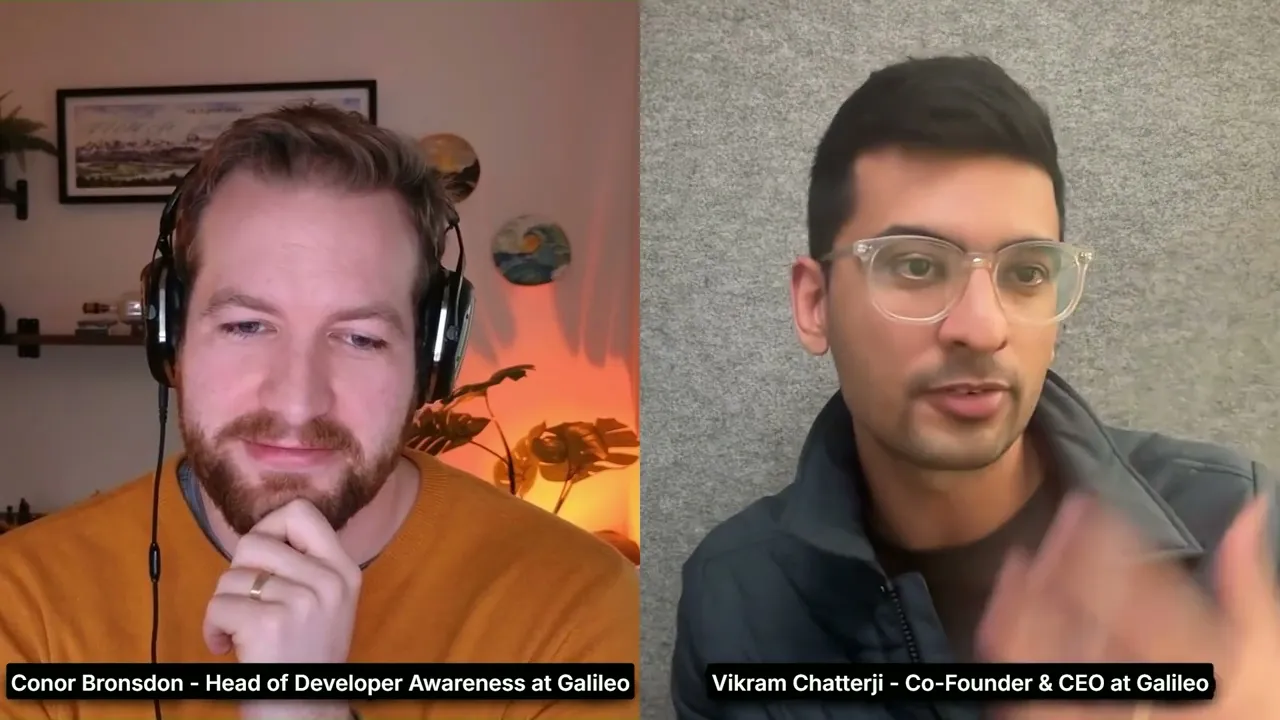
Mar 9, 2025
Functional Correctness in Modern AI: What It Is and Why It Matters


Conor Bronsdon
Head of Developer Awareness
Conor Bronsdon
Head of Developer Awareness


Your AI model passes test cases, yet in production, it consistently makes decisions that leave your team scratching their heads. Sound familiar? This disconnect between expected and actual behavior isn't just frustrating—it's a critical challenge in AI development that stems from gaps in functional correctness.
Whether it's a medical diagnostic tool delivering precise treatment recommendations or a financial system safeguarding fair and compliant loan approvals, functional correctness upholds the integrity of AI applications, boosts user trust, and allows for more advanced innovations.
This guide will explore how leading organizations are mastering functional correctness to build AI systems that not only work in theory but also deliver reliable, consistent results in real-world applications.
We recently explored this topic on our Chain of Thought podcast, where industry experts shared practical insights and real-world implementation strategies.
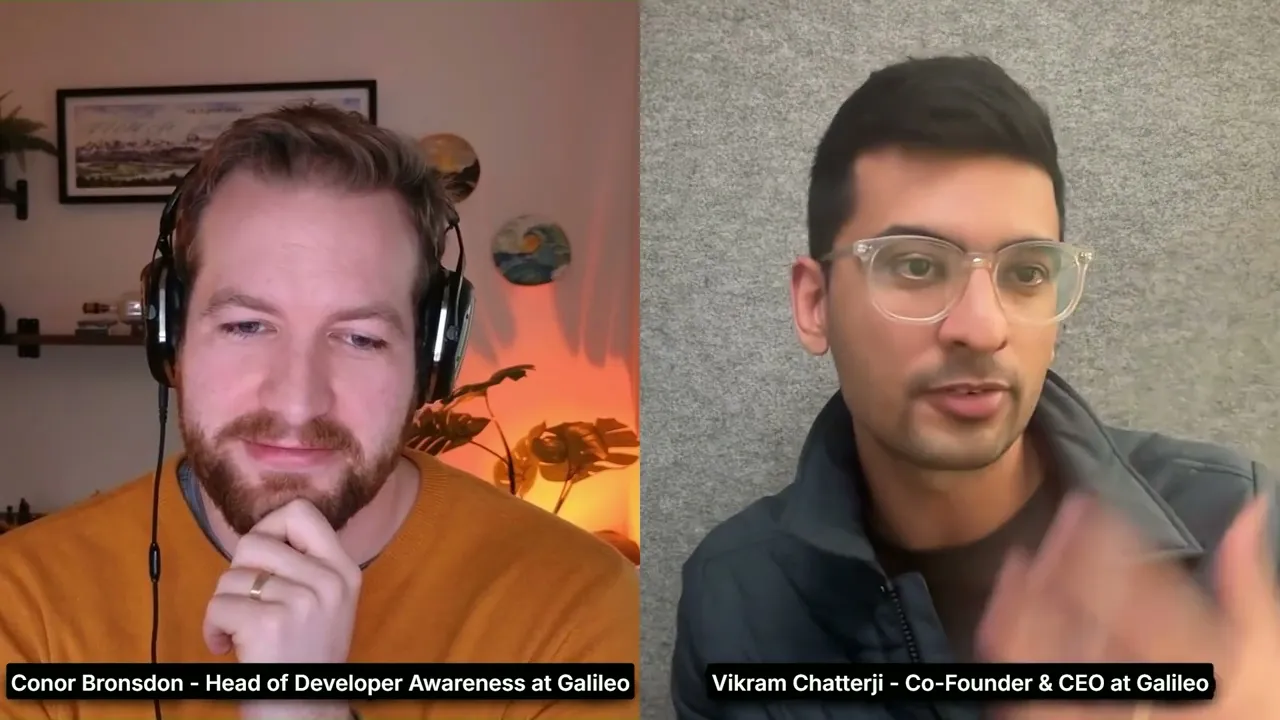
What is Functional Correctness in AI?
At its core, functional correctness represents the fundamental requirement that AI systems behave exactly as specified—not just in controlled environments but also in the complex, unpredictable world of production deployments.
Although this may seem straightforward, ensuring accurate functional correctness in AI systems poses unique challenges that distinguish it from traditional software testing.
Technical Foundations and Evolution from Traditional Metrics
Functional correctness in AI ensures systems perform precisely as intended across all scenarios. Unlike traditional software with precise inputs and outputs, AI operates in dynamic environments, requiring sophisticated validation processes to confirm models meet their requirements and behave predictably.
In safety-critical applications such as autonomous vehicles and healthcare, achieving accuracy benchmarks is only part of the equation—formal verification mechanisms are crucial to ensure that AI performs its designated tasks accurately. While traditional metrics like accuracy, precision, recall, and F1 scores provide valuable insights, they do not fully capture whether an AI system behaves correctly in varying conditions.
This limitation has driven a shift toward comprehensive evaluation frameworks, including advanced methods for evaluating AI agents that blend traditional metrics with robustness, adaptability, and ethical considerations. This evolution ensures systems are not just statistically performant but functionally correct and reliable for enterprise applications.
Real-World Impact on Production AI Systems
The emphasis on functional correctness leads to tangible improvements in production environments. When AI systems function properly, organizations experience enhanced decision-making quality, reduced operational risks, and increased stakeholder trust. Recent research on AI acceptance in healthcare emphasizes this point, demonstrating how reliable AI systems establish crucial trust among medical professionals.
Moreover, these rigorous evaluation frameworks, including real-world AI evaluation, don't just prevent failures—they actively contribute to successful AI deployments by ensuring functionalities align with real-world demands.
For instance, research in autonomous driving demonstrates how explainable AI and functional correctness work together to enhance system reliability and user confidence. As AI becomes increasingly central to business operations, this understanding of functional correctness serves as a crucial safeguard while maximizing the return on AI investments.

Functional Correctness in AI: Complex Evaluation Scenarios and Trade-offs
Ensuring functional correctness in AI systems requires navigating complex trade-offs between competing demands for effective AI risk management. Grasping these trade-offs is essential for developing robust LLM evaluation frameworks that balance various needs while maintaining system reliability.
Let’s see these evaluation trade-offs that go beyond basic testing paradigms.
Non-Deterministic Output Assessment
The challenge of evaluating non-deterministic outputs represents one of the fundamental trade-offs in AI functional correctness. Unlike traditional software, where identical inputs should produce identical outputs, AI systems—particularly LLMs may generate different but equally valid responses to the same prompt.
This variability creates a critical trade-off between consistency and creativity. Strict LLM evaluation metrics and frameworks that require exact output matching could hinder the AI's ability to generate novel, contextually appropriate responses. Conversely, excessive flexibility in evaluation criteria risks accepting incorrect or inappropriate outputs.
Consider a customer service AI: while we want consistent responses to similar queries, enforcing strict response templates could prevent the system from adapting to unique customer needs. The trade-off lies in balancing response variability with reliability.
Therefore, practical evaluation approaches must:
Define acceptable bounds for output variation
Measure semantic similarity rather than exact matches
Assess the consistency of reasoning patterns
Evaluate the impact on end-user experience and business outcomes
This balancing act requires sophisticated metrics that distinguish between beneficial creativity and harmful inconsistency while maintaining precise standards for functional correctness.
Context-Dependent Correctness
Context-dependent correctness presents a fundamental tension between generalization and specialization in AI systems. As operating environments become more complex, we face an increasingly tricky trade-off between building systems that work broadly and those that excel in specific contexts.
Take autonomous vehicles as an example: a vision system might achieve high accuracy in ideal conditions but struggle during harsh weather. The trade-off emerges between optimizing for perfect performance in common scenarios versus maintaining acceptable performance across all conditions. Pursuing both simultaneously often leads to increased computational costs and system complexity.
This creates several critical balancing acts:
Breadth vs. depth of context handling
Processing speed vs. contextual analysis depth
Resource utilization vs. environmental adaptation
Model complexity vs. maintainability
Real-world implementations must carefully navigate these trade-offs. For example, research on autonomous driving systems demonstrates that achieving robust performance across diverse contexts often requires sophisticated sensor fusion and redundant processing paths—methods that directly influence system cost and complexity.
Evaluation frameworks must therefore assess:
Performance stability across context shifts
Degradation patterns in challenging conditions
Resource costs of context adaptation
Recovery behavior when conditions improve
The key lies in finding the sweet spot where context awareness provides meaningful benefits without creating unsustainable computational or maintenance overhead.
Temporal Consistency in Responses
Temporal consistency introduces a critical trade-off between adaptability and stability in AI systems. As operational environments evolve over time, systems must balance maintaining reliable performance against adapting to new patterns and information.
This tension is particularly evident in financial AI systems. Consider a trading algorithm: it must adapt to changing market conditions while maintaining consistent decision-making logic to prevent erratic behavior. Too much emphasis on consistency could make the system rigid and unable to capture new market patterns, while excessive adaptability might lead to unstable or unpredictable trading strategies.
The core trade-offs include:
Short-term accuracy vs. long-term stability
Quick adaptation vs. reliable performance
Historical pattern preservation vs. new pattern recognition
Computational efficiency vs. temporal analysis depth
Effective evaluation strategies must therefore assess:
Drift patterns in system behavior
Adaptation speed to significant changes
Stability of core decision logic
Impact of temporal adjustments on overall reliability
This delicate balance becomes especially critical in high-stakes domains where both consistency and adaptability directly impact business outcomes.
Ground Truth Ambiguity
Perhaps the most complex trade-off in functional correctness involves scenarios where ground truth itself is uncertain or contested. This creates tension between precision and flexibility in evaluation frameworks.
Medical diagnosis systems exemplify this challenge. When expert opinions diverge on complex cases, the system must balance between making definitive recommendations and acknowledging diagnostic uncertainty. The trade-off lies in maintaining decision confidence while appropriately handling ambiguous cases.
Key tensions include:
Confidence vs. uncertainty communication
Precision vs. inclusivity in valid responses
Processing speed vs. thorough analysis
Resource allocation vs. comprehensive evaluation
Evaluation approaches must therefore:
Incorporate multiple expert perspectives
Weight-varying levels of certainty
Assess reasoning quality beyond outcomes
Balance decisiveness with appropriate caution
Success in handling these trade-offs requires sophisticated evaluation frameworks, including monitoring RAG performance metrics that can accommodate ambiguity while maintaining practical utility for end users.
Five Challenges of Ensuring Functional Correctness in AI (and Their Solutions)
As organizations scale their AI deployments, they face critical challenges in maintaining functional correctness, including common AI agent issues. While these challenges are complex, understanding them—and their solutions—is essential for building reliable AI systems that perform consistently in production environments.
Let's see how traditional approaches have evolved and how modern platforms like Galileo are supercharging these solutions.
Data Quality and Evaluation Precision
Production AI systems often struggle with data quality issues that impact functional correctness and evaluation accuracy. Ensuring the use of high-quality data is crucial in maintaining system performance. Implementing effective ML data intelligence strategies helps in achieving this goal. Even minor data inconsistencies can lead to significant evaluation errors in critical applications like healthcare diagnostics or financial risk assessment, highlighting the high-quality data necessity in AI systems.
Traditional approaches relied heavily on manual validation and basic statistical checks. While these methods helped identify apparent issues, they proved inadequate for complex AI models that require nuanced evaluation across multiple dimensions.
Galileo's Evaluate module tackles this challenge head-on by introducing automated quality assessment and continuous validation pipelines. It uses proprietary metrics, combining research-backed guardrail metrics with custom metrics, to provide comprehensive evaluation coverage and automatically detect data quality issues that could affect model performance. This supports rapid, collaborative experimentation and evaluation of LLM applications.
Production Monitoring at Scale
Production AI systems often struggle with data quality issues that impact functional correctness and evaluation accuracy. Ensuring the use of high-quality data is crucial in maintaining system performance. Implementing effective ML data intelligence strategies helps in achieving this goal. Even minor data inconsistencies can lead to significant evaluation errors in critical applications like healthcare diagnostics or financial risk assessment, highlighting the high-quality data necessity in AI systems.
Traditional approaches relied heavily on manual validation and basic statistical checks. While these methods helped identify apparent issues, they proved inadequate for complex AI models that require nuanced evaluation across multiple dimensions.
Galileo's Evaluate module tackles this challenge head-on by introducing automated quality assessment and continuous validation pipelines. It uses proprietary metrics, combining research-backed guardrail metrics with custom metrics, to provide comprehensive evaluation coverage and automatically detect data quality issues that could affect model performance. This supports rapid, collaborative experimentation and evaluation of LLM applications.
Security and Compliance in Evaluation
Maintaining functional correctness while ensuring compliance presents a significant challenge in highly regulated industries. AI systems must not only perform accurately but also adhere to strict regulatory requirements regarding data privacy, fairness, and transparency.
Traditional compliance approaches often involved separate audit processes and manual compliance checks. While thorough, these methods created operational bottlenecks and struggled to keep pace with rapidly evolving AI systems and regulatory landscapes.
This is where Galileo's Protect module comes in, integrating compliance into the evaluation process itself. It actively monitors outputs for potential compliance violations while maintaining audit trails of model behavior. The module uses Galileo's Guardrail Metrics to ensure its effectiveness in production environments. This integrated approach ensures that functional correctness and regulatory compliance work hand in hand rather than as competing priorities.
Domain-Specific Performance
AI systems deployed across different industries face unique challenges in maintaining functional correctness within their specific domains. A model that performs well in general scenarios may fail to capture crucial domain-specific nuances in fields like legal document analysis or medical diagnostics.
Conventional solutions typically involved creating separate evaluation frameworks for each domain—a resource-intensive approach that often led to inconsistent standards and difficulty in maintaining best practices across different applications.
Galileo's evaluation and optimization framework offers domain-adaptive evaluation capabilities with flexible metrics and evaluation criteria tailored to industry-specific benchmarks. It includes user-defined custom metrics and built-in Guardrail Metrics to assess application quality, such as measuring hallucinations through Context Adherence metrics.
Response Drift Detection
Perhaps the most insidious challenge to functional correctness is response drift—where AI system outputs gradually deviate from expected behavior over time. This drift often occurs so subtly that traditional monitoring methods fail to detect it until significant issues arise.
Historical approaches to drift detection relied on periodic model retraining and manual performance reviews. These methods frequently resulted in delayed responses to emerging issues and unnecessary retraining cycles when the drift wasn't present.
The modern solution? Galileo tackles this challenge through continuous drift monitoring and predictive analytics. The platform uses an advanced embedding-based, non-parametric nearest neighbor algorithm to detect out-of-distribution data, including drifted and out-of-coverage samples.
Moreover, this method capitalizes on the semantic structure in neural network embeddings, offering flexibility and ensuring that functional correctness remains stable over time while optimizing resource allocation for model maintenance.
By tackling these challenges with innovative solutions, Galileo enables enterprises to ensure functional correctness in AI systems, enhancing reliability and promoting scalable advancements.
Optimizing Functional Correctness in AI With Galileo
AI models and systems require meticulous attention to functional correctness, ensuring these systems operate as expected. Understanding functional correctness in AI involves aligning AI decision-making processes with predefined business objectives.
Galileo stands out as a pivotal tool in achieving this alignment in AI systems. The platform supports comprehensive end-to-end observability, enabling enterprises to track every facet of an AI system's operation—from plan generation using LLMs to task execution.
Ready to enhance your AI system's functional correctness? Get started with Galileo GenAI Studio today and experience the difference in your AI evaluation process.
Your AI model passes test cases, yet in production, it consistently makes decisions that leave your team scratching their heads. Sound familiar? This disconnect between expected and actual behavior isn't just frustrating—it's a critical challenge in AI development that stems from gaps in functional correctness.
Whether it's a medical diagnostic tool delivering precise treatment recommendations or a financial system safeguarding fair and compliant loan approvals, functional correctness upholds the integrity of AI applications, boosts user trust, and allows for more advanced innovations.
This guide will explore how leading organizations are mastering functional correctness to build AI systems that not only work in theory but also deliver reliable, consistent results in real-world applications.
We recently explored this topic on our Chain of Thought podcast, where industry experts shared practical insights and real-world implementation strategies.
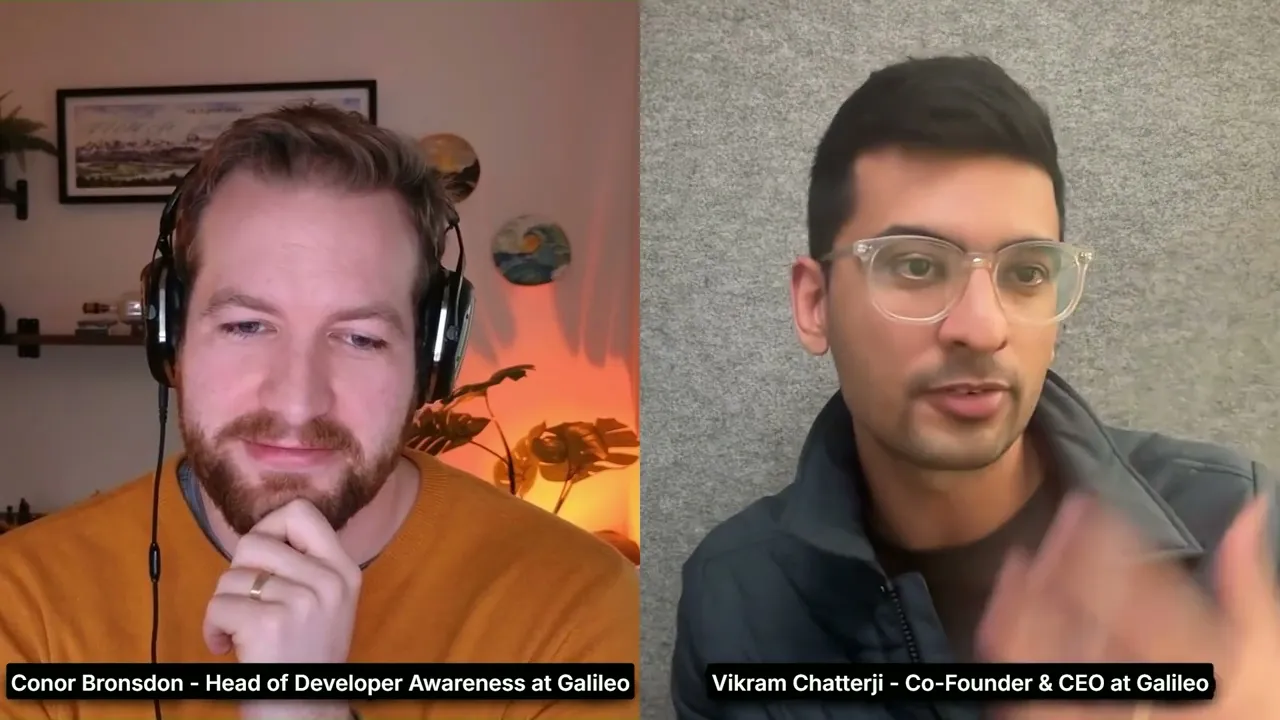
What is Functional Correctness in AI?
At its core, functional correctness represents the fundamental requirement that AI systems behave exactly as specified—not just in controlled environments but also in the complex, unpredictable world of production deployments.
Although this may seem straightforward, ensuring accurate functional correctness in AI systems poses unique challenges that distinguish it from traditional software testing.
Technical Foundations and Evolution from Traditional Metrics
Functional correctness in AI ensures systems perform precisely as intended across all scenarios. Unlike traditional software with precise inputs and outputs, AI operates in dynamic environments, requiring sophisticated validation processes to confirm models meet their requirements and behave predictably.
In safety-critical applications such as autonomous vehicles and healthcare, achieving accuracy benchmarks is only part of the equation—formal verification mechanisms are crucial to ensure that AI performs its designated tasks accurately. While traditional metrics like accuracy, precision, recall, and F1 scores provide valuable insights, they do not fully capture whether an AI system behaves correctly in varying conditions.
This limitation has driven a shift toward comprehensive evaluation frameworks, including advanced methods for evaluating AI agents that blend traditional metrics with robustness, adaptability, and ethical considerations. This evolution ensures systems are not just statistically performant but functionally correct and reliable for enterprise applications.
Real-World Impact on Production AI Systems
The emphasis on functional correctness leads to tangible improvements in production environments. When AI systems function properly, organizations experience enhanced decision-making quality, reduced operational risks, and increased stakeholder trust. Recent research on AI acceptance in healthcare emphasizes this point, demonstrating how reliable AI systems establish crucial trust among medical professionals.
Moreover, these rigorous evaluation frameworks, including real-world AI evaluation, don't just prevent failures—they actively contribute to successful AI deployments by ensuring functionalities align with real-world demands.
For instance, research in autonomous driving demonstrates how explainable AI and functional correctness work together to enhance system reliability and user confidence. As AI becomes increasingly central to business operations, this understanding of functional correctness serves as a crucial safeguard while maximizing the return on AI investments.

Functional Correctness in AI: Complex Evaluation Scenarios and Trade-offs
Ensuring functional correctness in AI systems requires navigating complex trade-offs between competing demands for effective AI risk management. Grasping these trade-offs is essential for developing robust LLM evaluation frameworks that balance various needs while maintaining system reliability.
Let’s see these evaluation trade-offs that go beyond basic testing paradigms.
Non-Deterministic Output Assessment
The challenge of evaluating non-deterministic outputs represents one of the fundamental trade-offs in AI functional correctness. Unlike traditional software, where identical inputs should produce identical outputs, AI systems—particularly LLMs may generate different but equally valid responses to the same prompt.
This variability creates a critical trade-off between consistency and creativity. Strict LLM evaluation metrics and frameworks that require exact output matching could hinder the AI's ability to generate novel, contextually appropriate responses. Conversely, excessive flexibility in evaluation criteria risks accepting incorrect or inappropriate outputs.
Consider a customer service AI: while we want consistent responses to similar queries, enforcing strict response templates could prevent the system from adapting to unique customer needs. The trade-off lies in balancing response variability with reliability.
Therefore, practical evaluation approaches must:
Define acceptable bounds for output variation
Measure semantic similarity rather than exact matches
Assess the consistency of reasoning patterns
Evaluate the impact on end-user experience and business outcomes
This balancing act requires sophisticated metrics that distinguish between beneficial creativity and harmful inconsistency while maintaining precise standards for functional correctness.
Context-Dependent Correctness
Context-dependent correctness presents a fundamental tension between generalization and specialization in AI systems. As operating environments become more complex, we face an increasingly tricky trade-off between building systems that work broadly and those that excel in specific contexts.
Take autonomous vehicles as an example: a vision system might achieve high accuracy in ideal conditions but struggle during harsh weather. The trade-off emerges between optimizing for perfect performance in common scenarios versus maintaining acceptable performance across all conditions. Pursuing both simultaneously often leads to increased computational costs and system complexity.
This creates several critical balancing acts:
Breadth vs. depth of context handling
Processing speed vs. contextual analysis depth
Resource utilization vs. environmental adaptation
Model complexity vs. maintainability
Real-world implementations must carefully navigate these trade-offs. For example, research on autonomous driving systems demonstrates that achieving robust performance across diverse contexts often requires sophisticated sensor fusion and redundant processing paths—methods that directly influence system cost and complexity.
Evaluation frameworks must therefore assess:
Performance stability across context shifts
Degradation patterns in challenging conditions
Resource costs of context adaptation
Recovery behavior when conditions improve
The key lies in finding the sweet spot where context awareness provides meaningful benefits without creating unsustainable computational or maintenance overhead.
Temporal Consistency in Responses
Temporal consistency introduces a critical trade-off between adaptability and stability in AI systems. As operational environments evolve over time, systems must balance maintaining reliable performance against adapting to new patterns and information.
This tension is particularly evident in financial AI systems. Consider a trading algorithm: it must adapt to changing market conditions while maintaining consistent decision-making logic to prevent erratic behavior. Too much emphasis on consistency could make the system rigid and unable to capture new market patterns, while excessive adaptability might lead to unstable or unpredictable trading strategies.
The core trade-offs include:
Short-term accuracy vs. long-term stability
Quick adaptation vs. reliable performance
Historical pattern preservation vs. new pattern recognition
Computational efficiency vs. temporal analysis depth
Effective evaluation strategies must therefore assess:
Drift patterns in system behavior
Adaptation speed to significant changes
Stability of core decision logic
Impact of temporal adjustments on overall reliability
This delicate balance becomes especially critical in high-stakes domains where both consistency and adaptability directly impact business outcomes.
Ground Truth Ambiguity
Perhaps the most complex trade-off in functional correctness involves scenarios where ground truth itself is uncertain or contested. This creates tension between precision and flexibility in evaluation frameworks.
Medical diagnosis systems exemplify this challenge. When expert opinions diverge on complex cases, the system must balance between making definitive recommendations and acknowledging diagnostic uncertainty. The trade-off lies in maintaining decision confidence while appropriately handling ambiguous cases.
Key tensions include:
Confidence vs. uncertainty communication
Precision vs. inclusivity in valid responses
Processing speed vs. thorough analysis
Resource allocation vs. comprehensive evaluation
Evaluation approaches must therefore:
Incorporate multiple expert perspectives
Weight-varying levels of certainty
Assess reasoning quality beyond outcomes
Balance decisiveness with appropriate caution
Success in handling these trade-offs requires sophisticated evaluation frameworks, including monitoring RAG performance metrics that can accommodate ambiguity while maintaining practical utility for end users.
Five Challenges of Ensuring Functional Correctness in AI (and Their Solutions)
As organizations scale their AI deployments, they face critical challenges in maintaining functional correctness, including common AI agent issues. While these challenges are complex, understanding them—and their solutions—is essential for building reliable AI systems that perform consistently in production environments.
Let's see how traditional approaches have evolved and how modern platforms like Galileo are supercharging these solutions.
Data Quality and Evaluation Precision
Production AI systems often struggle with data quality issues that impact functional correctness and evaluation accuracy. Ensuring the use of high-quality data is crucial in maintaining system performance. Implementing effective ML data intelligence strategies helps in achieving this goal. Even minor data inconsistencies can lead to significant evaluation errors in critical applications like healthcare diagnostics or financial risk assessment, highlighting the high-quality data necessity in AI systems.
Traditional approaches relied heavily on manual validation and basic statistical checks. While these methods helped identify apparent issues, they proved inadequate for complex AI models that require nuanced evaluation across multiple dimensions.
Galileo's Evaluate module tackles this challenge head-on by introducing automated quality assessment and continuous validation pipelines. It uses proprietary metrics, combining research-backed guardrail metrics with custom metrics, to provide comprehensive evaluation coverage and automatically detect data quality issues that could affect model performance. This supports rapid, collaborative experimentation and evaluation of LLM applications.
Production Monitoring at Scale
Production AI systems often struggle with data quality issues that impact functional correctness and evaluation accuracy. Ensuring the use of high-quality data is crucial in maintaining system performance. Implementing effective ML data intelligence strategies helps in achieving this goal. Even minor data inconsistencies can lead to significant evaluation errors in critical applications like healthcare diagnostics or financial risk assessment, highlighting the high-quality data necessity in AI systems.
Traditional approaches relied heavily on manual validation and basic statistical checks. While these methods helped identify apparent issues, they proved inadequate for complex AI models that require nuanced evaluation across multiple dimensions.
Galileo's Evaluate module tackles this challenge head-on by introducing automated quality assessment and continuous validation pipelines. It uses proprietary metrics, combining research-backed guardrail metrics with custom metrics, to provide comprehensive evaluation coverage and automatically detect data quality issues that could affect model performance. This supports rapid, collaborative experimentation and evaluation of LLM applications.
Security and Compliance in Evaluation
Maintaining functional correctness while ensuring compliance presents a significant challenge in highly regulated industries. AI systems must not only perform accurately but also adhere to strict regulatory requirements regarding data privacy, fairness, and transparency.
Traditional compliance approaches often involved separate audit processes and manual compliance checks. While thorough, these methods created operational bottlenecks and struggled to keep pace with rapidly evolving AI systems and regulatory landscapes.
This is where Galileo's Protect module comes in, integrating compliance into the evaluation process itself. It actively monitors outputs for potential compliance violations while maintaining audit trails of model behavior. The module uses Galileo's Guardrail Metrics to ensure its effectiveness in production environments. This integrated approach ensures that functional correctness and regulatory compliance work hand in hand rather than as competing priorities.
Domain-Specific Performance
AI systems deployed across different industries face unique challenges in maintaining functional correctness within their specific domains. A model that performs well in general scenarios may fail to capture crucial domain-specific nuances in fields like legal document analysis or medical diagnostics.
Conventional solutions typically involved creating separate evaluation frameworks for each domain—a resource-intensive approach that often led to inconsistent standards and difficulty in maintaining best practices across different applications.
Galileo's evaluation and optimization framework offers domain-adaptive evaluation capabilities with flexible metrics and evaluation criteria tailored to industry-specific benchmarks. It includes user-defined custom metrics and built-in Guardrail Metrics to assess application quality, such as measuring hallucinations through Context Adherence metrics.
Response Drift Detection
Perhaps the most insidious challenge to functional correctness is response drift—where AI system outputs gradually deviate from expected behavior over time. This drift often occurs so subtly that traditional monitoring methods fail to detect it until significant issues arise.
Historical approaches to drift detection relied on periodic model retraining and manual performance reviews. These methods frequently resulted in delayed responses to emerging issues and unnecessary retraining cycles when the drift wasn't present.
The modern solution? Galileo tackles this challenge through continuous drift monitoring and predictive analytics. The platform uses an advanced embedding-based, non-parametric nearest neighbor algorithm to detect out-of-distribution data, including drifted and out-of-coverage samples.
Moreover, this method capitalizes on the semantic structure in neural network embeddings, offering flexibility and ensuring that functional correctness remains stable over time while optimizing resource allocation for model maintenance.
By tackling these challenges with innovative solutions, Galileo enables enterprises to ensure functional correctness in AI systems, enhancing reliability and promoting scalable advancements.
Optimizing Functional Correctness in AI With Galileo
AI models and systems require meticulous attention to functional correctness, ensuring these systems operate as expected. Understanding functional correctness in AI involves aligning AI decision-making processes with predefined business objectives.
Galileo stands out as a pivotal tool in achieving this alignment in AI systems. The platform supports comprehensive end-to-end observability, enabling enterprises to track every facet of an AI system's operation—from plan generation using LLMs to task execution.
Ready to enhance your AI system's functional correctness? Get started with Galileo GenAI Studio today and experience the difference in your AI evaluation process.
Your AI model passes test cases, yet in production, it consistently makes decisions that leave your team scratching their heads. Sound familiar? This disconnect between expected and actual behavior isn't just frustrating—it's a critical challenge in AI development that stems from gaps in functional correctness.
Whether it's a medical diagnostic tool delivering precise treatment recommendations or a financial system safeguarding fair and compliant loan approvals, functional correctness upholds the integrity of AI applications, boosts user trust, and allows for more advanced innovations.
This guide will explore how leading organizations are mastering functional correctness to build AI systems that not only work in theory but also deliver reliable, consistent results in real-world applications.
We recently explored this topic on our Chain of Thought podcast, where industry experts shared practical insights and real-world implementation strategies.
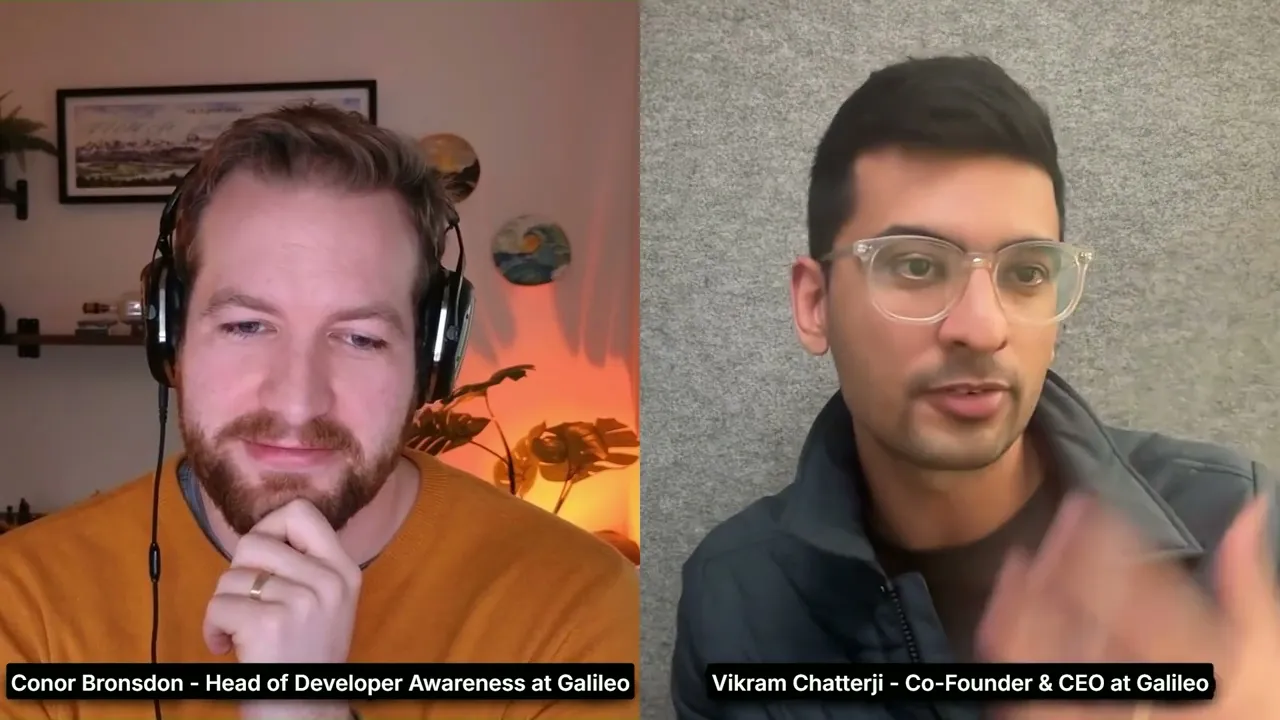
What is Functional Correctness in AI?
At its core, functional correctness represents the fundamental requirement that AI systems behave exactly as specified—not just in controlled environments but also in the complex, unpredictable world of production deployments.
Although this may seem straightforward, ensuring accurate functional correctness in AI systems poses unique challenges that distinguish it from traditional software testing.
Technical Foundations and Evolution from Traditional Metrics
Functional correctness in AI ensures systems perform precisely as intended across all scenarios. Unlike traditional software with precise inputs and outputs, AI operates in dynamic environments, requiring sophisticated validation processes to confirm models meet their requirements and behave predictably.
In safety-critical applications such as autonomous vehicles and healthcare, achieving accuracy benchmarks is only part of the equation—formal verification mechanisms are crucial to ensure that AI performs its designated tasks accurately. While traditional metrics like accuracy, precision, recall, and F1 scores provide valuable insights, they do not fully capture whether an AI system behaves correctly in varying conditions.
This limitation has driven a shift toward comprehensive evaluation frameworks, including advanced methods for evaluating AI agents that blend traditional metrics with robustness, adaptability, and ethical considerations. This evolution ensures systems are not just statistically performant but functionally correct and reliable for enterprise applications.
Real-World Impact on Production AI Systems
The emphasis on functional correctness leads to tangible improvements in production environments. When AI systems function properly, organizations experience enhanced decision-making quality, reduced operational risks, and increased stakeholder trust. Recent research on AI acceptance in healthcare emphasizes this point, demonstrating how reliable AI systems establish crucial trust among medical professionals.
Moreover, these rigorous evaluation frameworks, including real-world AI evaluation, don't just prevent failures—they actively contribute to successful AI deployments by ensuring functionalities align with real-world demands.
For instance, research in autonomous driving demonstrates how explainable AI and functional correctness work together to enhance system reliability and user confidence. As AI becomes increasingly central to business operations, this understanding of functional correctness serves as a crucial safeguard while maximizing the return on AI investments.

Functional Correctness in AI: Complex Evaluation Scenarios and Trade-offs
Ensuring functional correctness in AI systems requires navigating complex trade-offs between competing demands for effective AI risk management. Grasping these trade-offs is essential for developing robust LLM evaluation frameworks that balance various needs while maintaining system reliability.
Let’s see these evaluation trade-offs that go beyond basic testing paradigms.
Non-Deterministic Output Assessment
The challenge of evaluating non-deterministic outputs represents one of the fundamental trade-offs in AI functional correctness. Unlike traditional software, where identical inputs should produce identical outputs, AI systems—particularly LLMs may generate different but equally valid responses to the same prompt.
This variability creates a critical trade-off between consistency and creativity. Strict LLM evaluation metrics and frameworks that require exact output matching could hinder the AI's ability to generate novel, contextually appropriate responses. Conversely, excessive flexibility in evaluation criteria risks accepting incorrect or inappropriate outputs.
Consider a customer service AI: while we want consistent responses to similar queries, enforcing strict response templates could prevent the system from adapting to unique customer needs. The trade-off lies in balancing response variability with reliability.
Therefore, practical evaluation approaches must:
Define acceptable bounds for output variation
Measure semantic similarity rather than exact matches
Assess the consistency of reasoning patterns
Evaluate the impact on end-user experience and business outcomes
This balancing act requires sophisticated metrics that distinguish between beneficial creativity and harmful inconsistency while maintaining precise standards for functional correctness.
Context-Dependent Correctness
Context-dependent correctness presents a fundamental tension between generalization and specialization in AI systems. As operating environments become more complex, we face an increasingly tricky trade-off between building systems that work broadly and those that excel in specific contexts.
Take autonomous vehicles as an example: a vision system might achieve high accuracy in ideal conditions but struggle during harsh weather. The trade-off emerges between optimizing for perfect performance in common scenarios versus maintaining acceptable performance across all conditions. Pursuing both simultaneously often leads to increased computational costs and system complexity.
This creates several critical balancing acts:
Breadth vs. depth of context handling
Processing speed vs. contextual analysis depth
Resource utilization vs. environmental adaptation
Model complexity vs. maintainability
Real-world implementations must carefully navigate these trade-offs. For example, research on autonomous driving systems demonstrates that achieving robust performance across diverse contexts often requires sophisticated sensor fusion and redundant processing paths—methods that directly influence system cost and complexity.
Evaluation frameworks must therefore assess:
Performance stability across context shifts
Degradation patterns in challenging conditions
Resource costs of context adaptation
Recovery behavior when conditions improve
The key lies in finding the sweet spot where context awareness provides meaningful benefits without creating unsustainable computational or maintenance overhead.
Temporal Consistency in Responses
Temporal consistency introduces a critical trade-off between adaptability and stability in AI systems. As operational environments evolve over time, systems must balance maintaining reliable performance against adapting to new patterns and information.
This tension is particularly evident in financial AI systems. Consider a trading algorithm: it must adapt to changing market conditions while maintaining consistent decision-making logic to prevent erratic behavior. Too much emphasis on consistency could make the system rigid and unable to capture new market patterns, while excessive adaptability might lead to unstable or unpredictable trading strategies.
The core trade-offs include:
Short-term accuracy vs. long-term stability
Quick adaptation vs. reliable performance
Historical pattern preservation vs. new pattern recognition
Computational efficiency vs. temporal analysis depth
Effective evaluation strategies must therefore assess:
Drift patterns in system behavior
Adaptation speed to significant changes
Stability of core decision logic
Impact of temporal adjustments on overall reliability
This delicate balance becomes especially critical in high-stakes domains where both consistency and adaptability directly impact business outcomes.
Ground Truth Ambiguity
Perhaps the most complex trade-off in functional correctness involves scenarios where ground truth itself is uncertain or contested. This creates tension between precision and flexibility in evaluation frameworks.
Medical diagnosis systems exemplify this challenge. When expert opinions diverge on complex cases, the system must balance between making definitive recommendations and acknowledging diagnostic uncertainty. The trade-off lies in maintaining decision confidence while appropriately handling ambiguous cases.
Key tensions include:
Confidence vs. uncertainty communication
Precision vs. inclusivity in valid responses
Processing speed vs. thorough analysis
Resource allocation vs. comprehensive evaluation
Evaluation approaches must therefore:
Incorporate multiple expert perspectives
Weight-varying levels of certainty
Assess reasoning quality beyond outcomes
Balance decisiveness with appropriate caution
Success in handling these trade-offs requires sophisticated evaluation frameworks, including monitoring RAG performance metrics that can accommodate ambiguity while maintaining practical utility for end users.
Five Challenges of Ensuring Functional Correctness in AI (and Their Solutions)
As organizations scale their AI deployments, they face critical challenges in maintaining functional correctness, including common AI agent issues. While these challenges are complex, understanding them—and their solutions—is essential for building reliable AI systems that perform consistently in production environments.
Let's see how traditional approaches have evolved and how modern platforms like Galileo are supercharging these solutions.
Data Quality and Evaluation Precision
Production AI systems often struggle with data quality issues that impact functional correctness and evaluation accuracy. Ensuring the use of high-quality data is crucial in maintaining system performance. Implementing effective ML data intelligence strategies helps in achieving this goal. Even minor data inconsistencies can lead to significant evaluation errors in critical applications like healthcare diagnostics or financial risk assessment, highlighting the high-quality data necessity in AI systems.
Traditional approaches relied heavily on manual validation and basic statistical checks. While these methods helped identify apparent issues, they proved inadequate for complex AI models that require nuanced evaluation across multiple dimensions.
Galileo's Evaluate module tackles this challenge head-on by introducing automated quality assessment and continuous validation pipelines. It uses proprietary metrics, combining research-backed guardrail metrics with custom metrics, to provide comprehensive evaluation coverage and automatically detect data quality issues that could affect model performance. This supports rapid, collaborative experimentation and evaluation of LLM applications.
Production Monitoring at Scale
Production AI systems often struggle with data quality issues that impact functional correctness and evaluation accuracy. Ensuring the use of high-quality data is crucial in maintaining system performance. Implementing effective ML data intelligence strategies helps in achieving this goal. Even minor data inconsistencies can lead to significant evaluation errors in critical applications like healthcare diagnostics or financial risk assessment, highlighting the high-quality data necessity in AI systems.
Traditional approaches relied heavily on manual validation and basic statistical checks. While these methods helped identify apparent issues, they proved inadequate for complex AI models that require nuanced evaluation across multiple dimensions.
Galileo's Evaluate module tackles this challenge head-on by introducing automated quality assessment and continuous validation pipelines. It uses proprietary metrics, combining research-backed guardrail metrics with custom metrics, to provide comprehensive evaluation coverage and automatically detect data quality issues that could affect model performance. This supports rapid, collaborative experimentation and evaluation of LLM applications.
Security and Compliance in Evaluation
Maintaining functional correctness while ensuring compliance presents a significant challenge in highly regulated industries. AI systems must not only perform accurately but also adhere to strict regulatory requirements regarding data privacy, fairness, and transparency.
Traditional compliance approaches often involved separate audit processes and manual compliance checks. While thorough, these methods created operational bottlenecks and struggled to keep pace with rapidly evolving AI systems and regulatory landscapes.
This is where Galileo's Protect module comes in, integrating compliance into the evaluation process itself. It actively monitors outputs for potential compliance violations while maintaining audit trails of model behavior. The module uses Galileo's Guardrail Metrics to ensure its effectiveness in production environments. This integrated approach ensures that functional correctness and regulatory compliance work hand in hand rather than as competing priorities.
Domain-Specific Performance
AI systems deployed across different industries face unique challenges in maintaining functional correctness within their specific domains. A model that performs well in general scenarios may fail to capture crucial domain-specific nuances in fields like legal document analysis or medical diagnostics.
Conventional solutions typically involved creating separate evaluation frameworks for each domain—a resource-intensive approach that often led to inconsistent standards and difficulty in maintaining best practices across different applications.
Galileo's evaluation and optimization framework offers domain-adaptive evaluation capabilities with flexible metrics and evaluation criteria tailored to industry-specific benchmarks. It includes user-defined custom metrics and built-in Guardrail Metrics to assess application quality, such as measuring hallucinations through Context Adherence metrics.
Response Drift Detection
Perhaps the most insidious challenge to functional correctness is response drift—where AI system outputs gradually deviate from expected behavior over time. This drift often occurs so subtly that traditional monitoring methods fail to detect it until significant issues arise.
Historical approaches to drift detection relied on periodic model retraining and manual performance reviews. These methods frequently resulted in delayed responses to emerging issues and unnecessary retraining cycles when the drift wasn't present.
The modern solution? Galileo tackles this challenge through continuous drift monitoring and predictive analytics. The platform uses an advanced embedding-based, non-parametric nearest neighbor algorithm to detect out-of-distribution data, including drifted and out-of-coverage samples.
Moreover, this method capitalizes on the semantic structure in neural network embeddings, offering flexibility and ensuring that functional correctness remains stable over time while optimizing resource allocation for model maintenance.
By tackling these challenges with innovative solutions, Galileo enables enterprises to ensure functional correctness in AI systems, enhancing reliability and promoting scalable advancements.
Optimizing Functional Correctness in AI With Galileo
AI models and systems require meticulous attention to functional correctness, ensuring these systems operate as expected. Understanding functional correctness in AI involves aligning AI decision-making processes with predefined business objectives.
Galileo stands out as a pivotal tool in achieving this alignment in AI systems. The platform supports comprehensive end-to-end observability, enabling enterprises to track every facet of an AI system's operation—from plan generation using LLMs to task execution.
Ready to enhance your AI system's functional correctness? Get started with Galileo GenAI Studio today and experience the difference in your AI evaluation process.
Your AI model passes test cases, yet in production, it consistently makes decisions that leave your team scratching their heads. Sound familiar? This disconnect between expected and actual behavior isn't just frustrating—it's a critical challenge in AI development that stems from gaps in functional correctness.
Whether it's a medical diagnostic tool delivering precise treatment recommendations or a financial system safeguarding fair and compliant loan approvals, functional correctness upholds the integrity of AI applications, boosts user trust, and allows for more advanced innovations.
This guide will explore how leading organizations are mastering functional correctness to build AI systems that not only work in theory but also deliver reliable, consistent results in real-world applications.
We recently explored this topic on our Chain of Thought podcast, where industry experts shared practical insights and real-world implementation strategies.
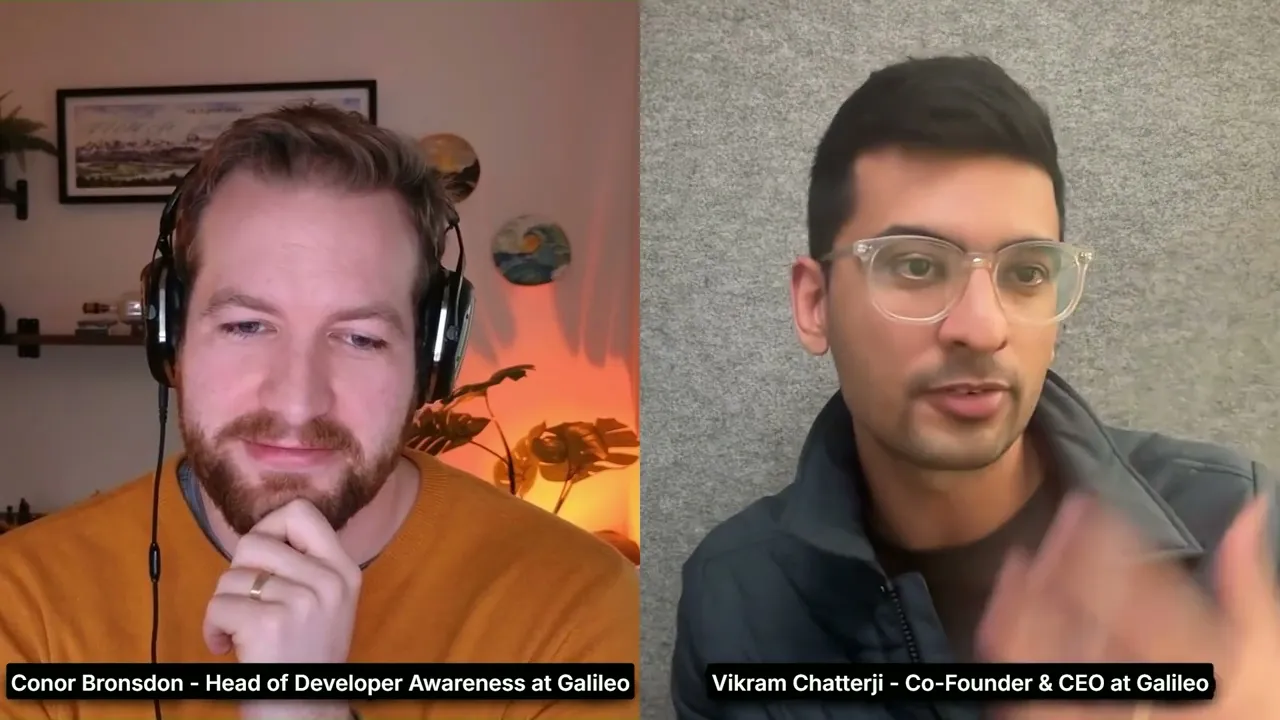
What is Functional Correctness in AI?
At its core, functional correctness represents the fundamental requirement that AI systems behave exactly as specified—not just in controlled environments but also in the complex, unpredictable world of production deployments.
Although this may seem straightforward, ensuring accurate functional correctness in AI systems poses unique challenges that distinguish it from traditional software testing.
Technical Foundations and Evolution from Traditional Metrics
Functional correctness in AI ensures systems perform precisely as intended across all scenarios. Unlike traditional software with precise inputs and outputs, AI operates in dynamic environments, requiring sophisticated validation processes to confirm models meet their requirements and behave predictably.
In safety-critical applications such as autonomous vehicles and healthcare, achieving accuracy benchmarks is only part of the equation—formal verification mechanisms are crucial to ensure that AI performs its designated tasks accurately. While traditional metrics like accuracy, precision, recall, and F1 scores provide valuable insights, they do not fully capture whether an AI system behaves correctly in varying conditions.
This limitation has driven a shift toward comprehensive evaluation frameworks, including advanced methods for evaluating AI agents that blend traditional metrics with robustness, adaptability, and ethical considerations. This evolution ensures systems are not just statistically performant but functionally correct and reliable for enterprise applications.
Real-World Impact on Production AI Systems
The emphasis on functional correctness leads to tangible improvements in production environments. When AI systems function properly, organizations experience enhanced decision-making quality, reduced operational risks, and increased stakeholder trust. Recent research on AI acceptance in healthcare emphasizes this point, demonstrating how reliable AI systems establish crucial trust among medical professionals.
Moreover, these rigorous evaluation frameworks, including real-world AI evaluation, don't just prevent failures—they actively contribute to successful AI deployments by ensuring functionalities align with real-world demands.
For instance, research in autonomous driving demonstrates how explainable AI and functional correctness work together to enhance system reliability and user confidence. As AI becomes increasingly central to business operations, this understanding of functional correctness serves as a crucial safeguard while maximizing the return on AI investments.

Functional Correctness in AI: Complex Evaluation Scenarios and Trade-offs
Ensuring functional correctness in AI systems requires navigating complex trade-offs between competing demands for effective AI risk management. Grasping these trade-offs is essential for developing robust LLM evaluation frameworks that balance various needs while maintaining system reliability.
Let’s see these evaluation trade-offs that go beyond basic testing paradigms.
Non-Deterministic Output Assessment
The challenge of evaluating non-deterministic outputs represents one of the fundamental trade-offs in AI functional correctness. Unlike traditional software, where identical inputs should produce identical outputs, AI systems—particularly LLMs may generate different but equally valid responses to the same prompt.
This variability creates a critical trade-off between consistency and creativity. Strict LLM evaluation metrics and frameworks that require exact output matching could hinder the AI's ability to generate novel, contextually appropriate responses. Conversely, excessive flexibility in evaluation criteria risks accepting incorrect or inappropriate outputs.
Consider a customer service AI: while we want consistent responses to similar queries, enforcing strict response templates could prevent the system from adapting to unique customer needs. The trade-off lies in balancing response variability with reliability.
Therefore, practical evaluation approaches must:
Define acceptable bounds for output variation
Measure semantic similarity rather than exact matches
Assess the consistency of reasoning patterns
Evaluate the impact on end-user experience and business outcomes
This balancing act requires sophisticated metrics that distinguish between beneficial creativity and harmful inconsistency while maintaining precise standards for functional correctness.
Context-Dependent Correctness
Context-dependent correctness presents a fundamental tension between generalization and specialization in AI systems. As operating environments become more complex, we face an increasingly tricky trade-off between building systems that work broadly and those that excel in specific contexts.
Take autonomous vehicles as an example: a vision system might achieve high accuracy in ideal conditions but struggle during harsh weather. The trade-off emerges between optimizing for perfect performance in common scenarios versus maintaining acceptable performance across all conditions. Pursuing both simultaneously often leads to increased computational costs and system complexity.
This creates several critical balancing acts:
Breadth vs. depth of context handling
Processing speed vs. contextual analysis depth
Resource utilization vs. environmental adaptation
Model complexity vs. maintainability
Real-world implementations must carefully navigate these trade-offs. For example, research on autonomous driving systems demonstrates that achieving robust performance across diverse contexts often requires sophisticated sensor fusion and redundant processing paths—methods that directly influence system cost and complexity.
Evaluation frameworks must therefore assess:
Performance stability across context shifts
Degradation patterns in challenging conditions
Resource costs of context adaptation
Recovery behavior when conditions improve
The key lies in finding the sweet spot where context awareness provides meaningful benefits without creating unsustainable computational or maintenance overhead.
Temporal Consistency in Responses
Temporal consistency introduces a critical trade-off between adaptability and stability in AI systems. As operational environments evolve over time, systems must balance maintaining reliable performance against adapting to new patterns and information.
This tension is particularly evident in financial AI systems. Consider a trading algorithm: it must adapt to changing market conditions while maintaining consistent decision-making logic to prevent erratic behavior. Too much emphasis on consistency could make the system rigid and unable to capture new market patterns, while excessive adaptability might lead to unstable or unpredictable trading strategies.
The core trade-offs include:
Short-term accuracy vs. long-term stability
Quick adaptation vs. reliable performance
Historical pattern preservation vs. new pattern recognition
Computational efficiency vs. temporal analysis depth
Effective evaluation strategies must therefore assess:
Drift patterns in system behavior
Adaptation speed to significant changes
Stability of core decision logic
Impact of temporal adjustments on overall reliability
This delicate balance becomes especially critical in high-stakes domains where both consistency and adaptability directly impact business outcomes.
Ground Truth Ambiguity
Perhaps the most complex trade-off in functional correctness involves scenarios where ground truth itself is uncertain or contested. This creates tension between precision and flexibility in evaluation frameworks.
Medical diagnosis systems exemplify this challenge. When expert opinions diverge on complex cases, the system must balance between making definitive recommendations and acknowledging diagnostic uncertainty. The trade-off lies in maintaining decision confidence while appropriately handling ambiguous cases.
Key tensions include:
Confidence vs. uncertainty communication
Precision vs. inclusivity in valid responses
Processing speed vs. thorough analysis
Resource allocation vs. comprehensive evaluation
Evaluation approaches must therefore:
Incorporate multiple expert perspectives
Weight-varying levels of certainty
Assess reasoning quality beyond outcomes
Balance decisiveness with appropriate caution
Success in handling these trade-offs requires sophisticated evaluation frameworks, including monitoring RAG performance metrics that can accommodate ambiguity while maintaining practical utility for end users.
Five Challenges of Ensuring Functional Correctness in AI (and Their Solutions)
As organizations scale their AI deployments, they face critical challenges in maintaining functional correctness, including common AI agent issues. While these challenges are complex, understanding them—and their solutions—is essential for building reliable AI systems that perform consistently in production environments.
Let's see how traditional approaches have evolved and how modern platforms like Galileo are supercharging these solutions.
Data Quality and Evaluation Precision
Production AI systems often struggle with data quality issues that impact functional correctness and evaluation accuracy. Ensuring the use of high-quality data is crucial in maintaining system performance. Implementing effective ML data intelligence strategies helps in achieving this goal. Even minor data inconsistencies can lead to significant evaluation errors in critical applications like healthcare diagnostics or financial risk assessment, highlighting the high-quality data necessity in AI systems.
Traditional approaches relied heavily on manual validation and basic statistical checks. While these methods helped identify apparent issues, they proved inadequate for complex AI models that require nuanced evaluation across multiple dimensions.
Galileo's Evaluate module tackles this challenge head-on by introducing automated quality assessment and continuous validation pipelines. It uses proprietary metrics, combining research-backed guardrail metrics with custom metrics, to provide comprehensive evaluation coverage and automatically detect data quality issues that could affect model performance. This supports rapid, collaborative experimentation and evaluation of LLM applications.
Production Monitoring at Scale
Production AI systems often struggle with data quality issues that impact functional correctness and evaluation accuracy. Ensuring the use of high-quality data is crucial in maintaining system performance. Implementing effective ML data intelligence strategies helps in achieving this goal. Even minor data inconsistencies can lead to significant evaluation errors in critical applications like healthcare diagnostics or financial risk assessment, highlighting the high-quality data necessity in AI systems.
Traditional approaches relied heavily on manual validation and basic statistical checks. While these methods helped identify apparent issues, they proved inadequate for complex AI models that require nuanced evaluation across multiple dimensions.
Galileo's Evaluate module tackles this challenge head-on by introducing automated quality assessment and continuous validation pipelines. It uses proprietary metrics, combining research-backed guardrail metrics with custom metrics, to provide comprehensive evaluation coverage and automatically detect data quality issues that could affect model performance. This supports rapid, collaborative experimentation and evaluation of LLM applications.
Security and Compliance in Evaluation
Maintaining functional correctness while ensuring compliance presents a significant challenge in highly regulated industries. AI systems must not only perform accurately but also adhere to strict regulatory requirements regarding data privacy, fairness, and transparency.
Traditional compliance approaches often involved separate audit processes and manual compliance checks. While thorough, these methods created operational bottlenecks and struggled to keep pace with rapidly evolving AI systems and regulatory landscapes.
This is where Galileo's Protect module comes in, integrating compliance into the evaluation process itself. It actively monitors outputs for potential compliance violations while maintaining audit trails of model behavior. The module uses Galileo's Guardrail Metrics to ensure its effectiveness in production environments. This integrated approach ensures that functional correctness and regulatory compliance work hand in hand rather than as competing priorities.
Domain-Specific Performance
AI systems deployed across different industries face unique challenges in maintaining functional correctness within their specific domains. A model that performs well in general scenarios may fail to capture crucial domain-specific nuances in fields like legal document analysis or medical diagnostics.
Conventional solutions typically involved creating separate evaluation frameworks for each domain—a resource-intensive approach that often led to inconsistent standards and difficulty in maintaining best practices across different applications.
Galileo's evaluation and optimization framework offers domain-adaptive evaluation capabilities with flexible metrics and evaluation criteria tailored to industry-specific benchmarks. It includes user-defined custom metrics and built-in Guardrail Metrics to assess application quality, such as measuring hallucinations through Context Adherence metrics.
Response Drift Detection
Perhaps the most insidious challenge to functional correctness is response drift—where AI system outputs gradually deviate from expected behavior over time. This drift often occurs so subtly that traditional monitoring methods fail to detect it until significant issues arise.
Historical approaches to drift detection relied on periodic model retraining and manual performance reviews. These methods frequently resulted in delayed responses to emerging issues and unnecessary retraining cycles when the drift wasn't present.
The modern solution? Galileo tackles this challenge through continuous drift monitoring and predictive analytics. The platform uses an advanced embedding-based, non-parametric nearest neighbor algorithm to detect out-of-distribution data, including drifted and out-of-coverage samples.
Moreover, this method capitalizes on the semantic structure in neural network embeddings, offering flexibility and ensuring that functional correctness remains stable over time while optimizing resource allocation for model maintenance.
By tackling these challenges with innovative solutions, Galileo enables enterprises to ensure functional correctness in AI systems, enhancing reliability and promoting scalable advancements.
Optimizing Functional Correctness in AI With Galileo
AI models and systems require meticulous attention to functional correctness, ensuring these systems operate as expected. Understanding functional correctness in AI involves aligning AI decision-making processes with predefined business objectives.
Galileo stands out as a pivotal tool in achieving this alignment in AI systems. The platform supports comprehensive end-to-end observability, enabling enterprises to track every facet of an AI system's operation—from plan generation using LLMs to task execution.
Ready to enhance your AI system's functional correctness? Get started with Galileo GenAI Studio today and experience the difference in your AI evaluation process.
Conor Bronsdon
Conor Bronsdon
Conor Bronsdon
Conor Bronsdon