehensive evaluation frameworks that combine human feedback, automated metrics, and red-teaming to test for vulnerabilities. Their approach tracks both improvements and regressions across multiple dimensions while protecting user privacy and system security.
Galileo further provides comprehensive evaluation capabilities without needing ground truth data, letting you assess model outputs across multiple dimensions, including factuality, coherence, and instruction following.
Galileo’s integrated framework combines automated metrics with customizable evaluation criteria, helping you maintain quality, catch regressions, and continuously improve your AI systems while meeting compliance requirements.
Step #4: Deploy Robust Monitoring and Observability
Continuous AI monitoring and observability are vital for effective AI governance, helping teams catch issues before they impact your business. Understanding the differences between monitoring and observability can further improve your strategies. Good monitoring systems identify model drift, performance problems, and potential bias that could create compliance or ethical risks.
Start by tracking metrics based on your specific risk profile and use cases. These typically include performance metrics (accuracy, precision, recall), fairness indicators across demographic groups, data drift measurements, and operational metrics like latency and throughput. Your monitoring framework should align with your AI risk assessments.
When implementing monitoring, connect it with your existing MLOps infrastructure to avoid creating new silos. Tools like Grafana can track model health and alert teams when metrics fall outside acceptable ranges. The key is automating this process to ensure vigilance.
Set clear performance thresholds based on your organization's risk tolerance and use case requirements. When creating alert mechanisms, ensure they reach the right people and include action plans, not just notifications.
To avoid building monitoring infrastructure from the ground up, Galileo provides real-time monitoring that simplifies compliance by automatically tracking model drift, bias, and performance issues. Galileo’s monitoring dashboard gives teams immediate visibility into potential governance issues, allowing for quick intervention before problems become regulatory concerns.
Step #5: Implement Comprehensive Protection and Risk Mitigation
Protecting your AI systems requires AI security practices and technical safeguards that address generative models' unique risks. Start by implementing robust input filtering to prevent prompt injection and other attacks. These filters should detect and block potentially harmful inputs before they reach your model, reducing the risk of exploitation.
Output verification is equally important. Deploy systems that check generated content against safety criteria before delivery to users. This verification layer should identify problematic content like misinformation, harmful advice, or biased responses that could damage trust and expose your organization to liability.
Different industries face unique AI risks requiring tailored approaches. In finance, follow established AI risk management frameworks that emphasize ongoing validation and monitoring. Banking regulators increasingly require comprehensive documentation of AI model behavior and performance metrics. Healthcare organizations must prioritize patient personal data protection with strict access controls and anonymization techniques.
The University of Technology Sydney provides an excellent example. They implemented collaborative AI governance by conducting detailed consultations with students and staff to establish policies aligned with their priorities. Their approach demonstrates how stakeholder engagement strengthens protection mechanisms and builds trust.
Galileo helps organizations implement these protection strategies with built-in guardrails metrics, bias detection, and monitoring tools. Galileo's customizable controls let you tailor risk mitigation to your specific industry requirements while maintaining visibility into how your models perform across diverse user populations and inputs.
Step #6: Build an AI Governance Culture and Cross-Functional Collaboration
Building a strong AI governance culture means addressing the human side of AI deployment. Develop AI governance literacy across your organization. Create targeted training programs that connect technical concepts with everyday applications, ensuring both developers and business teams understand their roles in maintaining compliance.
Similarly, cross-functional collaboration forms the backbone of effective AI governance. Form committees including legal, technical, and product teams to improve oversight. These groups should meet regularly to review model performance, discuss emerging risks, and align on governance priorities that balance innovation and compliance.
Executive support often determines whether AI governance efforts succeed. Secure leadership buy-in by showing the business value of governance through metrics like risk reduction, compliance efficiency, and improved customer trust.
Organizations like Telstra demonstrate how dedicated structures like their Risk Council of AI & Data establish clear ownership for oversight and decision-making. This approach creates accountability while preventing governance from becoming isolated within technical teams.
Galileo enhances cross-functional collaboration through intuitive interfaces and role-based access controls that make governance accessible to all stakeholders regardless of technical background. These capabilities ensure everyone can contribute effectively to responsible AI deployment, creating an integrated AI governance ecosystem.
Step #7: Future-Proof Your AI Governance Strategy
Technology changes rapidly, requiring AI governance frameworks that can evolve alongside emerging AI capabilities. Implement flexible policies that address fundamental principles rather than specific technologies so your framework adapts to new AI models without complete restructuring.
As you move from pilot projects to enterprise-wide AI deployment, establish scalable documentation processes and standardized risk assessment protocols. This prevents AI governance from becoming a bottleneck while maintaining necessary oversight. Consider automated monitoring tools that scale with your AI footprint while maintaining consistent oversight.
Multi-model systems and third-party AI components create unique challenges. Robust data lineage tracking becomes essential to maintain visibility across complex AI ecosystems. Successful organizations maintain comprehensive inventories of all AI components, including clear documentation of interdependencies and responsibilities.
To stay ahead of regulatory changes, establish dedicated teams responsible for monitoring evolving frameworks like the EU AI Act. The "Brussels Effect" means European regulations often influence global standards, making it prudent to align with the strictest requirements proactively rather than reactively.
Emerging trends point toward more collaborative AI governance models, including industry-specific coalitions and decentralized oversight. Consider joining these initiatives to share best practices and influence emerging standards. Galileo aligns with this forward-looking approach, providing the flexibility to adapt as AI technologies and regulatory requirements continue to evolve.
Transform Your AI Governance With Galileo
Good AI governance isn't just about checking regulatory boxes—it's a strategic advantage that drives innovation while managing risks. However, implementing strong AI governance requires the right tools. Galileo offers a complete solution that supports comprehensive AI governance implementation:
Explore how Galileo can help you implement a governance framework that balances innovation with responsibility, regardless of the complexity of your implementation.
A single AI system gone wrong can devastate many processes overnight. Without proper AI governance, companies face serious threats, from biased algorithms alienating customers to models leaking sensitive data, triggering penalties, and destroying trust.
Stakes are rising as enforcement tightens under frameworks like the EU AI Act, with fines reaching €35M or 7% of annual revenue. Organizations now face a clear challenge: unlock AI's benefits while ensuring responsible use. Without proper AI governance, AI systems can cause regulatory penalties, reputation damage, biased outcomes, and wasted resources.
This article explores seven key steps for organizations to implement effective, well-governed AI that delivers better results, accelerates innovation, and builds stakeholder confidence.
We recently explored this topic on our Chain of Thought podcast, where industry experts shared practical insights and real-world implementation strategies.
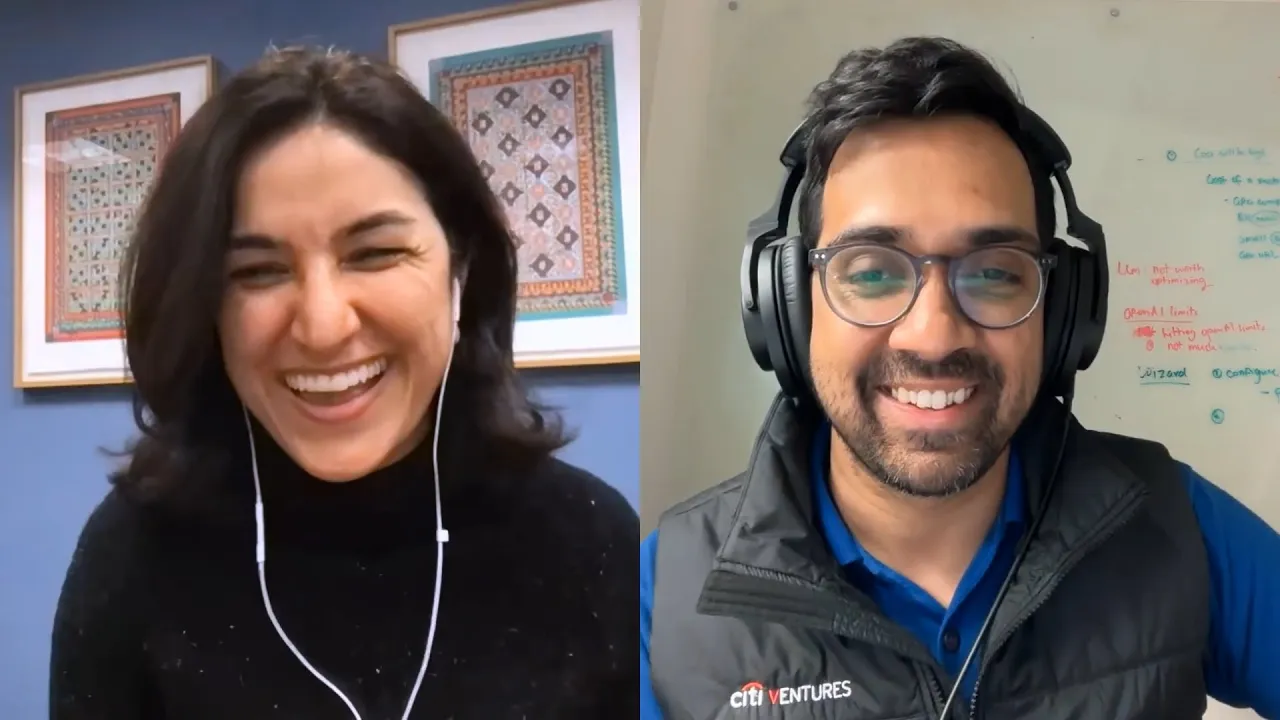
What is AI Governance?
AI governance is a structured framework of principles, best practices, and oversight mechanisms that enables organizations to maximize AI's potential while minimizing associated risks. It provides a comprehensive approach to managing artificial intelligence systems in alignment with organizational goals, regulatory requirements, and societal values.
The shift from traditional machine learning to generative AI has completely changed the AI governance landscape. Traditional ML models needed oversight for data accuracy and output validation, but generative AI demands stricter governance because it creates new content, shows unexpected behaviors, and interacts with users in unpredictable ways.
Implementing effective AI governance requires a systematic approach that addresses both technical requirements and organizational processes. Here is a seven-step framework that provides organizations with a practical roadmap for developing and maintaining robust AI governance in their AI implementations.

Step #1: Understand the Regulatory Landscape
The global AI regulatory picture is changing fast, with major regions taking different approaches. Knowing these differences is essential for creating your AI governance strategy.
The EU leads with the most thorough framework through the EU AI Act, which became law in August 2024. This regulation sorts AI systems by risk level and sets specific requirements for each category. Its influence extends beyond EU borders through the "Brussels Effect" as companies worldwide must comply to do business in European markets.
China takes a control-focused approach, requiring algorithm registration and content oversight. Their rules make companies register algorithms with the Cyberspace Administration of China (CAC) and emphasize information control, especially for technologies that shape public opinion.
Similarly, the United States has chosen a pro-innovation path with decentralized oversight, using mostly sectoral guidelines and voluntary frameworks instead of comprehensive laws. This creates a patchwork of state-level regulations that businesses must navigate.
For global organizations, these different approaches create significant compliance challenges. Your obligations vary dramatically based on where you operate and your industry, with healthcare, finance, and transportation facing the strictest requirements across all regions.
Understanding these differences isn't just about checking boxes—it's about future-proofing your AI governance strategy. Organizations that align their governance with regional requirements can navigate this complex landscape while maintaining room for innovation.
Step #2: Establish Your AI Governance Framework
To build a strong AI governance framework, start by defining clear organizational structures. Appoint AI ethics officers and create governance committees with members from different departments. These structures ensure consistent oversight across all AI projects and establish clear accountability for decisions at every level.
Your governance policies should match your organization's AI maturity. The NIST AI Risk Management Framework helps create approaches that grow with your capabilities. Begin with basic policies for high-risk systems, then expand as your AI matures and diversifies.
Documentation serves as the backbone of effective AI governance. Create standard templates for model cards, data lineage tracking, and risk assessments. Document when human oversight is needed and set approval thresholds based on potential impact. This documentation creates transparency and supports audits.
Make sure your framework addresses your industry's unique challenges. Financial institutions need strong bias monitoring for lending algorithms, while healthcare organizations must prioritize patient privacy and safety. Tailoring your framework to sector-specific requirements helps navigate complex regulations while maintaining innovation.
Galileo's platform offers specialized modules to put your AI governance framework into action, combining model documentation, risk assessment, and compliance monitoring into unified workflows. Galileo simplifies collaboration between technical teams and governance committees, providing complete visibility into AI systems while automating governance processes as your AI initiatives grow.
Step #3: Implement Effective Evaluation Systems
Evaluating generative AI outputs is challenging since there's often no "correct" answer for creative tasks. You need specialized approaches focused on consistency, coherence, and alignment with user intent rather than simple right/wrong metrics.
A/B testing lets you compare model variations by measuring user engagement, satisfaction, or task completion rates. Split traffic between different model versions and analyze performance differences across metrics that matter for your specific use case and business goals.
Nowadays, autonomous evaluation uses AI to evaluate AI, with evaluation models assessing outputs based on criteria like factual consistency or instruction adherence. This approach handles large volumes of outputs, but remember to verify that your evaluation models don't repeat the same biases as your production models.
In addition, benchmarking against standard datasets helps establish baseline performance, even for creative tasks. Create custom benchmarks for your specific use cases by developing test suites with diverse prompts that test different capabilities and potential failures of your AI system.
For creative outputs like marketing copy or design, evaluation becomes especially challenging. Technical solutions include developing multi-dimensional scoring systems that assess outputs for brand voice consistency, persuasiveness, and originality.
Additionally, being vigilant about LLM hallucinations ensures the content generated is both accurate and trustworthy. Evaluating multimodal AI systems presents unique challenges that require specialized multimodal AI evaluation methodologies. Consider using human review for a sample set to calibrate your automated metrics.
Companies like Anthropic demonstrate best practices by using comprehensive evaluation frameworks that combine human feedback, automated metrics, and red-teaming to test for vulnerabilities. Their approach tracks both improvements and regressions across multiple dimensions while protecting user privacy and system security.
Galileo further provides comprehensive evaluation capabilities without needing ground truth data, letting you assess model outputs across multiple dimensions, including factuality, coherence, and instruction following.
Galileo’s integrated framework combines automated metrics with customizable evaluation criteria, helping you maintain quality, catch regressions, and continuously improve your AI systems while meeting compliance requirements.
Step #4: Deploy Robust Monitoring and Observability
Continuous AI monitoring and observability are vital for effective AI governance, helping teams catch issues before they impact your business. Understanding the differences between monitoring and observability can further improve your strategies. Good monitoring systems identify model drift, performance problems, and potential bias that could create compliance or ethical risks.
Start by tracking metrics based on your specific risk profile and use cases. These typically include performance metrics (accuracy, precision, recall), fairness indicators across demographic groups, data drift measurements, and operational metrics like latency and throughput. Your monitoring framework should align with your AI risk assessments.
When implementing monitoring, connect it with your existing MLOps infrastructure to avoid creating new silos. Tools like Grafana can track model health and alert teams when metrics fall outside acceptable ranges. The key is automating this process to ensure vigilance.
Set clear performance thresholds based on your organization's risk tolerance and use case requirements. When creating alert mechanisms, ensure they reach the right people and include action plans, not just notifications.
To avoid building monitoring infrastructure from the ground up, Galileo provides real-time monitoring that simplifies compliance by automatically tracking model drift, bias, and performance issues. Galileo’s monitoring dashboard gives teams immediate visibility into potential governance issues, allowing for quick intervention before problems become regulatory concerns.
Step #5: Implement Comprehensive Protection and Risk Mitigation
Protecting your AI systems requires AI security practices and technical safeguards that address generative models' unique risks. Start by implementing robust input filtering to prevent prompt injection and other attacks. These filters should detect and block potentially harmful inputs before they reach your model, reducing the risk of exploitation.
Output verification is equally important. Deploy systems that check generated content against safety criteria before delivery to users. This verification layer should identify problematic content like misinformation, harmful advice, or biased responses that could damage trust and expose your organization to liability.
Different industries face unique AI risks requiring tailored approaches. In finance, follow established AI risk management frameworks that emphasize ongoing validation and monitoring. Banking regulators increasingly require comprehensive documentation of AI model behavior and performance metrics. Healthcare organizations must prioritize patient personal data protection with strict access controls and anonymization techniques.
The University of Technology Sydney provides an excellent example. They implemented collaborative AI governance by conducting detailed consultations with students and staff to establish policies aligned with their priorities. Their approach demonstrates how stakeholder engagement strengthens protection mechanisms and builds trust.
Galileo helps organizations implement these protection strategies with built-in guardrails metrics, bias detection, and monitoring tools. Galileo's customizable controls let you tailor risk mitigation to your specific industry requirements while maintaining visibility into how your models perform across diverse user populations and inputs.
Step #6: Build an AI Governance Culture and Cross-Functional Collaboration
Building a strong AI governance culture means addressing the human side of AI deployment. Develop AI governance literacy across your organization. Create targeted training programs that connect technical concepts with everyday applications, ensuring both developers and business teams understand their roles in maintaining compliance.
Similarly, cross-functional collaboration forms the backbone of effective AI governance. Form committees including legal, technical, and product teams to improve oversight. These groups should meet regularly to review model performance, discuss emerging risks, and align on governance priorities that balance innovation and compliance.
Executive support often determines whether AI governance efforts succeed. Secure leadership buy-in by showing the business value of governance through metrics like risk reduction, compliance efficiency, and improved customer trust.
Organizations like Telstra demonstrate how dedicated structures like their Risk Council of AI & Data establish clear ownership for oversight and decision-making. This approach creates accountability while preventing governance from becoming isolated within technical teams.
Galileo enhances cross-functional collaboration through intuitive interfaces and role-based access controls that make governance accessible to all stakeholders regardless of technical background. These capabilities ensure everyone can contribute effectively to responsible AI deployment, creating an integrated AI governance ecosystem.
Step #7: Future-Proof Your AI Governance Strategy
Technology changes rapidly, requiring AI governance frameworks that can evolve alongside emerging AI capabilities. Implement flexible policies that address fundamental principles rather than specific technologies so your framework adapts to new AI models without complete restructuring.
As you move from pilot projects to enterprise-wide AI deployment, establish scalable documentation processes and standardized risk assessment protocols. This prevents AI governance from becoming a bottleneck while maintaining necessary oversight. Consider automated monitoring tools that scale with your AI footprint while maintaining consistent oversight.
Multi-model systems and third-party AI components create unique challenges. Robust data lineage tracking becomes essential to maintain visibility across complex AI ecosystems. Successful organizations maintain comprehensive inventories of all AI components, including clear documentation of interdependencies and responsibilities.
To stay ahead of regulatory changes, establish dedicated teams responsible for monitoring evolving frameworks like the EU AI Act. The "Brussels Effect" means European regulations often influence global standards, making it prudent to align with the strictest requirements proactively rather than reactively.
Emerging trends point toward more collaborative AI governance models, including industry-specific coalitions and decentralized oversight. Consider joining these initiatives to share best practices and influence emerging standards. Galileo aligns with this forward-looking approach, providing the flexibility to adapt as AI technologies and regulatory requirements continue to evolve.
Transform Your AI Governance With Galileo
Good AI governance isn't just about checking regulatory boxes—it's a strategic advantage that drives innovation while managing risks. However, implementing strong AI governance requires the right tools. Galileo offers a complete solution that supports comprehensive AI governance implementation:
Explore how Galileo can help you implement a governance framework that balances innovation with responsibility, regardless of the complexity of your implementation.
Conor Bronsdon
Conor Bronsdon
Conor Bronsdon
Conor Bronsdon