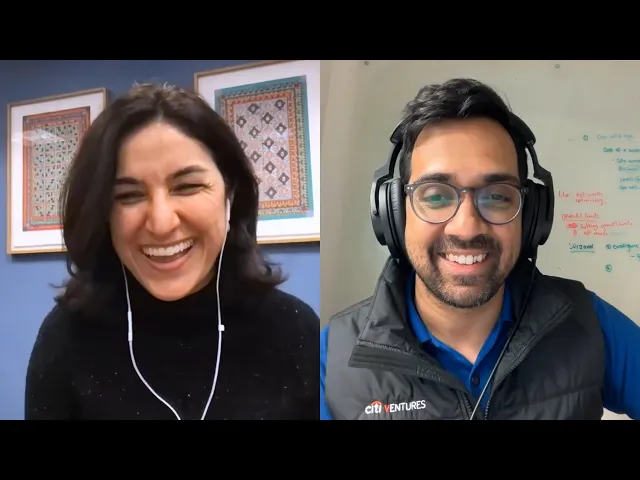
Apr 1, 2025
Building Trust and Transparency in Enterprise AI


Conor Bronsdon
Head of Developer Awareness
Conor Bronsdon
Head of Developer Awareness


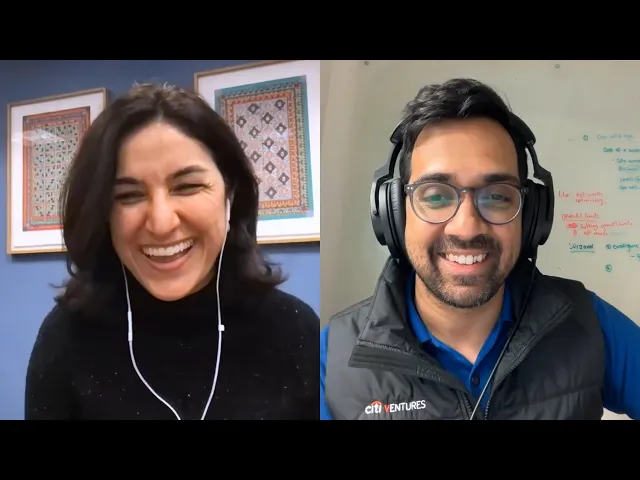
Technology is evolving at breakneck speed, and companies need AI solutions they can actually trust. Businesses want AI that boosts efficiency without compromising integrity or control while keeping data secure and complying with regulations like the EU AI Act. These themes took center stage in a recent Chain of Thought podcast episode.
The conversation brought together Conor Bronsdon, Galileo's Head of Developer Awareness and podcast host, with Atindriyo Sanyal, CTO and Co-Founder of Galileo, and Dr. Maryam Ashoori, Head of Product for watsonx AI at IBM.
Their discussion centered on AI integration in highly regulated industries like finance and healthcare sectors, balancing AI benefits against privacy concerns and strict compliance requirements.
The Necessity of Transparency and Governance in AI Platforms
For organizations in regulated industries, transparency and governance aren't optional features—they're foundational requirements. As AI applications proliferate in critical sectors, responsible implementation becomes the differentiating factor between systems that build trust and those that create liability.

Why Transparency Matters
The AI market has matured significantly in recent years, moving beyond initial excitement toward practical, enterprise-scale applications.
Dr. Ashoori observed this evolution firsthand: "The market has moved past that aha moment," emphasizing how serious applications now demand equally serious transparency frameworks. This transparency builds stakeholder trust by ensuring AI systems operate accurately, fairly, and consistently.
For heavily regulated industries, transparent AI operations directly impact compliance. Financial institutions and healthcare providers must maintain clear visibility into how their AI models arrive at decisions, requiring robust systems for monitoring and AI explainability to satisfy both ethical standards and regulatory scrutiny.
Governance as a Pillar of Trust
Effective governance in AI development provides the structural foundation upon which trustworthy AI systems are built.
Dr. Ashoori was unequivocal about its importance: "You cannot go to production without observability and governance. It's essential." Well-designed governance frameworks ensure AI systems remain aligned with organizational policies and legal requirements, substantially reducing deployment risks.
Comprehensive AI governance encompasses the entire model lifecycle, from initial training and validation through deployment, monitoring, and updates, incorporating practices like LLM observability.
This extends beyond merely establishing rules to actively supervising AI performance in real-world conditions, particularly critical in domains like banking or healthcare, where algorithmic decisions carry significant consequences.
As organizations scale their AI initiatives, they need governance strategies that thoroughly verify model integrity and performance across diverse applications. This verification builds genuine confidence among customers, regulators, and internal stakeholders—confidence that becomes increasingly valuable as AI systems tackle more complex and consequential tasks.
Strategies for Responsible AI Implementation
As enterprises transition from experimental AI to production-scale deployment, implementing responsible frameworks and effective AI risk management becomes non-negotiable. The discussion revealed several practical approaches to building ethical, accountable AI systems.
Initial Steps for Ethical AI Deployment
Ethical AI deployment begins with thorough model evaluation. Organizations need AI evaluation frameworks that assess AI systems across their entire lifecycle, examining both technical performance and ethical challenges in AI. IBM's governance platform plays a crucial role here, providing the monitoring capabilities and transparency necessary for regulatory compliance.
Dr. Ashoori outlined IBM's three-pillar approach to AI deployment: optimization, evaluating generative AI, and maximizing generative AI's return on investment. "These are the driving forces for what we are designing as part of the platform for watsonx," she explained.
This structured approach demonstrates how principles must translate into concrete practices that prioritize ethics and accountability.
Trust emerges as the cornerstone of responsible AI implementation. For IBM, whose clients often operate under intense regulatory scrutiny, building trustworthy systems means designing for transparency and accountability from the ground up.
This includes comprehensive documentation of data sources, training methodologies, and decision processes. Responsible AI also demands governance mechanisms that track models throughout their lifecycle, enabling users to trace and understand each action the system takes.
Human oversight represents another critical component, particularly in high-stakes environments. As Dr. Ashoori advised: "We need to have a mechanism where humans are in the loop and no actions are taken automatically when the stakes are high." This human supervision ensures ethical decision-making in contexts where AI determinations significantly impact individuals or organizations.
Build Effective Guardrails for Enterprise AI Systems
Properly designed guardrails and AI safety metrics prevent AI systems from making undesirable decisions or generating harmful content. Dr. Ashoori emphasized a comprehensive approach: "guardrails on input, guardrails on output, guardrails orchestrators." These safeguards keep AI actions within appropriate ethical boundaries, particularly important for high-stakes applications or newer systems like agentic AI.
For enterprise clients, guardrails serve multiple functions simultaneously. They protect organizational reputation by preventing biased or inappropriate outputs, ensuring compliance with industry-specific regulations, and maintaining data privacy standards.
IBM's approach incorporates these protections throughout the AI pipeline, from initial prompt engineering to final content filtering, guided by comprehensive AI security strategies.
The implementation of guardrails requires careful calibration. Overly restrictive controls can limit AI's problem-solving capabilities, while insufficient protections create unacceptable risks. The optimal solution involves configurable guardrails that adapt to each organization's risk tolerance and application context, allowing businesses to navigate compliance requirements without sacrificing innovation potential.
Document AI Model Lineage
As data volumes expand exponentially, ensuring data quality in AI and maintaining precise records of which data trained which models—and their resulting outputs—has become essential for trustworthy AI. This documentation creates an audit trail critical for both compliance and risk management.
Dr. Ashoori shared a compelling example from insurance: "I need to know exactly what version of what model was trained on what version of data." This level of detail enables companies to trace AI decisions back to their origins, vital for addressing regulatory inquiries or troubleshooting performance issues.
Model lineage documentation serves diverse stakeholders across the organization. It provides developers with insights into model evolution, gives compliance officers verification that AI systems meet requirements, and reduces executive liability by demonstrating due diligence in AI governance. IBM's watsonx platform incorporates these capabilities, creating persistent records that track models throughout their lifecycle while enabling version control when needed.
Beyond governance, watsonx exemplifies how enterprise AI platforms can balance innovation with security requirements. Dr. Ashoori highlighted the platform's ability to integrate open-source models while ensuring they remain governable and secure.
This approach gives organizations the flexibility to use cutting-edge models while maintaining enterprise-grade controls. "Companies want the best of both worlds – the innovation of open source with the security of enterprise-grade governance," Dr. Ashoori explained. This balanced approach has established IBM's platform as a benchmark for responsible AI deployment in regulated industries.
Balancing Innovation With Responsibility
As AI technologies continue to evolve at unprecedented speed, the next generation of AI systems will need to seamlessly integrate advanced capabilities with increasingly sophisticated governance frameworks. Organizations that master this balance will gain significant competitive advantages while mitigating the escalating risks associated with ungoverned AI deployment.
The regulatory landscape surrounding AI is also rapidly developing, with new frameworks emerging across different jurisdictions. Forward-thinking enterprises are preparing for this evolving environment by implementing flexible governance structures that can adapt to changing requirements.
Building adaptable governance capabilities today creates resilience against tomorrow's compliance challenges, particularly for organizations operating across multiple regulatory environments.
To directly address these emerging needs, solutions like Galileo empower organizations to implement AI with confidence across diverse industries, providing the crucial infrastructure for responsible innovation. These capabilities enable enterprises to maintain control and visibility across their AI systems while accelerating deployment.
To deepen your understanding of enterprise AI governance, listen to the complete Chain of Thought podcast for invaluable insights from specialists at the forefront of the field.
And check out the Chain of Thought podcast series, which continues to explore cutting-edge developments in generative AI through conversations with industry pioneers and practitioners, making it an essential resource for professionals seeking to stay ahead of the curve in responsible AI implementation.
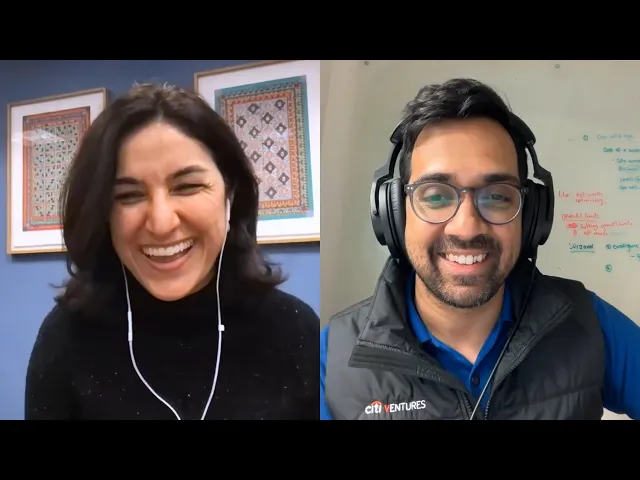
Technology is evolving at breakneck speed, and companies need AI solutions they can actually trust. Businesses want AI that boosts efficiency without compromising integrity or control while keeping data secure and complying with regulations like the EU AI Act. These themes took center stage in a recent Chain of Thought podcast episode.
The conversation brought together Conor Bronsdon, Galileo's Head of Developer Awareness and podcast host, with Atindriyo Sanyal, CTO and Co-Founder of Galileo, and Dr. Maryam Ashoori, Head of Product for watsonx AI at IBM.
Their discussion centered on AI integration in highly regulated industries like finance and healthcare sectors, balancing AI benefits against privacy concerns and strict compliance requirements.
The Necessity of Transparency and Governance in AI Platforms
For organizations in regulated industries, transparency and governance aren't optional features—they're foundational requirements. As AI applications proliferate in critical sectors, responsible implementation becomes the differentiating factor between systems that build trust and those that create liability.

Why Transparency Matters
The AI market has matured significantly in recent years, moving beyond initial excitement toward practical, enterprise-scale applications.
Dr. Ashoori observed this evolution firsthand: "The market has moved past that aha moment," emphasizing how serious applications now demand equally serious transparency frameworks. This transparency builds stakeholder trust by ensuring AI systems operate accurately, fairly, and consistently.
For heavily regulated industries, transparent AI operations directly impact compliance. Financial institutions and healthcare providers must maintain clear visibility into how their AI models arrive at decisions, requiring robust systems for monitoring and AI explainability to satisfy both ethical standards and regulatory scrutiny.
Governance as a Pillar of Trust
Effective governance in AI development provides the structural foundation upon which trustworthy AI systems are built.
Dr. Ashoori was unequivocal about its importance: "You cannot go to production without observability and governance. It's essential." Well-designed governance frameworks ensure AI systems remain aligned with organizational policies and legal requirements, substantially reducing deployment risks.
Comprehensive AI governance encompasses the entire model lifecycle, from initial training and validation through deployment, monitoring, and updates, incorporating practices like LLM observability.
This extends beyond merely establishing rules to actively supervising AI performance in real-world conditions, particularly critical in domains like banking or healthcare, where algorithmic decisions carry significant consequences.
As organizations scale their AI initiatives, they need governance strategies that thoroughly verify model integrity and performance across diverse applications. This verification builds genuine confidence among customers, regulators, and internal stakeholders—confidence that becomes increasingly valuable as AI systems tackle more complex and consequential tasks.
Strategies for Responsible AI Implementation
As enterprises transition from experimental AI to production-scale deployment, implementing responsible frameworks and effective AI risk management becomes non-negotiable. The discussion revealed several practical approaches to building ethical, accountable AI systems.
Initial Steps for Ethical AI Deployment
Ethical AI deployment begins with thorough model evaluation. Organizations need AI evaluation frameworks that assess AI systems across their entire lifecycle, examining both technical performance and ethical challenges in AI. IBM's governance platform plays a crucial role here, providing the monitoring capabilities and transparency necessary for regulatory compliance.
Dr. Ashoori outlined IBM's three-pillar approach to AI deployment: optimization, evaluating generative AI, and maximizing generative AI's return on investment. "These are the driving forces for what we are designing as part of the platform for watsonx," she explained.
This structured approach demonstrates how principles must translate into concrete practices that prioritize ethics and accountability.
Trust emerges as the cornerstone of responsible AI implementation. For IBM, whose clients often operate under intense regulatory scrutiny, building trustworthy systems means designing for transparency and accountability from the ground up.
This includes comprehensive documentation of data sources, training methodologies, and decision processes. Responsible AI also demands governance mechanisms that track models throughout their lifecycle, enabling users to trace and understand each action the system takes.
Human oversight represents another critical component, particularly in high-stakes environments. As Dr. Ashoori advised: "We need to have a mechanism where humans are in the loop and no actions are taken automatically when the stakes are high." This human supervision ensures ethical decision-making in contexts where AI determinations significantly impact individuals or organizations.
Build Effective Guardrails for Enterprise AI Systems
Properly designed guardrails and AI safety metrics prevent AI systems from making undesirable decisions or generating harmful content. Dr. Ashoori emphasized a comprehensive approach: "guardrails on input, guardrails on output, guardrails orchestrators." These safeguards keep AI actions within appropriate ethical boundaries, particularly important for high-stakes applications or newer systems like agentic AI.
For enterprise clients, guardrails serve multiple functions simultaneously. They protect organizational reputation by preventing biased or inappropriate outputs, ensuring compliance with industry-specific regulations, and maintaining data privacy standards.
IBM's approach incorporates these protections throughout the AI pipeline, from initial prompt engineering to final content filtering, guided by comprehensive AI security strategies.
The implementation of guardrails requires careful calibration. Overly restrictive controls can limit AI's problem-solving capabilities, while insufficient protections create unacceptable risks. The optimal solution involves configurable guardrails that adapt to each organization's risk tolerance and application context, allowing businesses to navigate compliance requirements without sacrificing innovation potential.
Document AI Model Lineage
As data volumes expand exponentially, ensuring data quality in AI and maintaining precise records of which data trained which models—and their resulting outputs—has become essential for trustworthy AI. This documentation creates an audit trail critical for both compliance and risk management.
Dr. Ashoori shared a compelling example from insurance: "I need to know exactly what version of what model was trained on what version of data." This level of detail enables companies to trace AI decisions back to their origins, vital for addressing regulatory inquiries or troubleshooting performance issues.
Model lineage documentation serves diverse stakeholders across the organization. It provides developers with insights into model evolution, gives compliance officers verification that AI systems meet requirements, and reduces executive liability by demonstrating due diligence in AI governance. IBM's watsonx platform incorporates these capabilities, creating persistent records that track models throughout their lifecycle while enabling version control when needed.
Beyond governance, watsonx exemplifies how enterprise AI platforms can balance innovation with security requirements. Dr. Ashoori highlighted the platform's ability to integrate open-source models while ensuring they remain governable and secure.
This approach gives organizations the flexibility to use cutting-edge models while maintaining enterprise-grade controls. "Companies want the best of both worlds – the innovation of open source with the security of enterprise-grade governance," Dr. Ashoori explained. This balanced approach has established IBM's platform as a benchmark for responsible AI deployment in regulated industries.
Balancing Innovation With Responsibility
As AI technologies continue to evolve at unprecedented speed, the next generation of AI systems will need to seamlessly integrate advanced capabilities with increasingly sophisticated governance frameworks. Organizations that master this balance will gain significant competitive advantages while mitigating the escalating risks associated with ungoverned AI deployment.
The regulatory landscape surrounding AI is also rapidly developing, with new frameworks emerging across different jurisdictions. Forward-thinking enterprises are preparing for this evolving environment by implementing flexible governance structures that can adapt to changing requirements.
Building adaptable governance capabilities today creates resilience against tomorrow's compliance challenges, particularly for organizations operating across multiple regulatory environments.
To directly address these emerging needs, solutions like Galileo empower organizations to implement AI with confidence across diverse industries, providing the crucial infrastructure for responsible innovation. These capabilities enable enterprises to maintain control and visibility across their AI systems while accelerating deployment.
To deepen your understanding of enterprise AI governance, listen to the complete Chain of Thought podcast for invaluable insights from specialists at the forefront of the field.
And check out the Chain of Thought podcast series, which continues to explore cutting-edge developments in generative AI through conversations with industry pioneers and practitioners, making it an essential resource for professionals seeking to stay ahead of the curve in responsible AI implementation.
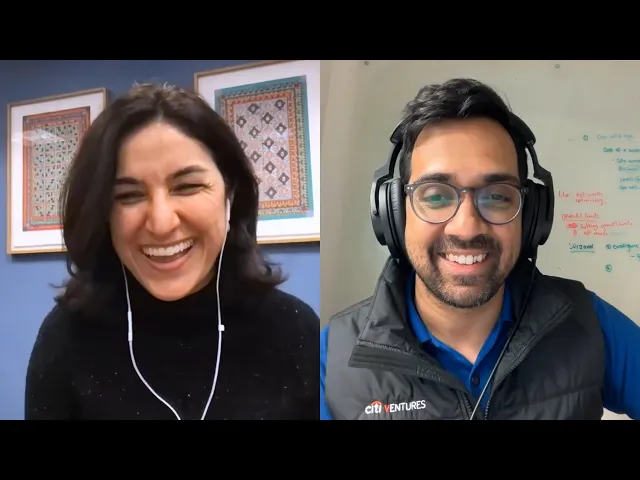
Technology is evolving at breakneck speed, and companies need AI solutions they can actually trust. Businesses want AI that boosts efficiency without compromising integrity or control while keeping data secure and complying with regulations like the EU AI Act. These themes took center stage in a recent Chain of Thought podcast episode.
The conversation brought together Conor Bronsdon, Galileo's Head of Developer Awareness and podcast host, with Atindriyo Sanyal, CTO and Co-Founder of Galileo, and Dr. Maryam Ashoori, Head of Product for watsonx AI at IBM.
Their discussion centered on AI integration in highly regulated industries like finance and healthcare sectors, balancing AI benefits against privacy concerns and strict compliance requirements.
The Necessity of Transparency and Governance in AI Platforms
For organizations in regulated industries, transparency and governance aren't optional features—they're foundational requirements. As AI applications proliferate in critical sectors, responsible implementation becomes the differentiating factor between systems that build trust and those that create liability.

Why Transparency Matters
The AI market has matured significantly in recent years, moving beyond initial excitement toward practical, enterprise-scale applications.
Dr. Ashoori observed this evolution firsthand: "The market has moved past that aha moment," emphasizing how serious applications now demand equally serious transparency frameworks. This transparency builds stakeholder trust by ensuring AI systems operate accurately, fairly, and consistently.
For heavily regulated industries, transparent AI operations directly impact compliance. Financial institutions and healthcare providers must maintain clear visibility into how their AI models arrive at decisions, requiring robust systems for monitoring and AI explainability to satisfy both ethical standards and regulatory scrutiny.
Governance as a Pillar of Trust
Effective governance in AI development provides the structural foundation upon which trustworthy AI systems are built.
Dr. Ashoori was unequivocal about its importance: "You cannot go to production without observability and governance. It's essential." Well-designed governance frameworks ensure AI systems remain aligned with organizational policies and legal requirements, substantially reducing deployment risks.
Comprehensive AI governance encompasses the entire model lifecycle, from initial training and validation through deployment, monitoring, and updates, incorporating practices like LLM observability.
This extends beyond merely establishing rules to actively supervising AI performance in real-world conditions, particularly critical in domains like banking or healthcare, where algorithmic decisions carry significant consequences.
As organizations scale their AI initiatives, they need governance strategies that thoroughly verify model integrity and performance across diverse applications. This verification builds genuine confidence among customers, regulators, and internal stakeholders—confidence that becomes increasingly valuable as AI systems tackle more complex and consequential tasks.
Strategies for Responsible AI Implementation
As enterprises transition from experimental AI to production-scale deployment, implementing responsible frameworks and effective AI risk management becomes non-negotiable. The discussion revealed several practical approaches to building ethical, accountable AI systems.
Initial Steps for Ethical AI Deployment
Ethical AI deployment begins with thorough model evaluation. Organizations need AI evaluation frameworks that assess AI systems across their entire lifecycle, examining both technical performance and ethical challenges in AI. IBM's governance platform plays a crucial role here, providing the monitoring capabilities and transparency necessary for regulatory compliance.
Dr. Ashoori outlined IBM's three-pillar approach to AI deployment: optimization, evaluating generative AI, and maximizing generative AI's return on investment. "These are the driving forces for what we are designing as part of the platform for watsonx," she explained.
This structured approach demonstrates how principles must translate into concrete practices that prioritize ethics and accountability.
Trust emerges as the cornerstone of responsible AI implementation. For IBM, whose clients often operate under intense regulatory scrutiny, building trustworthy systems means designing for transparency and accountability from the ground up.
This includes comprehensive documentation of data sources, training methodologies, and decision processes. Responsible AI also demands governance mechanisms that track models throughout their lifecycle, enabling users to trace and understand each action the system takes.
Human oversight represents another critical component, particularly in high-stakes environments. As Dr. Ashoori advised: "We need to have a mechanism where humans are in the loop and no actions are taken automatically when the stakes are high." This human supervision ensures ethical decision-making in contexts where AI determinations significantly impact individuals or organizations.
Build Effective Guardrails for Enterprise AI Systems
Properly designed guardrails and AI safety metrics prevent AI systems from making undesirable decisions or generating harmful content. Dr. Ashoori emphasized a comprehensive approach: "guardrails on input, guardrails on output, guardrails orchestrators." These safeguards keep AI actions within appropriate ethical boundaries, particularly important for high-stakes applications or newer systems like agentic AI.
For enterprise clients, guardrails serve multiple functions simultaneously. They protect organizational reputation by preventing biased or inappropriate outputs, ensuring compliance with industry-specific regulations, and maintaining data privacy standards.
IBM's approach incorporates these protections throughout the AI pipeline, from initial prompt engineering to final content filtering, guided by comprehensive AI security strategies.
The implementation of guardrails requires careful calibration. Overly restrictive controls can limit AI's problem-solving capabilities, while insufficient protections create unacceptable risks. The optimal solution involves configurable guardrails that adapt to each organization's risk tolerance and application context, allowing businesses to navigate compliance requirements without sacrificing innovation potential.
Document AI Model Lineage
As data volumes expand exponentially, ensuring data quality in AI and maintaining precise records of which data trained which models—and their resulting outputs—has become essential for trustworthy AI. This documentation creates an audit trail critical for both compliance and risk management.
Dr. Ashoori shared a compelling example from insurance: "I need to know exactly what version of what model was trained on what version of data." This level of detail enables companies to trace AI decisions back to their origins, vital for addressing regulatory inquiries or troubleshooting performance issues.
Model lineage documentation serves diverse stakeholders across the organization. It provides developers with insights into model evolution, gives compliance officers verification that AI systems meet requirements, and reduces executive liability by demonstrating due diligence in AI governance. IBM's watsonx platform incorporates these capabilities, creating persistent records that track models throughout their lifecycle while enabling version control when needed.
Beyond governance, watsonx exemplifies how enterprise AI platforms can balance innovation with security requirements. Dr. Ashoori highlighted the platform's ability to integrate open-source models while ensuring they remain governable and secure.
This approach gives organizations the flexibility to use cutting-edge models while maintaining enterprise-grade controls. "Companies want the best of both worlds – the innovation of open source with the security of enterprise-grade governance," Dr. Ashoori explained. This balanced approach has established IBM's platform as a benchmark for responsible AI deployment in regulated industries.
Balancing Innovation With Responsibility
As AI technologies continue to evolve at unprecedented speed, the next generation of AI systems will need to seamlessly integrate advanced capabilities with increasingly sophisticated governance frameworks. Organizations that master this balance will gain significant competitive advantages while mitigating the escalating risks associated with ungoverned AI deployment.
The regulatory landscape surrounding AI is also rapidly developing, with new frameworks emerging across different jurisdictions. Forward-thinking enterprises are preparing for this evolving environment by implementing flexible governance structures that can adapt to changing requirements.
Building adaptable governance capabilities today creates resilience against tomorrow's compliance challenges, particularly for organizations operating across multiple regulatory environments.
To directly address these emerging needs, solutions like Galileo empower organizations to implement AI with confidence across diverse industries, providing the crucial infrastructure for responsible innovation. These capabilities enable enterprises to maintain control and visibility across their AI systems while accelerating deployment.
To deepen your understanding of enterprise AI governance, listen to the complete Chain of Thought podcast for invaluable insights from specialists at the forefront of the field.
And check out the Chain of Thought podcast series, which continues to explore cutting-edge developments in generative AI through conversations with industry pioneers and practitioners, making it an essential resource for professionals seeking to stay ahead of the curve in responsible AI implementation.
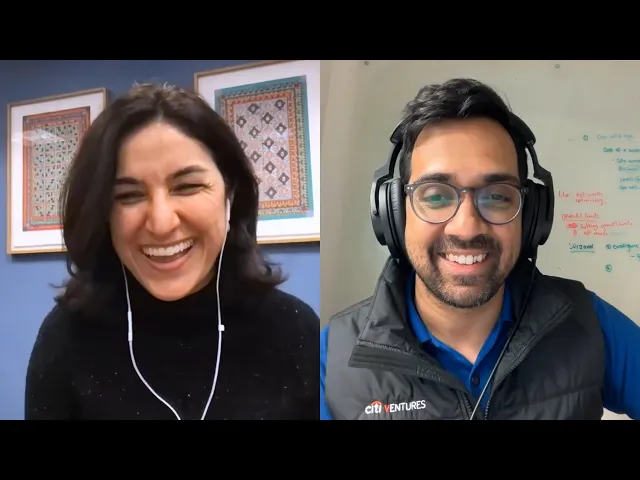
Technology is evolving at breakneck speed, and companies need AI solutions they can actually trust. Businesses want AI that boosts efficiency without compromising integrity or control while keeping data secure and complying with regulations like the EU AI Act. These themes took center stage in a recent Chain of Thought podcast episode.
The conversation brought together Conor Bronsdon, Galileo's Head of Developer Awareness and podcast host, with Atindriyo Sanyal, CTO and Co-Founder of Galileo, and Dr. Maryam Ashoori, Head of Product for watsonx AI at IBM.
Their discussion centered on AI integration in highly regulated industries like finance and healthcare sectors, balancing AI benefits against privacy concerns and strict compliance requirements.
The Necessity of Transparency and Governance in AI Platforms
For organizations in regulated industries, transparency and governance aren't optional features—they're foundational requirements. As AI applications proliferate in critical sectors, responsible implementation becomes the differentiating factor between systems that build trust and those that create liability.

Why Transparency Matters
The AI market has matured significantly in recent years, moving beyond initial excitement toward practical, enterprise-scale applications.
Dr. Ashoori observed this evolution firsthand: "The market has moved past that aha moment," emphasizing how serious applications now demand equally serious transparency frameworks. This transparency builds stakeholder trust by ensuring AI systems operate accurately, fairly, and consistently.
For heavily regulated industries, transparent AI operations directly impact compliance. Financial institutions and healthcare providers must maintain clear visibility into how their AI models arrive at decisions, requiring robust systems for monitoring and AI explainability to satisfy both ethical standards and regulatory scrutiny.
Governance as a Pillar of Trust
Effective governance in AI development provides the structural foundation upon which trustworthy AI systems are built.
Dr. Ashoori was unequivocal about its importance: "You cannot go to production without observability and governance. It's essential." Well-designed governance frameworks ensure AI systems remain aligned with organizational policies and legal requirements, substantially reducing deployment risks.
Comprehensive AI governance encompasses the entire model lifecycle, from initial training and validation through deployment, monitoring, and updates, incorporating practices like LLM observability.
This extends beyond merely establishing rules to actively supervising AI performance in real-world conditions, particularly critical in domains like banking or healthcare, where algorithmic decisions carry significant consequences.
As organizations scale their AI initiatives, they need governance strategies that thoroughly verify model integrity and performance across diverse applications. This verification builds genuine confidence among customers, regulators, and internal stakeholders—confidence that becomes increasingly valuable as AI systems tackle more complex and consequential tasks.
Strategies for Responsible AI Implementation
As enterprises transition from experimental AI to production-scale deployment, implementing responsible frameworks and effective AI risk management becomes non-negotiable. The discussion revealed several practical approaches to building ethical, accountable AI systems.
Initial Steps for Ethical AI Deployment
Ethical AI deployment begins with thorough model evaluation. Organizations need AI evaluation frameworks that assess AI systems across their entire lifecycle, examining both technical performance and ethical challenges in AI. IBM's governance platform plays a crucial role here, providing the monitoring capabilities and transparency necessary for regulatory compliance.
Dr. Ashoori outlined IBM's three-pillar approach to AI deployment: optimization, evaluating generative AI, and maximizing generative AI's return on investment. "These are the driving forces for what we are designing as part of the platform for watsonx," she explained.
This structured approach demonstrates how principles must translate into concrete practices that prioritize ethics and accountability.
Trust emerges as the cornerstone of responsible AI implementation. For IBM, whose clients often operate under intense regulatory scrutiny, building trustworthy systems means designing for transparency and accountability from the ground up.
This includes comprehensive documentation of data sources, training methodologies, and decision processes. Responsible AI also demands governance mechanisms that track models throughout their lifecycle, enabling users to trace and understand each action the system takes.
Human oversight represents another critical component, particularly in high-stakes environments. As Dr. Ashoori advised: "We need to have a mechanism where humans are in the loop and no actions are taken automatically when the stakes are high." This human supervision ensures ethical decision-making in contexts where AI determinations significantly impact individuals or organizations.
Build Effective Guardrails for Enterprise AI Systems
Properly designed guardrails and AI safety metrics prevent AI systems from making undesirable decisions or generating harmful content. Dr. Ashoori emphasized a comprehensive approach: "guardrails on input, guardrails on output, guardrails orchestrators." These safeguards keep AI actions within appropriate ethical boundaries, particularly important for high-stakes applications or newer systems like agentic AI.
For enterprise clients, guardrails serve multiple functions simultaneously. They protect organizational reputation by preventing biased or inappropriate outputs, ensuring compliance with industry-specific regulations, and maintaining data privacy standards.
IBM's approach incorporates these protections throughout the AI pipeline, from initial prompt engineering to final content filtering, guided by comprehensive AI security strategies.
The implementation of guardrails requires careful calibration. Overly restrictive controls can limit AI's problem-solving capabilities, while insufficient protections create unacceptable risks. The optimal solution involves configurable guardrails that adapt to each organization's risk tolerance and application context, allowing businesses to navigate compliance requirements without sacrificing innovation potential.
Document AI Model Lineage
As data volumes expand exponentially, ensuring data quality in AI and maintaining precise records of which data trained which models—and their resulting outputs—has become essential for trustworthy AI. This documentation creates an audit trail critical for both compliance and risk management.
Dr. Ashoori shared a compelling example from insurance: "I need to know exactly what version of what model was trained on what version of data." This level of detail enables companies to trace AI decisions back to their origins, vital for addressing regulatory inquiries or troubleshooting performance issues.
Model lineage documentation serves diverse stakeholders across the organization. It provides developers with insights into model evolution, gives compliance officers verification that AI systems meet requirements, and reduces executive liability by demonstrating due diligence in AI governance. IBM's watsonx platform incorporates these capabilities, creating persistent records that track models throughout their lifecycle while enabling version control when needed.
Beyond governance, watsonx exemplifies how enterprise AI platforms can balance innovation with security requirements. Dr. Ashoori highlighted the platform's ability to integrate open-source models while ensuring they remain governable and secure.
This approach gives organizations the flexibility to use cutting-edge models while maintaining enterprise-grade controls. "Companies want the best of both worlds – the innovation of open source with the security of enterprise-grade governance," Dr. Ashoori explained. This balanced approach has established IBM's platform as a benchmark for responsible AI deployment in regulated industries.
Balancing Innovation With Responsibility
As AI technologies continue to evolve at unprecedented speed, the next generation of AI systems will need to seamlessly integrate advanced capabilities with increasingly sophisticated governance frameworks. Organizations that master this balance will gain significant competitive advantages while mitigating the escalating risks associated with ungoverned AI deployment.
The regulatory landscape surrounding AI is also rapidly developing, with new frameworks emerging across different jurisdictions. Forward-thinking enterprises are preparing for this evolving environment by implementing flexible governance structures that can adapt to changing requirements.
Building adaptable governance capabilities today creates resilience against tomorrow's compliance challenges, particularly for organizations operating across multiple regulatory environments.
To directly address these emerging needs, solutions like Galileo empower organizations to implement AI with confidence across diverse industries, providing the crucial infrastructure for responsible innovation. These capabilities enable enterprises to maintain control and visibility across their AI systems while accelerating deployment.
To deepen your understanding of enterprise AI governance, listen to the complete Chain of Thought podcast for invaluable insights from specialists at the forefront of the field.
And check out the Chain of Thought podcast series, which continues to explore cutting-edge developments in generative AI through conversations with industry pioneers and practitioners, making it an essential resource for professionals seeking to stay ahead of the curve in responsible AI implementation.
Conor Bronsdon
Conor Bronsdon
Conor Bronsdon
Conor Bronsdon