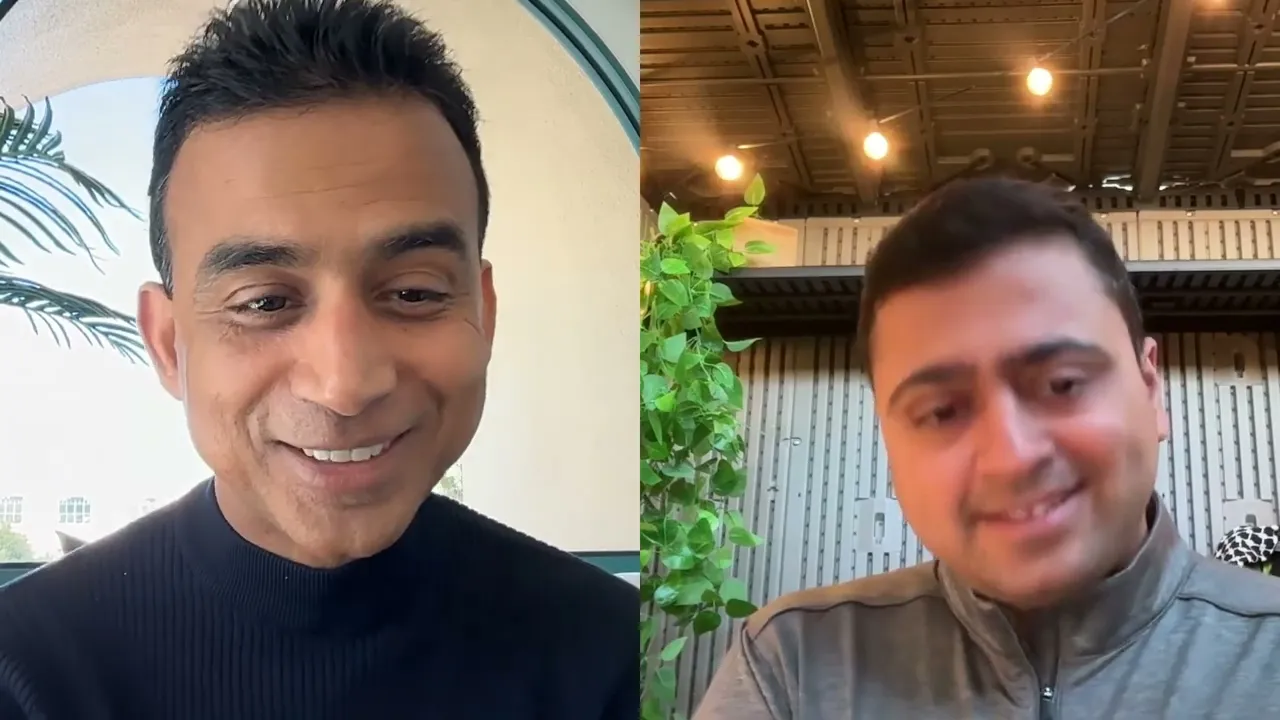
Apr 10, 2025
A Guide to Measuring Communication Efficiency in Multi-Agent AI Systems


Conor Bronsdon
Head of Developer Awareness
Conor Bronsdon
Head of Developer Awareness


Ever wondered what happens when AI agents try to work together? Multi-agent AI systems are transforming business operations by distributing complex tasks among specialized AI agents that collaborate toward common goals. The success of these systems depends critically on something fundamentally human: effective communication between agents.
Inefficient communication between AI agents translates directly to business costs. Users experience frustration with delayed responses, computing resources are wasted, and output quality deteriorates.
As organizations increasingly deploy these systems across functions from customer service to supply chain management, optimizing agent communication becomes a significant competitive advantage.
This article explores practical approaches to measuring communication efficiency in multi-agent AI systems, providing you with actionable insights to maximize your AI investments while avoiding the pitfalls of poorly designed interactions.
We recently explored this topic on our Chain of Thought podcast, where industry experts shared practical insights and real-world implementation strategies.
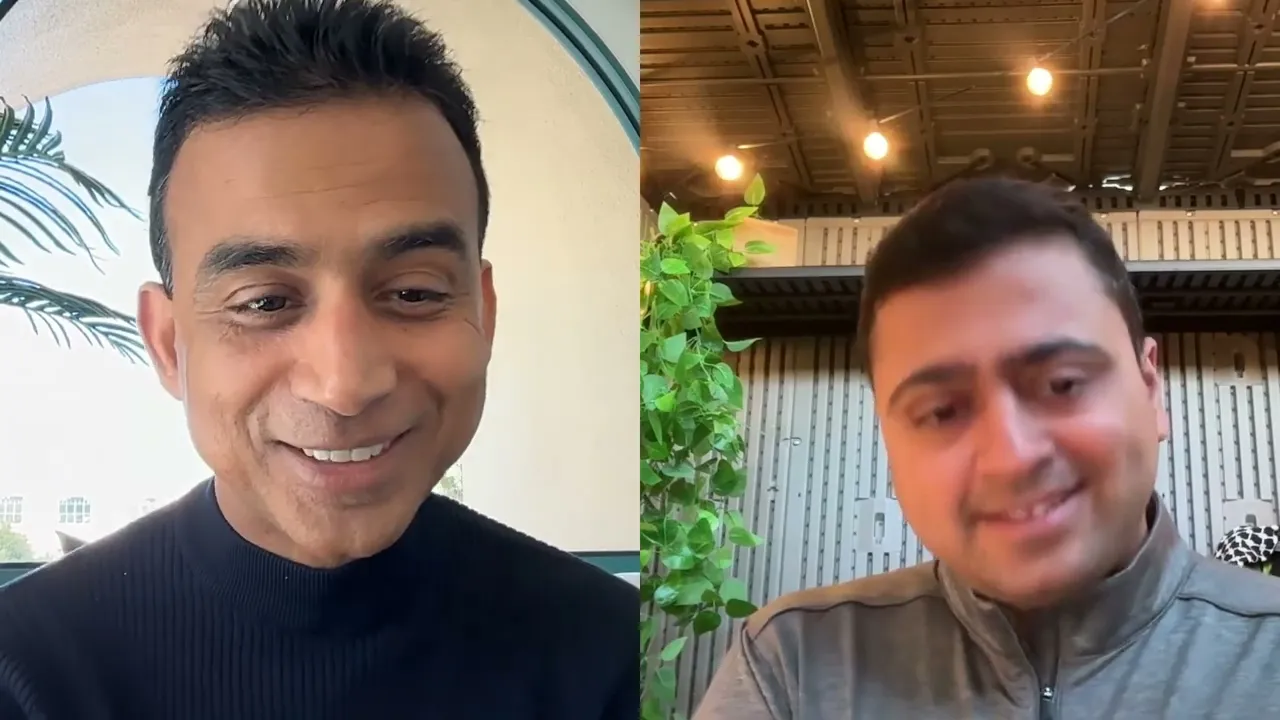
Foundational Communication Patterns in Multi-Agent AI Systems
Before diving into measurement techniques, let’s understand the foundational communication architectures that define how multiple AI agents interact.
Communication patterns dictate how information flows between agents, directly impacting system performance, scalability, and reliability. These patterns fall into several key dimensions: direct and indirect communication, centralized and decentralized architectures, and synchronous versus asynchronous messaging.

Direct and Indirect
Direct communication occurs when agents explicitly address and send messages to specific recipient agents, creating point-to-point connections. Direct communication between AI agents enables faster responses and clearer information exchange. This approach excels when agents require real-time coordination for time-sensitive tasks.
Conversely, indirect communication occurs through shared environments or intermediaries. This method reduces network congestion by eliminating direct connections, making it ideal for large-scale systems where direct messaging would overwhelm the network.
These architectural decisions fundamentally shape your system design. Direct methods require robust addressing systems to properly route messages, while indirect approaches need well-designed environmental interfaces.
Research demonstrates that indirect communication works better at scale with thousands of agents, though it makes coordination more complex and may introduce security challenges in multi-agent systems.
For scenarios requiring rapid coordination among a limited number of agents, direct communication proves most effective. When building systems requiring environmental persistence or loose coupling, indirect methods typically deliver better results.
Centralized and Decentralized
Your architectural choice impacts more than message routing. Centralized systems excel at enforcing global policies but face scalability challenges, while decentralized systems adapt more readily to network changes but require sophisticated coordination mechanisms
Centralized systems route all communication through a control hub. This provides a single source of truth and streamlined decision-making. The controller maintains complete visibility, facilitating implementation of sophisticated coordination strategies.
On the other hand, decentralized systems distribute communication responsibilities across the network without a central authority. This eliminates single points of failure and offers superior scalability as your agent population expands.
Financial trading systems frequently employ decentralized communication to minimize latency, with agents making autonomous decisions based on local data. However, maintaining global consistency becomes challenging without central oversight. Many contemporary systems implement hybrid models—decentralized execution with centralized monitoring.
Synchronous and Asynchronous
Synchronous communication requires the sending agent to wait for a response before proceeding. This ensures consistency and simplifies implementation, but wastes resources when agents remain idle awaiting replies.
Conversely, asynchronous communication allows agents to continue processing after sending messages without waiting. This enables higher concurrency and improved resource utilization, particularly in high-volume systems. Agents can manage multiple tasks simultaneously rather than blocking on communication.
Implementing asynchronous systems requires thoughtful handling of message queues, callbacks, and error recovery procedures. Managing timeouts and failure scenarios introduces additional complexity.
Align your communication style with your specific application requirements. Systems demanding strict transactional guarantees often benefit from synchronous approaches, while applications prioritizing throughput and responsiveness typically perform better with asynchronous patterns.
Comprehensive Strategies for Measuring Communication Efficiency in Multi-Agent AI
Let's explore practical approaches to measure communication efficiency in multi-agent systems.
Implement Quantitative Communication Volume Metrics
Begin with foundational measurements: quantify data flow between agents. Track message-to-task ratio to determine how many communications your system requires to complete specific operations. This straightforward metric reveals whether your agents communicate efficiently or excessively.
Also, establish lightweight logging around message transmission points—capture timestamps, size, sender, receiver, and purpose. Collect this data asynchronously to a dedicated analytics store to ensure monitoring doesn't impact performance.
In addition, monitor bandwidth utilization to identify potential system constraints. Calculate this as the percentage of available network capacity consumed by agent communications. High-performing financial trading systems typically maintain bandwidth utilization below 40% to accommodate traffic spikes.
Determine communication overhead percentage by dividing protocol bytes, metadata, and coordination information by total bytes transferred. Well-optimized systems maintain this below 15%, ensuring most bandwidth carries valuable information.
Monitor message complexity to identify unnecessarily verbose communications. Track average message size and analyze semantic density to confirm messages contain meaningful, non-redundant information that contributes directly to task completion.
Galileo supports quantitative communication metrics through its real-time monitoring capabilities, allowing teams to track message volume, size, and frequency across agent interactions.
Galileo automatically logs communication patterns and calculates key metrics such as message-to-task ratios and bandwidth utilization, providing insights into potential optimization opportunities without adding monitoring overhead to your production systems.
Apply Latency and Temporal Efficiency Measurements
Latency is paramount for time-critical applications. Measure the end-to-end delay from message creation through processing completion at the receiving agent. This captures the true experience rather than simply network transmission time.
Avoid the pitfalls of averages, which can mask serious issues. Track 95th and 99th percentile latencies to understand worst-case scenarios affecting user experience. Examine latency patterns over time to identify cyclical bottlenecks. Many systems experience predictable load patterns, causing periodic delays. Correlating these patterns with system events helps identify root causes for proactive remediation.
Furthermore, utilize cumulative distribution functions (CDFs) to visualize latency distribution. This reveals whether delays affect a small percentage of communications or represent system-wide issues requiring architectural adjustments.
For prioritization, establish context-aware latency targets based on priority. Critical messages may require sub-50ms delivery, while background synchronization can tolerate higher latencies. Measuring against these differentiated targets provides more actionable insights than universal metrics.
Galileo provides comprehensive latency tracking and temporal analysis, with built-in visualization tools for examining the distribution of communication delays. With dashboards presenting metrics and patterns, teams can identify bottlenecks affecting system performance and establish appropriate latency benchmarks based on message priority and context.
Use Information Quality and Relevance Metrics
Beyond quantity and speed, assess quality and AI fluency. Apply embedding-based techniques to evaluate how effectively each message contributes to the current task. This identifies when agents exchange unnecessary information.
Assess information entropy to quantify communication predictability. Low entropy indicates agents exchange repetitive or redundant messages. Efficient systems maximize information content while minimizing message size.
Evaluate the proportion of actionable information to coordination overhead using signal-to-noise ratio analysis. For transmission, determine semantic compression ratios by comparing message size to unique information content. This identifies opportunities for more efficient encoding. Advanced systems achieve 3:1 or higher compression without sacrificing critical meaning.
Correlate communication patterns with outcomes through task achievement analysis. Examining which communication strategies lead to successful task completion helps evolve messaging protocols toward effectiveness rather than focusing exclusively on speed or volume.
Galileo offers advanced information quality analysis capabilities, automatically evaluating message relevance and identifying redundant communications between agents. Galileo connects metrics with task completion outcomes to help teams develop more efficient messaging strategies based on empirical performance data.
Deploy Advanced Dimensional Analysis Techniques
Dimensional analysis reveals inefficiencies in your communication information space. Apply Principal Component Analysis (PCA) to message embeddings to identify redundant dimensions. This frequently reveals that 60–70% of dimensions carry minimal unique information.
Similarly, calculate the effective information dimension using intrinsic dimensionality estimation. This determines how many dimensions are actually required to represent the exchanged information. Many systems use 3–4× more dimensions than necessary, creating avoidable overhead.
Visualize message clusters with t-SNE or UMAP to identify semantic patterns and redundancies. These techniques highlight when similar messages are repeatedly exchanged or when agents fail to share context effectively.
For timely measurements, monitor dimensional compression ratios over time to measure progress. Train autoencoders on agent messages to measure communication compressibility. This helps determine the minimum dimensional representation needed for effective information exchange while maintaining message semantics.
For continuous analysis, apply Singular Value Decomposition (SVD) to communication matrices to identify dominant patterns. This reveals which aspects carry the most significant information, enabling targeted optimization of important components rather than treating all dimensions equally.
Galileo helps identify redundant information dimensions and provides visualization capabilities to highlight semantic clustering patterns, assisting teams to implement more efficient encoding schemes and reduce communication overhead.
Industry-Specific Measurement Frameworks
Financial services require specialized metrics focused on both speed and compliance. Implement sequence-aware latency tracking for trading systems where message order impacts outcomes. Monitor regulatory compliance through message persistence, audit trail completeness, and information disclosure metrics, all of which are critical components of AI risk management.
For trading platforms, measure information advantage decay—how quickly information value diminishes over time. High-frequency trading systems require sub-millisecond communication to maintain information advantages, while longer-term strategies can accommodate higher latencies without performance degradation.
Healthcare systems must balance privacy with efficiency. Apply differential privacy when measuring communication patterns to safeguard sensitive patient information. Monitor HIPAA-compliant message sanitization overhead to understand the performance impact of maintaining privacy.
For clinical decision support networks, track diagnostic information efficiency—how effectively communications contribute to accurate and timely diagnoses. Leading healthcare AI systems maintain diagnostic precision while reducing irrelevant information exchange through optimized protocols.
Manufacturing environments require real-time control system metrics. Analyze jitter to measure communication timing consistency, critical for synchronized operations. Track closed-loop response time across multi-agent systems controlling physical equipment to ensure safe operation.
Galileo provides industry-specific measurement templates and compliance tools designed for sectors with specialized communication requirements. Teams can access and implement domain-specific metrics and compliance monitoring, allowing teams to evaluate communication efficiency within their regulatory and operational constraints while maintaining appropriate security protocols.
Optimize Communication in Your Multi-Agent Systems with Galileo
Effective measurement of communication efficiency in multi-agent AI encompasses factors from latency and throughput to message complexity and coordination. Galileo's platform supports your communication measurement within these systems directly:
Real-Time Communication Monitoring: Galileo provides detailed analytics on message passing between agents, helping you identify bottlenecks and inefficiencies impacting system performance.
Bandwidth Optimization: Galileo analyzes data transfer patterns to help reduce unnecessary communication overhead while preserving effective agent coordination.
Scalability Analysis: Galileo measures how communication overhead increases as agent count grows, supporting the development of systems that scale efficiently.
Custom Metrics Dashboard: Monitor the metrics most relevant to your specific use case with customizable dashboards providing at-a-glance insights for data-driven optimization.
Protocol Optimization: Galileo’s intelligent analysis tools recommend the most efficient communication protocols for your specific multi-agent architecture, enhancing overall system performance.
Explore Galileo today and discover how our platform helps you build more efficient, responsive, and scalable multi-agent AI systems that communicate effectively regardless of your implementation complexity.
Ever wondered what happens when AI agents try to work together? Multi-agent AI systems are transforming business operations by distributing complex tasks among specialized AI agents that collaborate toward common goals. The success of these systems depends critically on something fundamentally human: effective communication between agents.
Inefficient communication between AI agents translates directly to business costs. Users experience frustration with delayed responses, computing resources are wasted, and output quality deteriorates.
As organizations increasingly deploy these systems across functions from customer service to supply chain management, optimizing agent communication becomes a significant competitive advantage.
This article explores practical approaches to measuring communication efficiency in multi-agent AI systems, providing you with actionable insights to maximize your AI investments while avoiding the pitfalls of poorly designed interactions.
We recently explored this topic on our Chain of Thought podcast, where industry experts shared practical insights and real-world implementation strategies.
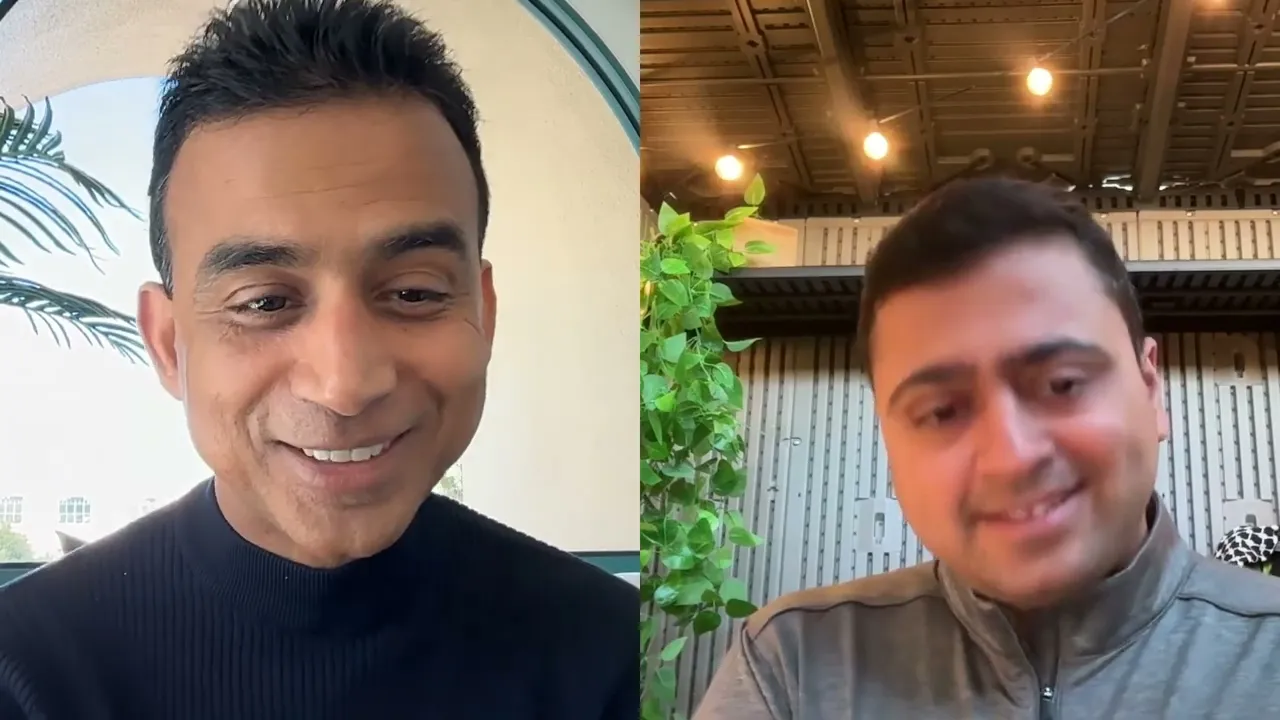
Foundational Communication Patterns in Multi-Agent AI Systems
Before diving into measurement techniques, let’s understand the foundational communication architectures that define how multiple AI agents interact.
Communication patterns dictate how information flows between agents, directly impacting system performance, scalability, and reliability. These patterns fall into several key dimensions: direct and indirect communication, centralized and decentralized architectures, and synchronous versus asynchronous messaging.

Direct and Indirect
Direct communication occurs when agents explicitly address and send messages to specific recipient agents, creating point-to-point connections. Direct communication between AI agents enables faster responses and clearer information exchange. This approach excels when agents require real-time coordination for time-sensitive tasks.
Conversely, indirect communication occurs through shared environments or intermediaries. This method reduces network congestion by eliminating direct connections, making it ideal for large-scale systems where direct messaging would overwhelm the network.
These architectural decisions fundamentally shape your system design. Direct methods require robust addressing systems to properly route messages, while indirect approaches need well-designed environmental interfaces.
Research demonstrates that indirect communication works better at scale with thousands of agents, though it makes coordination more complex and may introduce security challenges in multi-agent systems.
For scenarios requiring rapid coordination among a limited number of agents, direct communication proves most effective. When building systems requiring environmental persistence or loose coupling, indirect methods typically deliver better results.
Centralized and Decentralized
Your architectural choice impacts more than message routing. Centralized systems excel at enforcing global policies but face scalability challenges, while decentralized systems adapt more readily to network changes but require sophisticated coordination mechanisms
Centralized systems route all communication through a control hub. This provides a single source of truth and streamlined decision-making. The controller maintains complete visibility, facilitating implementation of sophisticated coordination strategies.
On the other hand, decentralized systems distribute communication responsibilities across the network without a central authority. This eliminates single points of failure and offers superior scalability as your agent population expands.
Financial trading systems frequently employ decentralized communication to minimize latency, with agents making autonomous decisions based on local data. However, maintaining global consistency becomes challenging without central oversight. Many contemporary systems implement hybrid models—decentralized execution with centralized monitoring.
Synchronous and Asynchronous
Synchronous communication requires the sending agent to wait for a response before proceeding. This ensures consistency and simplifies implementation, but wastes resources when agents remain idle awaiting replies.
Conversely, asynchronous communication allows agents to continue processing after sending messages without waiting. This enables higher concurrency and improved resource utilization, particularly in high-volume systems. Agents can manage multiple tasks simultaneously rather than blocking on communication.
Implementing asynchronous systems requires thoughtful handling of message queues, callbacks, and error recovery procedures. Managing timeouts and failure scenarios introduces additional complexity.
Align your communication style with your specific application requirements. Systems demanding strict transactional guarantees often benefit from synchronous approaches, while applications prioritizing throughput and responsiveness typically perform better with asynchronous patterns.
Comprehensive Strategies for Measuring Communication Efficiency in Multi-Agent AI
Let's explore practical approaches to measure communication efficiency in multi-agent systems.
Implement Quantitative Communication Volume Metrics
Begin with foundational measurements: quantify data flow between agents. Track message-to-task ratio to determine how many communications your system requires to complete specific operations. This straightforward metric reveals whether your agents communicate efficiently or excessively.
Also, establish lightweight logging around message transmission points—capture timestamps, size, sender, receiver, and purpose. Collect this data asynchronously to a dedicated analytics store to ensure monitoring doesn't impact performance.
In addition, monitor bandwidth utilization to identify potential system constraints. Calculate this as the percentage of available network capacity consumed by agent communications. High-performing financial trading systems typically maintain bandwidth utilization below 40% to accommodate traffic spikes.
Determine communication overhead percentage by dividing protocol bytes, metadata, and coordination information by total bytes transferred. Well-optimized systems maintain this below 15%, ensuring most bandwidth carries valuable information.
Monitor message complexity to identify unnecessarily verbose communications. Track average message size and analyze semantic density to confirm messages contain meaningful, non-redundant information that contributes directly to task completion.
Galileo supports quantitative communication metrics through its real-time monitoring capabilities, allowing teams to track message volume, size, and frequency across agent interactions.
Galileo automatically logs communication patterns and calculates key metrics such as message-to-task ratios and bandwidth utilization, providing insights into potential optimization opportunities without adding monitoring overhead to your production systems.
Apply Latency and Temporal Efficiency Measurements
Latency is paramount for time-critical applications. Measure the end-to-end delay from message creation through processing completion at the receiving agent. This captures the true experience rather than simply network transmission time.
Avoid the pitfalls of averages, which can mask serious issues. Track 95th and 99th percentile latencies to understand worst-case scenarios affecting user experience. Examine latency patterns over time to identify cyclical bottlenecks. Many systems experience predictable load patterns, causing periodic delays. Correlating these patterns with system events helps identify root causes for proactive remediation.
Furthermore, utilize cumulative distribution functions (CDFs) to visualize latency distribution. This reveals whether delays affect a small percentage of communications or represent system-wide issues requiring architectural adjustments.
For prioritization, establish context-aware latency targets based on priority. Critical messages may require sub-50ms delivery, while background synchronization can tolerate higher latencies. Measuring against these differentiated targets provides more actionable insights than universal metrics.
Galileo provides comprehensive latency tracking and temporal analysis, with built-in visualization tools for examining the distribution of communication delays. With dashboards presenting metrics and patterns, teams can identify bottlenecks affecting system performance and establish appropriate latency benchmarks based on message priority and context.
Use Information Quality and Relevance Metrics
Beyond quantity and speed, assess quality and AI fluency. Apply embedding-based techniques to evaluate how effectively each message contributes to the current task. This identifies when agents exchange unnecessary information.
Assess information entropy to quantify communication predictability. Low entropy indicates agents exchange repetitive or redundant messages. Efficient systems maximize information content while minimizing message size.
Evaluate the proportion of actionable information to coordination overhead using signal-to-noise ratio analysis. For transmission, determine semantic compression ratios by comparing message size to unique information content. This identifies opportunities for more efficient encoding. Advanced systems achieve 3:1 or higher compression without sacrificing critical meaning.
Correlate communication patterns with outcomes through task achievement analysis. Examining which communication strategies lead to successful task completion helps evolve messaging protocols toward effectiveness rather than focusing exclusively on speed or volume.
Galileo offers advanced information quality analysis capabilities, automatically evaluating message relevance and identifying redundant communications between agents. Galileo connects metrics with task completion outcomes to help teams develop more efficient messaging strategies based on empirical performance data.
Deploy Advanced Dimensional Analysis Techniques
Dimensional analysis reveals inefficiencies in your communication information space. Apply Principal Component Analysis (PCA) to message embeddings to identify redundant dimensions. This frequently reveals that 60–70% of dimensions carry minimal unique information.
Similarly, calculate the effective information dimension using intrinsic dimensionality estimation. This determines how many dimensions are actually required to represent the exchanged information. Many systems use 3–4× more dimensions than necessary, creating avoidable overhead.
Visualize message clusters with t-SNE or UMAP to identify semantic patterns and redundancies. These techniques highlight when similar messages are repeatedly exchanged or when agents fail to share context effectively.
For timely measurements, monitor dimensional compression ratios over time to measure progress. Train autoencoders on agent messages to measure communication compressibility. This helps determine the minimum dimensional representation needed for effective information exchange while maintaining message semantics.
For continuous analysis, apply Singular Value Decomposition (SVD) to communication matrices to identify dominant patterns. This reveals which aspects carry the most significant information, enabling targeted optimization of important components rather than treating all dimensions equally.
Galileo helps identify redundant information dimensions and provides visualization capabilities to highlight semantic clustering patterns, assisting teams to implement more efficient encoding schemes and reduce communication overhead.
Industry-Specific Measurement Frameworks
Financial services require specialized metrics focused on both speed and compliance. Implement sequence-aware latency tracking for trading systems where message order impacts outcomes. Monitor regulatory compliance through message persistence, audit trail completeness, and information disclosure metrics, all of which are critical components of AI risk management.
For trading platforms, measure information advantage decay—how quickly information value diminishes over time. High-frequency trading systems require sub-millisecond communication to maintain information advantages, while longer-term strategies can accommodate higher latencies without performance degradation.
Healthcare systems must balance privacy with efficiency. Apply differential privacy when measuring communication patterns to safeguard sensitive patient information. Monitor HIPAA-compliant message sanitization overhead to understand the performance impact of maintaining privacy.
For clinical decision support networks, track diagnostic information efficiency—how effectively communications contribute to accurate and timely diagnoses. Leading healthcare AI systems maintain diagnostic precision while reducing irrelevant information exchange through optimized protocols.
Manufacturing environments require real-time control system metrics. Analyze jitter to measure communication timing consistency, critical for synchronized operations. Track closed-loop response time across multi-agent systems controlling physical equipment to ensure safe operation.
Galileo provides industry-specific measurement templates and compliance tools designed for sectors with specialized communication requirements. Teams can access and implement domain-specific metrics and compliance monitoring, allowing teams to evaluate communication efficiency within their regulatory and operational constraints while maintaining appropriate security protocols.
Optimize Communication in Your Multi-Agent Systems with Galileo
Effective measurement of communication efficiency in multi-agent AI encompasses factors from latency and throughput to message complexity and coordination. Galileo's platform supports your communication measurement within these systems directly:
Real-Time Communication Monitoring: Galileo provides detailed analytics on message passing between agents, helping you identify bottlenecks and inefficiencies impacting system performance.
Bandwidth Optimization: Galileo analyzes data transfer patterns to help reduce unnecessary communication overhead while preserving effective agent coordination.
Scalability Analysis: Galileo measures how communication overhead increases as agent count grows, supporting the development of systems that scale efficiently.
Custom Metrics Dashboard: Monitor the metrics most relevant to your specific use case with customizable dashboards providing at-a-glance insights for data-driven optimization.
Protocol Optimization: Galileo’s intelligent analysis tools recommend the most efficient communication protocols for your specific multi-agent architecture, enhancing overall system performance.
Explore Galileo today and discover how our platform helps you build more efficient, responsive, and scalable multi-agent AI systems that communicate effectively regardless of your implementation complexity.
Ever wondered what happens when AI agents try to work together? Multi-agent AI systems are transforming business operations by distributing complex tasks among specialized AI agents that collaborate toward common goals. The success of these systems depends critically on something fundamentally human: effective communication between agents.
Inefficient communication between AI agents translates directly to business costs. Users experience frustration with delayed responses, computing resources are wasted, and output quality deteriorates.
As organizations increasingly deploy these systems across functions from customer service to supply chain management, optimizing agent communication becomes a significant competitive advantage.
This article explores practical approaches to measuring communication efficiency in multi-agent AI systems, providing you with actionable insights to maximize your AI investments while avoiding the pitfalls of poorly designed interactions.
We recently explored this topic on our Chain of Thought podcast, where industry experts shared practical insights and real-world implementation strategies.
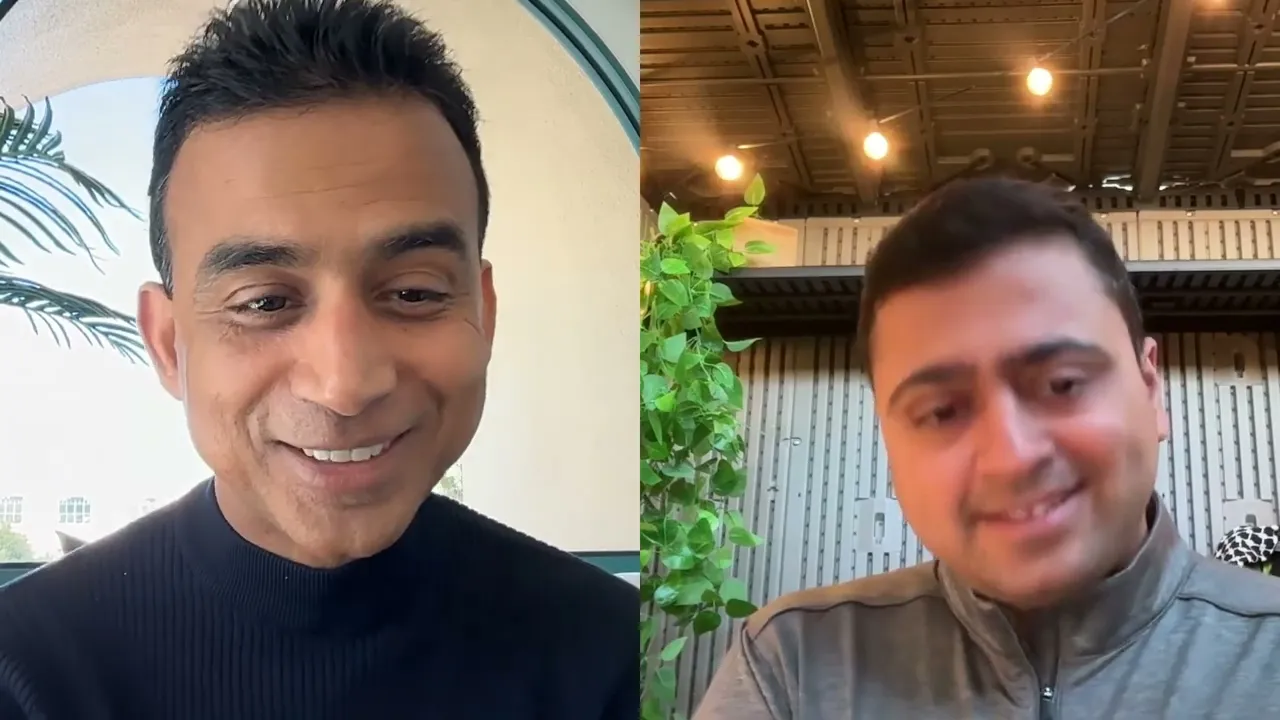
Foundational Communication Patterns in Multi-Agent AI Systems
Before diving into measurement techniques, let’s understand the foundational communication architectures that define how multiple AI agents interact.
Communication patterns dictate how information flows between agents, directly impacting system performance, scalability, and reliability. These patterns fall into several key dimensions: direct and indirect communication, centralized and decentralized architectures, and synchronous versus asynchronous messaging.

Direct and Indirect
Direct communication occurs when agents explicitly address and send messages to specific recipient agents, creating point-to-point connections. Direct communication between AI agents enables faster responses and clearer information exchange. This approach excels when agents require real-time coordination for time-sensitive tasks.
Conversely, indirect communication occurs through shared environments or intermediaries. This method reduces network congestion by eliminating direct connections, making it ideal for large-scale systems where direct messaging would overwhelm the network.
These architectural decisions fundamentally shape your system design. Direct methods require robust addressing systems to properly route messages, while indirect approaches need well-designed environmental interfaces.
Research demonstrates that indirect communication works better at scale with thousands of agents, though it makes coordination more complex and may introduce security challenges in multi-agent systems.
For scenarios requiring rapid coordination among a limited number of agents, direct communication proves most effective. When building systems requiring environmental persistence or loose coupling, indirect methods typically deliver better results.
Centralized and Decentralized
Your architectural choice impacts more than message routing. Centralized systems excel at enforcing global policies but face scalability challenges, while decentralized systems adapt more readily to network changes but require sophisticated coordination mechanisms
Centralized systems route all communication through a control hub. This provides a single source of truth and streamlined decision-making. The controller maintains complete visibility, facilitating implementation of sophisticated coordination strategies.
On the other hand, decentralized systems distribute communication responsibilities across the network without a central authority. This eliminates single points of failure and offers superior scalability as your agent population expands.
Financial trading systems frequently employ decentralized communication to minimize latency, with agents making autonomous decisions based on local data. However, maintaining global consistency becomes challenging without central oversight. Many contemporary systems implement hybrid models—decentralized execution with centralized monitoring.
Synchronous and Asynchronous
Synchronous communication requires the sending agent to wait for a response before proceeding. This ensures consistency and simplifies implementation, but wastes resources when agents remain idle awaiting replies.
Conversely, asynchronous communication allows agents to continue processing after sending messages without waiting. This enables higher concurrency and improved resource utilization, particularly in high-volume systems. Agents can manage multiple tasks simultaneously rather than blocking on communication.
Implementing asynchronous systems requires thoughtful handling of message queues, callbacks, and error recovery procedures. Managing timeouts and failure scenarios introduces additional complexity.
Align your communication style with your specific application requirements. Systems demanding strict transactional guarantees often benefit from synchronous approaches, while applications prioritizing throughput and responsiveness typically perform better with asynchronous patterns.
Comprehensive Strategies for Measuring Communication Efficiency in Multi-Agent AI
Let's explore practical approaches to measure communication efficiency in multi-agent systems.
Implement Quantitative Communication Volume Metrics
Begin with foundational measurements: quantify data flow between agents. Track message-to-task ratio to determine how many communications your system requires to complete specific operations. This straightforward metric reveals whether your agents communicate efficiently or excessively.
Also, establish lightweight logging around message transmission points—capture timestamps, size, sender, receiver, and purpose. Collect this data asynchronously to a dedicated analytics store to ensure monitoring doesn't impact performance.
In addition, monitor bandwidth utilization to identify potential system constraints. Calculate this as the percentage of available network capacity consumed by agent communications. High-performing financial trading systems typically maintain bandwidth utilization below 40% to accommodate traffic spikes.
Determine communication overhead percentage by dividing protocol bytes, metadata, and coordination information by total bytes transferred. Well-optimized systems maintain this below 15%, ensuring most bandwidth carries valuable information.
Monitor message complexity to identify unnecessarily verbose communications. Track average message size and analyze semantic density to confirm messages contain meaningful, non-redundant information that contributes directly to task completion.
Galileo supports quantitative communication metrics through its real-time monitoring capabilities, allowing teams to track message volume, size, and frequency across agent interactions.
Galileo automatically logs communication patterns and calculates key metrics such as message-to-task ratios and bandwidth utilization, providing insights into potential optimization opportunities without adding monitoring overhead to your production systems.
Apply Latency and Temporal Efficiency Measurements
Latency is paramount for time-critical applications. Measure the end-to-end delay from message creation through processing completion at the receiving agent. This captures the true experience rather than simply network transmission time.
Avoid the pitfalls of averages, which can mask serious issues. Track 95th and 99th percentile latencies to understand worst-case scenarios affecting user experience. Examine latency patterns over time to identify cyclical bottlenecks. Many systems experience predictable load patterns, causing periodic delays. Correlating these patterns with system events helps identify root causes for proactive remediation.
Furthermore, utilize cumulative distribution functions (CDFs) to visualize latency distribution. This reveals whether delays affect a small percentage of communications or represent system-wide issues requiring architectural adjustments.
For prioritization, establish context-aware latency targets based on priority. Critical messages may require sub-50ms delivery, while background synchronization can tolerate higher latencies. Measuring against these differentiated targets provides more actionable insights than universal metrics.
Galileo provides comprehensive latency tracking and temporal analysis, with built-in visualization tools for examining the distribution of communication delays. With dashboards presenting metrics and patterns, teams can identify bottlenecks affecting system performance and establish appropriate latency benchmarks based on message priority and context.
Use Information Quality and Relevance Metrics
Beyond quantity and speed, assess quality and AI fluency. Apply embedding-based techniques to evaluate how effectively each message contributes to the current task. This identifies when agents exchange unnecessary information.
Assess information entropy to quantify communication predictability. Low entropy indicates agents exchange repetitive or redundant messages. Efficient systems maximize information content while minimizing message size.
Evaluate the proportion of actionable information to coordination overhead using signal-to-noise ratio analysis. For transmission, determine semantic compression ratios by comparing message size to unique information content. This identifies opportunities for more efficient encoding. Advanced systems achieve 3:1 or higher compression without sacrificing critical meaning.
Correlate communication patterns with outcomes through task achievement analysis. Examining which communication strategies lead to successful task completion helps evolve messaging protocols toward effectiveness rather than focusing exclusively on speed or volume.
Galileo offers advanced information quality analysis capabilities, automatically evaluating message relevance and identifying redundant communications between agents. Galileo connects metrics with task completion outcomes to help teams develop more efficient messaging strategies based on empirical performance data.
Deploy Advanced Dimensional Analysis Techniques
Dimensional analysis reveals inefficiencies in your communication information space. Apply Principal Component Analysis (PCA) to message embeddings to identify redundant dimensions. This frequently reveals that 60–70% of dimensions carry minimal unique information.
Similarly, calculate the effective information dimension using intrinsic dimensionality estimation. This determines how many dimensions are actually required to represent the exchanged information. Many systems use 3–4× more dimensions than necessary, creating avoidable overhead.
Visualize message clusters with t-SNE or UMAP to identify semantic patterns and redundancies. These techniques highlight when similar messages are repeatedly exchanged or when agents fail to share context effectively.
For timely measurements, monitor dimensional compression ratios over time to measure progress. Train autoencoders on agent messages to measure communication compressibility. This helps determine the minimum dimensional representation needed for effective information exchange while maintaining message semantics.
For continuous analysis, apply Singular Value Decomposition (SVD) to communication matrices to identify dominant patterns. This reveals which aspects carry the most significant information, enabling targeted optimization of important components rather than treating all dimensions equally.
Galileo helps identify redundant information dimensions and provides visualization capabilities to highlight semantic clustering patterns, assisting teams to implement more efficient encoding schemes and reduce communication overhead.
Industry-Specific Measurement Frameworks
Financial services require specialized metrics focused on both speed and compliance. Implement sequence-aware latency tracking for trading systems where message order impacts outcomes. Monitor regulatory compliance through message persistence, audit trail completeness, and information disclosure metrics, all of which are critical components of AI risk management.
For trading platforms, measure information advantage decay—how quickly information value diminishes over time. High-frequency trading systems require sub-millisecond communication to maintain information advantages, while longer-term strategies can accommodate higher latencies without performance degradation.
Healthcare systems must balance privacy with efficiency. Apply differential privacy when measuring communication patterns to safeguard sensitive patient information. Monitor HIPAA-compliant message sanitization overhead to understand the performance impact of maintaining privacy.
For clinical decision support networks, track diagnostic information efficiency—how effectively communications contribute to accurate and timely diagnoses. Leading healthcare AI systems maintain diagnostic precision while reducing irrelevant information exchange through optimized protocols.
Manufacturing environments require real-time control system metrics. Analyze jitter to measure communication timing consistency, critical for synchronized operations. Track closed-loop response time across multi-agent systems controlling physical equipment to ensure safe operation.
Galileo provides industry-specific measurement templates and compliance tools designed for sectors with specialized communication requirements. Teams can access and implement domain-specific metrics and compliance monitoring, allowing teams to evaluate communication efficiency within their regulatory and operational constraints while maintaining appropriate security protocols.
Optimize Communication in Your Multi-Agent Systems with Galileo
Effective measurement of communication efficiency in multi-agent AI encompasses factors from latency and throughput to message complexity and coordination. Galileo's platform supports your communication measurement within these systems directly:
Real-Time Communication Monitoring: Galileo provides detailed analytics on message passing between agents, helping you identify bottlenecks and inefficiencies impacting system performance.
Bandwidth Optimization: Galileo analyzes data transfer patterns to help reduce unnecessary communication overhead while preserving effective agent coordination.
Scalability Analysis: Galileo measures how communication overhead increases as agent count grows, supporting the development of systems that scale efficiently.
Custom Metrics Dashboard: Monitor the metrics most relevant to your specific use case with customizable dashboards providing at-a-glance insights for data-driven optimization.
Protocol Optimization: Galileo’s intelligent analysis tools recommend the most efficient communication protocols for your specific multi-agent architecture, enhancing overall system performance.
Explore Galileo today and discover how our platform helps you build more efficient, responsive, and scalable multi-agent AI systems that communicate effectively regardless of your implementation complexity.
Ever wondered what happens when AI agents try to work together? Multi-agent AI systems are transforming business operations by distributing complex tasks among specialized AI agents that collaborate toward common goals. The success of these systems depends critically on something fundamentally human: effective communication between agents.
Inefficient communication between AI agents translates directly to business costs. Users experience frustration with delayed responses, computing resources are wasted, and output quality deteriorates.
As organizations increasingly deploy these systems across functions from customer service to supply chain management, optimizing agent communication becomes a significant competitive advantage.
This article explores practical approaches to measuring communication efficiency in multi-agent AI systems, providing you with actionable insights to maximize your AI investments while avoiding the pitfalls of poorly designed interactions.
We recently explored this topic on our Chain of Thought podcast, where industry experts shared practical insights and real-world implementation strategies.
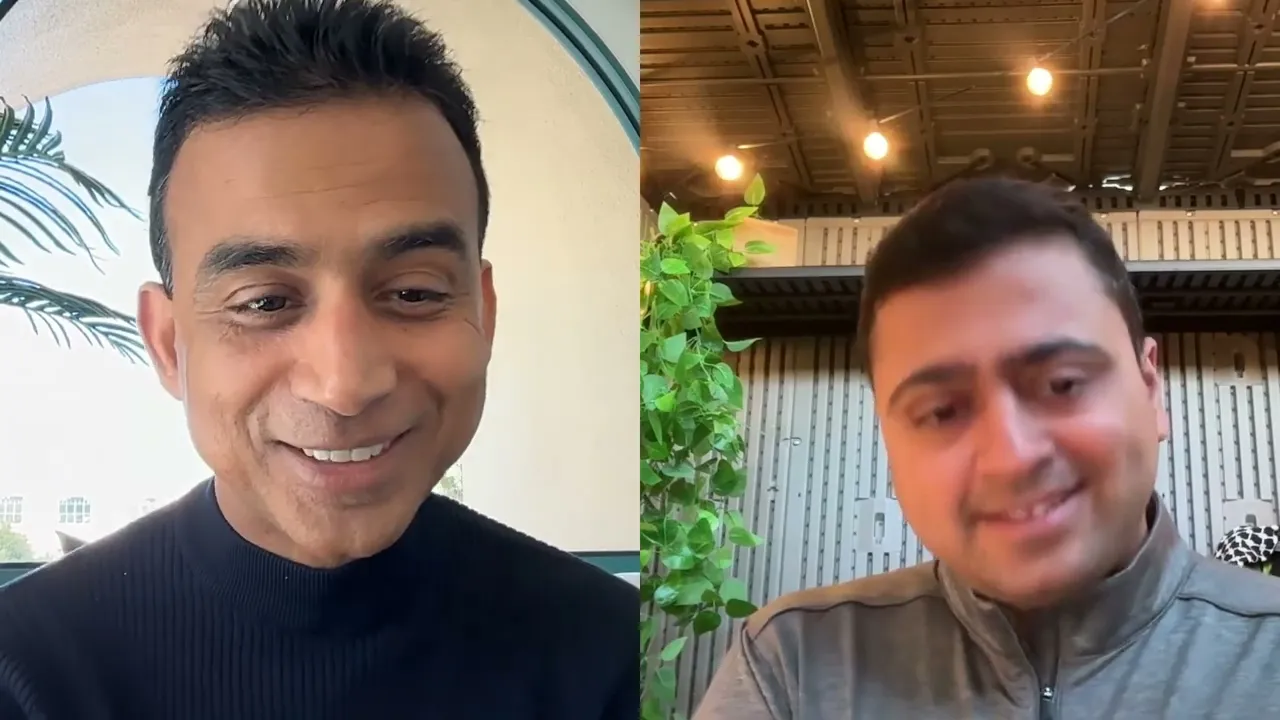
Foundational Communication Patterns in Multi-Agent AI Systems
Before diving into measurement techniques, let’s understand the foundational communication architectures that define how multiple AI agents interact.
Communication patterns dictate how information flows between agents, directly impacting system performance, scalability, and reliability. These patterns fall into several key dimensions: direct and indirect communication, centralized and decentralized architectures, and synchronous versus asynchronous messaging.

Direct and Indirect
Direct communication occurs when agents explicitly address and send messages to specific recipient agents, creating point-to-point connections. Direct communication between AI agents enables faster responses and clearer information exchange. This approach excels when agents require real-time coordination for time-sensitive tasks.
Conversely, indirect communication occurs through shared environments or intermediaries. This method reduces network congestion by eliminating direct connections, making it ideal for large-scale systems where direct messaging would overwhelm the network.
These architectural decisions fundamentally shape your system design. Direct methods require robust addressing systems to properly route messages, while indirect approaches need well-designed environmental interfaces.
Research demonstrates that indirect communication works better at scale with thousands of agents, though it makes coordination more complex and may introduce security challenges in multi-agent systems.
For scenarios requiring rapid coordination among a limited number of agents, direct communication proves most effective. When building systems requiring environmental persistence or loose coupling, indirect methods typically deliver better results.
Centralized and Decentralized
Your architectural choice impacts more than message routing. Centralized systems excel at enforcing global policies but face scalability challenges, while decentralized systems adapt more readily to network changes but require sophisticated coordination mechanisms
Centralized systems route all communication through a control hub. This provides a single source of truth and streamlined decision-making. The controller maintains complete visibility, facilitating implementation of sophisticated coordination strategies.
On the other hand, decentralized systems distribute communication responsibilities across the network without a central authority. This eliminates single points of failure and offers superior scalability as your agent population expands.
Financial trading systems frequently employ decentralized communication to minimize latency, with agents making autonomous decisions based on local data. However, maintaining global consistency becomes challenging without central oversight. Many contemporary systems implement hybrid models—decentralized execution with centralized monitoring.
Synchronous and Asynchronous
Synchronous communication requires the sending agent to wait for a response before proceeding. This ensures consistency and simplifies implementation, but wastes resources when agents remain idle awaiting replies.
Conversely, asynchronous communication allows agents to continue processing after sending messages without waiting. This enables higher concurrency and improved resource utilization, particularly in high-volume systems. Agents can manage multiple tasks simultaneously rather than blocking on communication.
Implementing asynchronous systems requires thoughtful handling of message queues, callbacks, and error recovery procedures. Managing timeouts and failure scenarios introduces additional complexity.
Align your communication style with your specific application requirements. Systems demanding strict transactional guarantees often benefit from synchronous approaches, while applications prioritizing throughput and responsiveness typically perform better with asynchronous patterns.
Comprehensive Strategies for Measuring Communication Efficiency in Multi-Agent AI
Let's explore practical approaches to measure communication efficiency in multi-agent systems.
Implement Quantitative Communication Volume Metrics
Begin with foundational measurements: quantify data flow between agents. Track message-to-task ratio to determine how many communications your system requires to complete specific operations. This straightforward metric reveals whether your agents communicate efficiently or excessively.
Also, establish lightweight logging around message transmission points—capture timestamps, size, sender, receiver, and purpose. Collect this data asynchronously to a dedicated analytics store to ensure monitoring doesn't impact performance.
In addition, monitor bandwidth utilization to identify potential system constraints. Calculate this as the percentage of available network capacity consumed by agent communications. High-performing financial trading systems typically maintain bandwidth utilization below 40% to accommodate traffic spikes.
Determine communication overhead percentage by dividing protocol bytes, metadata, and coordination information by total bytes transferred. Well-optimized systems maintain this below 15%, ensuring most bandwidth carries valuable information.
Monitor message complexity to identify unnecessarily verbose communications. Track average message size and analyze semantic density to confirm messages contain meaningful, non-redundant information that contributes directly to task completion.
Galileo supports quantitative communication metrics through its real-time monitoring capabilities, allowing teams to track message volume, size, and frequency across agent interactions.
Galileo automatically logs communication patterns and calculates key metrics such as message-to-task ratios and bandwidth utilization, providing insights into potential optimization opportunities without adding monitoring overhead to your production systems.
Apply Latency and Temporal Efficiency Measurements
Latency is paramount for time-critical applications. Measure the end-to-end delay from message creation through processing completion at the receiving agent. This captures the true experience rather than simply network transmission time.
Avoid the pitfalls of averages, which can mask serious issues. Track 95th and 99th percentile latencies to understand worst-case scenarios affecting user experience. Examine latency patterns over time to identify cyclical bottlenecks. Many systems experience predictable load patterns, causing periodic delays. Correlating these patterns with system events helps identify root causes for proactive remediation.
Furthermore, utilize cumulative distribution functions (CDFs) to visualize latency distribution. This reveals whether delays affect a small percentage of communications or represent system-wide issues requiring architectural adjustments.
For prioritization, establish context-aware latency targets based on priority. Critical messages may require sub-50ms delivery, while background synchronization can tolerate higher latencies. Measuring against these differentiated targets provides more actionable insights than universal metrics.
Galileo provides comprehensive latency tracking and temporal analysis, with built-in visualization tools for examining the distribution of communication delays. With dashboards presenting metrics and patterns, teams can identify bottlenecks affecting system performance and establish appropriate latency benchmarks based on message priority and context.
Use Information Quality and Relevance Metrics
Beyond quantity and speed, assess quality and AI fluency. Apply embedding-based techniques to evaluate how effectively each message contributes to the current task. This identifies when agents exchange unnecessary information.
Assess information entropy to quantify communication predictability. Low entropy indicates agents exchange repetitive or redundant messages. Efficient systems maximize information content while minimizing message size.
Evaluate the proportion of actionable information to coordination overhead using signal-to-noise ratio analysis. For transmission, determine semantic compression ratios by comparing message size to unique information content. This identifies opportunities for more efficient encoding. Advanced systems achieve 3:1 or higher compression without sacrificing critical meaning.
Correlate communication patterns with outcomes through task achievement analysis. Examining which communication strategies lead to successful task completion helps evolve messaging protocols toward effectiveness rather than focusing exclusively on speed or volume.
Galileo offers advanced information quality analysis capabilities, automatically evaluating message relevance and identifying redundant communications between agents. Galileo connects metrics with task completion outcomes to help teams develop more efficient messaging strategies based on empirical performance data.
Deploy Advanced Dimensional Analysis Techniques
Dimensional analysis reveals inefficiencies in your communication information space. Apply Principal Component Analysis (PCA) to message embeddings to identify redundant dimensions. This frequently reveals that 60–70% of dimensions carry minimal unique information.
Similarly, calculate the effective information dimension using intrinsic dimensionality estimation. This determines how many dimensions are actually required to represent the exchanged information. Many systems use 3–4× more dimensions than necessary, creating avoidable overhead.
Visualize message clusters with t-SNE or UMAP to identify semantic patterns and redundancies. These techniques highlight when similar messages are repeatedly exchanged or when agents fail to share context effectively.
For timely measurements, monitor dimensional compression ratios over time to measure progress. Train autoencoders on agent messages to measure communication compressibility. This helps determine the minimum dimensional representation needed for effective information exchange while maintaining message semantics.
For continuous analysis, apply Singular Value Decomposition (SVD) to communication matrices to identify dominant patterns. This reveals which aspects carry the most significant information, enabling targeted optimization of important components rather than treating all dimensions equally.
Galileo helps identify redundant information dimensions and provides visualization capabilities to highlight semantic clustering patterns, assisting teams to implement more efficient encoding schemes and reduce communication overhead.
Industry-Specific Measurement Frameworks
Financial services require specialized metrics focused on both speed and compliance. Implement sequence-aware latency tracking for trading systems where message order impacts outcomes. Monitor regulatory compliance through message persistence, audit trail completeness, and information disclosure metrics, all of which are critical components of AI risk management.
For trading platforms, measure information advantage decay—how quickly information value diminishes over time. High-frequency trading systems require sub-millisecond communication to maintain information advantages, while longer-term strategies can accommodate higher latencies without performance degradation.
Healthcare systems must balance privacy with efficiency. Apply differential privacy when measuring communication patterns to safeguard sensitive patient information. Monitor HIPAA-compliant message sanitization overhead to understand the performance impact of maintaining privacy.
For clinical decision support networks, track diagnostic information efficiency—how effectively communications contribute to accurate and timely diagnoses. Leading healthcare AI systems maintain diagnostic precision while reducing irrelevant information exchange through optimized protocols.
Manufacturing environments require real-time control system metrics. Analyze jitter to measure communication timing consistency, critical for synchronized operations. Track closed-loop response time across multi-agent systems controlling physical equipment to ensure safe operation.
Galileo provides industry-specific measurement templates and compliance tools designed for sectors with specialized communication requirements. Teams can access and implement domain-specific metrics and compliance monitoring, allowing teams to evaluate communication efficiency within their regulatory and operational constraints while maintaining appropriate security protocols.
Optimize Communication in Your Multi-Agent Systems with Galileo
Effective measurement of communication efficiency in multi-agent AI encompasses factors from latency and throughput to message complexity and coordination. Galileo's platform supports your communication measurement within these systems directly:
Real-Time Communication Monitoring: Galileo provides detailed analytics on message passing between agents, helping you identify bottlenecks and inefficiencies impacting system performance.
Bandwidth Optimization: Galileo analyzes data transfer patterns to help reduce unnecessary communication overhead while preserving effective agent coordination.
Scalability Analysis: Galileo measures how communication overhead increases as agent count grows, supporting the development of systems that scale efficiently.
Custom Metrics Dashboard: Monitor the metrics most relevant to your specific use case with customizable dashboards providing at-a-glance insights for data-driven optimization.
Protocol Optimization: Galileo’s intelligent analysis tools recommend the most efficient communication protocols for your specific multi-agent architecture, enhancing overall system performance.
Explore Galileo today and discover how our platform helps you build more efficient, responsive, and scalable multi-agent AI systems that communicate effectively regardless of your implementation complexity.
Conor Bronsdon
Conor Bronsdon
Conor Bronsdon
Conor Bronsdon