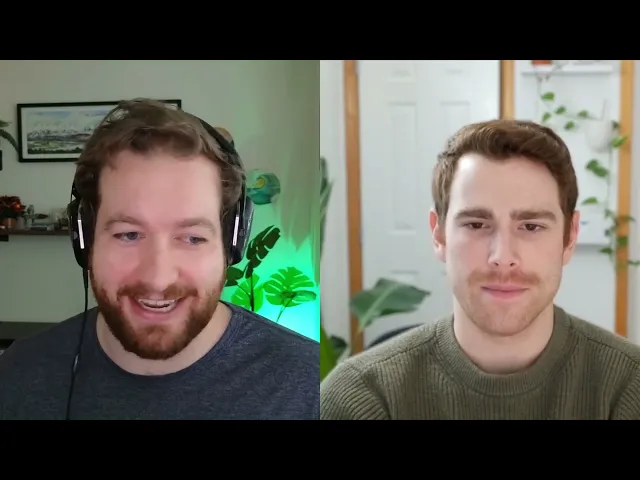
Feb 11, 2025
Unlocking the Power of Multimodal AI and Insights from Google’s Gemini Models


Conor Bronsdon
Head of Developer Awareness
Conor Bronsdon
Head of Developer Awareness


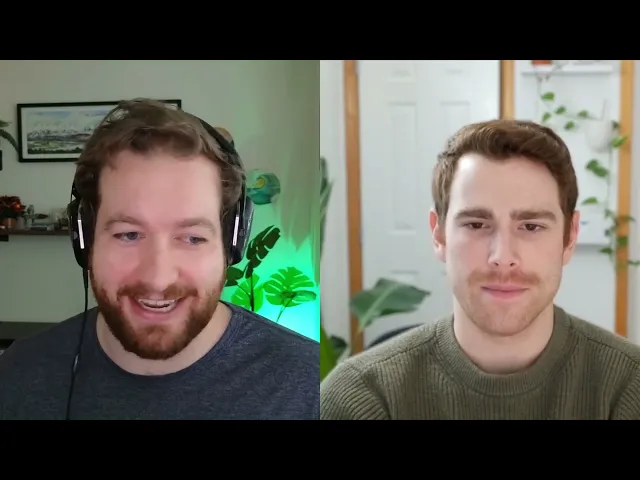
Multimodal AI combines text, images, audio, and video, giving AI multiple senses just like humans use sight, sound, and touch. In a Chain of Thought episode, Conor Bronsdon, Head of Developer Awareness at Galileo, explored the power of Multimodal AI with Logan Kilpatrick, a Senior Product Manager at Google DeepMind.
Google's Gemini was designed from scratch to handle multiple data types simultaneously, making machine intelligence more practical for real-world problems. This native multimodal design represents a significant shift in AI development.
The conversation explored how multimodal AI capabilities make AI smarter and more versatile, whether it's a virtual assistant that both sees and hears or healthcare systems analyzing medical texts alongside images.
Understanding Multimodal AI Capabilities
Multimodal AI functions like human intelligence by integrating multiple senses simultaneously. These systems combine different data forms to create a complete picture rather than fragmented insights, enabling better pattern recognition and problem-solving. To achieve this, teams employ evaluation techniques for multimodal AI to ensure optimal performance.
As Kilpatrick explained, "Think of multimodal AI as an intelligence that can see, listen, and read simultaneously." Just as we use multiple senses to understand our world, these AI systems combine different data forms for better decision-making.
Benefits of Integrating Multiple Modalities
Multimodal AI excels where single-mode systems fail by shifting seamlessly between different data formats. This approach reduces ambiguity in AI responses and provides contextual richness. When a system can simultaneously analyze a medical image and the patient's history, it delivers more nuanced, accurate insights than either modality could provide alone.
"It's about bringing intuitive and simplified user experiences into AI-enabled environments," Kilpatrick notes. By analyzing correlations across modalities, these systems can identify subtle patterns invisible within a single data type, thus enhancing performance and security.
However, teams must be cautious of phenomena like hallucinations in multimodal models, which can impact the reliability of AI outputs. In security applications, suspicious behavior might only become apparent when analyzing both visual footage and audio cues together.
Practical Applications of Multimodal AI
Multimodal AI is already transforming industries. Kilpatrick discusses how multimodal AI models excel in agentic AI systems that act on users' behalf, by processing multiple data types simultaneously. Their performance stands out on industry leaderboards for both capability and cost-efficiency.
In education, these systems create engaging environments where visuals, sounds, and narratives work together to boost understanding and retention.
Medical diagnostics has emerged as a particularly promising field. Radiologists using multimodal AI can simultaneously analyze X-ray images, patient medical histories, lab results, and related research papers, significantly improving diagnostic accuracy and treatment planning.
Customer service has been revolutionized by systems that understand both written complaints and uploaded images of defective products. This comprehensive understanding allows for more precise troubleshooting and faster resolution times.
E-commerce platforms now employ multimodal AI to enhance product discovery by understanding both text queries and visual preferences, creating more personalized shopping experiences that match functional needs and aesthetic preferences.
While these applications showcase the potential of multimodal AI, teams must navigate the challenges in building Multimodal LLMs to fully realize these benefits.
Democratizing AI with Multimodal Technology
Multimodal AI is breaking down barriers to entry. Traditional AI development required deep technical knowledge, but multimodal AI tools like Google's Gemini are changing that equation.
Reducing Dependence on Specialized ML Expertise
Building AI solutions previously demanded extensive machine learning expertise. Today's multimodal AI platforms simplify this process significantly, enabling developers to focus on solving business problems rather than wrestling with complex ML infrastructure.
"Gemini models enable developers and democratize access," Kilpatrick emphasizes. Google's integration of these tools into their platforms means small businesses can innovate without massive investments, fostering a broader range of AI applications.
The intuitive API design allows a restaurant owner with basic programming knowledge to build a system that analyzes food photos and reviews to identify trending preferences without hiring an ML specialist.
Pre-trained multimodal capabilities eliminate the need for extensive dataset collection and model training. Previously, creating even simple vision-language applications required separate datasets for each modality, specialized training workflows, and complex model fusion techniques—barriers that have largely disappeared with Gemini's unified approach.
Domain experts without AI backgrounds can now directly leverage their knowledge through these accessible tools, bringing specialized expertise to underserved communities without requiring machine learning skills.
Additionally, incorporating human-in-the-loop AI strategies further enhances the effectiveness and reliability of these AI systems, ensuring that human oversight complements automated processes.
Impact on Accessibility and Inclusivity in AI
Multimodal AI makes technology more accessible across different sectors and populations. By processing text, images, and audio, AI becomes more intuitive for diverse users. In education and healthcare, these systems can simplify complex data for non-specialists, expanding AI's reach to communities that can benefit from its capabilities.
For people with disabilities, multimodal systems offer transformative alternatives for interaction. Someone with limited mobility might use voice commands alongside simple gestures, while those with visual impairments can receive audio descriptions of images.
Global reach expands as these systems overcome language barriers through visual context understanding. A farmer in a developing region might photograph a crop disease and receive treatment advice in their local language, even if detailed agricultural resources aren't available in that language.
Cultural inclusivity improves as multimodal systems recognize diverse expressions and contexts without requiring users to adapt to Western-centric interaction patterns.
Google's Vision-First Strategy With Gemini Models and Multimodal Implications
Google is prioritizing a "vision-first" approach, focusing on how we interact with AI systems. This shift moves beyond text-based interactions to incorporate images and videos.
Explanation of the Vision-First Approach
This approach makes AI more like human perception by integrating visual data with text, making AI more context-aware and capable of offering richer responses. "The future is going to be a really interesting vision-first moment for AI agents," Kilpatrick predicts.
Vision-first represents a fundamental shift in how information flows through AI systems. Rather than treating visual data as supplementary to text, Gemini processes visual information as a primary channel. This prioritization reflects how humans typically perceive the world—we see first, then contextualize with language.
The architectural implications are significant. Google designed Gemini's internal representations to handle visual data natively rather than converting it to text-compatible formats. This enables richer visual reasoning capabilities, allowing the model to understand spatial relationships, color patterns, and visual semantics with unprecedented sophistication.
This approach also changes how users interact with AI systems. Rather than describing what they see, users can simply show the AI and have a conversation about what they're both observing—much like how humans naturally interact when sharing visual experiences.
Role of Gemini Models in Enhancing Multimodal Capabilities
Gemini models drive this transformation with their unified architecture. Kilpatrick described them as "good enough to build for this agentic era." They seamlessly integrate various data types, handling everything from image detection to real-time processing.
Unlike previous approaches that used separate encoders for different modalities, Gemini processes all inputs through a unified transformer architecture, allowing cross-modal attention at every layer.
This deep integration enables more sophisticated reasoning across modalities. Gemini's training methodology breaks new ground by simultaneously training on aligned multimodal data at unprecedented scale, creating rich conceptual connections between what things look like, how they're described, and how they behave in videos.
The efficiency gains translate directly to practical benefits. Systems built on Gemini can process a customer's photographed product issue alongside their text description in a single inference pass, improving both accuracy and computational efficiency.
"These models are good enough to build for this agentic era," Kilpatrick asserts, highlighting how they seamlessly integrate various data types from image detection to real-time processing. By advancing multimodal AI, Gemini helps create systems that aren't just reactive but proactive, enhancing experiences across industries.
Future Directions for Multimodal AI
Multimodal AI continues to evolve, with key growth areas including scaling systems and improving infrastructure for advanced applications. Better infrastructure will make AI more efficient and cost-effective. Kilpatrick stressed the need for affordable AI: "The future requires models at the cost-effectiveness of Gemini's offerings."
Performance tracking will advance, helping AI integrate into various industries amid evolving regulations. It's essential to stay informed with insights on AI growth and regulation to navigate these changes.
The boundary between research and product development is fading, creating a synergy that propels innovation. To confidently explore these advancements, Galileo offers a platform for AI optimization and secure deployment.
The future of multimodal AI focuses not just on technological breakthroughs but on making AI accessible in everyday life. With Google and innovators like Galileo leading the way, AI promises to transform industries and empower people in unprecedented ways.
For more insights, listen to the rest of the conversation, where Bronsdon and Kilpatrick explore the story behind the making of Gemini 2.0. And check out other Chain of Thought episodes, where we break down complex Generative AI concepts into actionable strategies for software engineers and AI leaders.
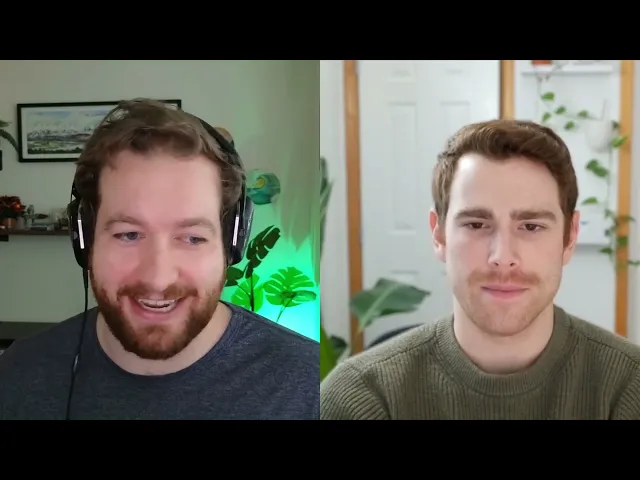
Multimodal AI combines text, images, audio, and video, giving AI multiple senses just like humans use sight, sound, and touch. In a Chain of Thought episode, Conor Bronsdon, Head of Developer Awareness at Galileo, explored the power of Multimodal AI with Logan Kilpatrick, a Senior Product Manager at Google DeepMind.
Google's Gemini was designed from scratch to handle multiple data types simultaneously, making machine intelligence more practical for real-world problems. This native multimodal design represents a significant shift in AI development.
The conversation explored how multimodal AI capabilities make AI smarter and more versatile, whether it's a virtual assistant that both sees and hears or healthcare systems analyzing medical texts alongside images.
Understanding Multimodal AI Capabilities
Multimodal AI functions like human intelligence by integrating multiple senses simultaneously. These systems combine different data forms to create a complete picture rather than fragmented insights, enabling better pattern recognition and problem-solving. To achieve this, teams employ evaluation techniques for multimodal AI to ensure optimal performance.
As Kilpatrick explained, "Think of multimodal AI as an intelligence that can see, listen, and read simultaneously." Just as we use multiple senses to understand our world, these AI systems combine different data forms for better decision-making.
Benefits of Integrating Multiple Modalities
Multimodal AI excels where single-mode systems fail by shifting seamlessly between different data formats. This approach reduces ambiguity in AI responses and provides contextual richness. When a system can simultaneously analyze a medical image and the patient's history, it delivers more nuanced, accurate insights than either modality could provide alone.
"It's about bringing intuitive and simplified user experiences into AI-enabled environments," Kilpatrick notes. By analyzing correlations across modalities, these systems can identify subtle patterns invisible within a single data type, thus enhancing performance and security.
However, teams must be cautious of phenomena like hallucinations in multimodal models, which can impact the reliability of AI outputs. In security applications, suspicious behavior might only become apparent when analyzing both visual footage and audio cues together.
Practical Applications of Multimodal AI
Multimodal AI is already transforming industries. Kilpatrick discusses how multimodal AI models excel in agentic AI systems that act on users' behalf, by processing multiple data types simultaneously. Their performance stands out on industry leaderboards for both capability and cost-efficiency.
In education, these systems create engaging environments where visuals, sounds, and narratives work together to boost understanding and retention.
Medical diagnostics has emerged as a particularly promising field. Radiologists using multimodal AI can simultaneously analyze X-ray images, patient medical histories, lab results, and related research papers, significantly improving diagnostic accuracy and treatment planning.
Customer service has been revolutionized by systems that understand both written complaints and uploaded images of defective products. This comprehensive understanding allows for more precise troubleshooting and faster resolution times.
E-commerce platforms now employ multimodal AI to enhance product discovery by understanding both text queries and visual preferences, creating more personalized shopping experiences that match functional needs and aesthetic preferences.
While these applications showcase the potential of multimodal AI, teams must navigate the challenges in building Multimodal LLMs to fully realize these benefits.
Democratizing AI with Multimodal Technology
Multimodal AI is breaking down barriers to entry. Traditional AI development required deep technical knowledge, but multimodal AI tools like Google's Gemini are changing that equation.
Reducing Dependence on Specialized ML Expertise
Building AI solutions previously demanded extensive machine learning expertise. Today's multimodal AI platforms simplify this process significantly, enabling developers to focus on solving business problems rather than wrestling with complex ML infrastructure.
"Gemini models enable developers and democratize access," Kilpatrick emphasizes. Google's integration of these tools into their platforms means small businesses can innovate without massive investments, fostering a broader range of AI applications.
The intuitive API design allows a restaurant owner with basic programming knowledge to build a system that analyzes food photos and reviews to identify trending preferences without hiring an ML specialist.
Pre-trained multimodal capabilities eliminate the need for extensive dataset collection and model training. Previously, creating even simple vision-language applications required separate datasets for each modality, specialized training workflows, and complex model fusion techniques—barriers that have largely disappeared with Gemini's unified approach.
Domain experts without AI backgrounds can now directly leverage their knowledge through these accessible tools, bringing specialized expertise to underserved communities without requiring machine learning skills.
Additionally, incorporating human-in-the-loop AI strategies further enhances the effectiveness and reliability of these AI systems, ensuring that human oversight complements automated processes.
Impact on Accessibility and Inclusivity in AI
Multimodal AI makes technology more accessible across different sectors and populations. By processing text, images, and audio, AI becomes more intuitive for diverse users. In education and healthcare, these systems can simplify complex data for non-specialists, expanding AI's reach to communities that can benefit from its capabilities.
For people with disabilities, multimodal systems offer transformative alternatives for interaction. Someone with limited mobility might use voice commands alongside simple gestures, while those with visual impairments can receive audio descriptions of images.
Global reach expands as these systems overcome language barriers through visual context understanding. A farmer in a developing region might photograph a crop disease and receive treatment advice in their local language, even if detailed agricultural resources aren't available in that language.
Cultural inclusivity improves as multimodal systems recognize diverse expressions and contexts without requiring users to adapt to Western-centric interaction patterns.
Google's Vision-First Strategy With Gemini Models and Multimodal Implications
Google is prioritizing a "vision-first" approach, focusing on how we interact with AI systems. This shift moves beyond text-based interactions to incorporate images and videos.
Explanation of the Vision-First Approach
This approach makes AI more like human perception by integrating visual data with text, making AI more context-aware and capable of offering richer responses. "The future is going to be a really interesting vision-first moment for AI agents," Kilpatrick predicts.
Vision-first represents a fundamental shift in how information flows through AI systems. Rather than treating visual data as supplementary to text, Gemini processes visual information as a primary channel. This prioritization reflects how humans typically perceive the world—we see first, then contextualize with language.
The architectural implications are significant. Google designed Gemini's internal representations to handle visual data natively rather than converting it to text-compatible formats. This enables richer visual reasoning capabilities, allowing the model to understand spatial relationships, color patterns, and visual semantics with unprecedented sophistication.
This approach also changes how users interact with AI systems. Rather than describing what they see, users can simply show the AI and have a conversation about what they're both observing—much like how humans naturally interact when sharing visual experiences.
Role of Gemini Models in Enhancing Multimodal Capabilities
Gemini models drive this transformation with their unified architecture. Kilpatrick described them as "good enough to build for this agentic era." They seamlessly integrate various data types, handling everything from image detection to real-time processing.
Unlike previous approaches that used separate encoders for different modalities, Gemini processes all inputs through a unified transformer architecture, allowing cross-modal attention at every layer.
This deep integration enables more sophisticated reasoning across modalities. Gemini's training methodology breaks new ground by simultaneously training on aligned multimodal data at unprecedented scale, creating rich conceptual connections between what things look like, how they're described, and how they behave in videos.
The efficiency gains translate directly to practical benefits. Systems built on Gemini can process a customer's photographed product issue alongside their text description in a single inference pass, improving both accuracy and computational efficiency.
"These models are good enough to build for this agentic era," Kilpatrick asserts, highlighting how they seamlessly integrate various data types from image detection to real-time processing. By advancing multimodal AI, Gemini helps create systems that aren't just reactive but proactive, enhancing experiences across industries.
Future Directions for Multimodal AI
Multimodal AI continues to evolve, with key growth areas including scaling systems and improving infrastructure for advanced applications. Better infrastructure will make AI more efficient and cost-effective. Kilpatrick stressed the need for affordable AI: "The future requires models at the cost-effectiveness of Gemini's offerings."
Performance tracking will advance, helping AI integrate into various industries amid evolving regulations. It's essential to stay informed with insights on AI growth and regulation to navigate these changes.
The boundary between research and product development is fading, creating a synergy that propels innovation. To confidently explore these advancements, Galileo offers a platform for AI optimization and secure deployment.
The future of multimodal AI focuses not just on technological breakthroughs but on making AI accessible in everyday life. With Google and innovators like Galileo leading the way, AI promises to transform industries and empower people in unprecedented ways.
For more insights, listen to the rest of the conversation, where Bronsdon and Kilpatrick explore the story behind the making of Gemini 2.0. And check out other Chain of Thought episodes, where we break down complex Generative AI concepts into actionable strategies for software engineers and AI leaders.
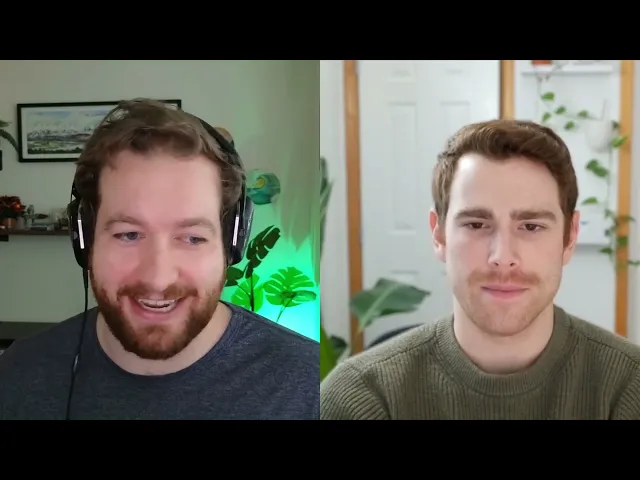
Multimodal AI combines text, images, audio, and video, giving AI multiple senses just like humans use sight, sound, and touch. In a Chain of Thought episode, Conor Bronsdon, Head of Developer Awareness at Galileo, explored the power of Multimodal AI with Logan Kilpatrick, a Senior Product Manager at Google DeepMind.
Google's Gemini was designed from scratch to handle multiple data types simultaneously, making machine intelligence more practical for real-world problems. This native multimodal design represents a significant shift in AI development.
The conversation explored how multimodal AI capabilities make AI smarter and more versatile, whether it's a virtual assistant that both sees and hears or healthcare systems analyzing medical texts alongside images.
Understanding Multimodal AI Capabilities
Multimodal AI functions like human intelligence by integrating multiple senses simultaneously. These systems combine different data forms to create a complete picture rather than fragmented insights, enabling better pattern recognition and problem-solving. To achieve this, teams employ evaluation techniques for multimodal AI to ensure optimal performance.
As Kilpatrick explained, "Think of multimodal AI as an intelligence that can see, listen, and read simultaneously." Just as we use multiple senses to understand our world, these AI systems combine different data forms for better decision-making.
Benefits of Integrating Multiple Modalities
Multimodal AI excels where single-mode systems fail by shifting seamlessly between different data formats. This approach reduces ambiguity in AI responses and provides contextual richness. When a system can simultaneously analyze a medical image and the patient's history, it delivers more nuanced, accurate insights than either modality could provide alone.
"It's about bringing intuitive and simplified user experiences into AI-enabled environments," Kilpatrick notes. By analyzing correlations across modalities, these systems can identify subtle patterns invisible within a single data type, thus enhancing performance and security.
However, teams must be cautious of phenomena like hallucinations in multimodal models, which can impact the reliability of AI outputs. In security applications, suspicious behavior might only become apparent when analyzing both visual footage and audio cues together.
Practical Applications of Multimodal AI
Multimodal AI is already transforming industries. Kilpatrick discusses how multimodal AI models excel in agentic AI systems that act on users' behalf, by processing multiple data types simultaneously. Their performance stands out on industry leaderboards for both capability and cost-efficiency.
In education, these systems create engaging environments where visuals, sounds, and narratives work together to boost understanding and retention.
Medical diagnostics has emerged as a particularly promising field. Radiologists using multimodal AI can simultaneously analyze X-ray images, patient medical histories, lab results, and related research papers, significantly improving diagnostic accuracy and treatment planning.
Customer service has been revolutionized by systems that understand both written complaints and uploaded images of defective products. This comprehensive understanding allows for more precise troubleshooting and faster resolution times.
E-commerce platforms now employ multimodal AI to enhance product discovery by understanding both text queries and visual preferences, creating more personalized shopping experiences that match functional needs and aesthetic preferences.
While these applications showcase the potential of multimodal AI, teams must navigate the challenges in building Multimodal LLMs to fully realize these benefits.
Democratizing AI with Multimodal Technology
Multimodal AI is breaking down barriers to entry. Traditional AI development required deep technical knowledge, but multimodal AI tools like Google's Gemini are changing that equation.
Reducing Dependence on Specialized ML Expertise
Building AI solutions previously demanded extensive machine learning expertise. Today's multimodal AI platforms simplify this process significantly, enabling developers to focus on solving business problems rather than wrestling with complex ML infrastructure.
"Gemini models enable developers and democratize access," Kilpatrick emphasizes. Google's integration of these tools into their platforms means small businesses can innovate without massive investments, fostering a broader range of AI applications.
The intuitive API design allows a restaurant owner with basic programming knowledge to build a system that analyzes food photos and reviews to identify trending preferences without hiring an ML specialist.
Pre-trained multimodal capabilities eliminate the need for extensive dataset collection and model training. Previously, creating even simple vision-language applications required separate datasets for each modality, specialized training workflows, and complex model fusion techniques—barriers that have largely disappeared with Gemini's unified approach.
Domain experts without AI backgrounds can now directly leverage their knowledge through these accessible tools, bringing specialized expertise to underserved communities without requiring machine learning skills.
Additionally, incorporating human-in-the-loop AI strategies further enhances the effectiveness and reliability of these AI systems, ensuring that human oversight complements automated processes.
Impact on Accessibility and Inclusivity in AI
Multimodal AI makes technology more accessible across different sectors and populations. By processing text, images, and audio, AI becomes more intuitive for diverse users. In education and healthcare, these systems can simplify complex data for non-specialists, expanding AI's reach to communities that can benefit from its capabilities.
For people with disabilities, multimodal systems offer transformative alternatives for interaction. Someone with limited mobility might use voice commands alongside simple gestures, while those with visual impairments can receive audio descriptions of images.
Global reach expands as these systems overcome language barriers through visual context understanding. A farmer in a developing region might photograph a crop disease and receive treatment advice in their local language, even if detailed agricultural resources aren't available in that language.
Cultural inclusivity improves as multimodal systems recognize diverse expressions and contexts without requiring users to adapt to Western-centric interaction patterns.
Google's Vision-First Strategy With Gemini Models and Multimodal Implications
Google is prioritizing a "vision-first" approach, focusing on how we interact with AI systems. This shift moves beyond text-based interactions to incorporate images and videos.
Explanation of the Vision-First Approach
This approach makes AI more like human perception by integrating visual data with text, making AI more context-aware and capable of offering richer responses. "The future is going to be a really interesting vision-first moment for AI agents," Kilpatrick predicts.
Vision-first represents a fundamental shift in how information flows through AI systems. Rather than treating visual data as supplementary to text, Gemini processes visual information as a primary channel. This prioritization reflects how humans typically perceive the world—we see first, then contextualize with language.
The architectural implications are significant. Google designed Gemini's internal representations to handle visual data natively rather than converting it to text-compatible formats. This enables richer visual reasoning capabilities, allowing the model to understand spatial relationships, color patterns, and visual semantics with unprecedented sophistication.
This approach also changes how users interact with AI systems. Rather than describing what they see, users can simply show the AI and have a conversation about what they're both observing—much like how humans naturally interact when sharing visual experiences.
Role of Gemini Models in Enhancing Multimodal Capabilities
Gemini models drive this transformation with their unified architecture. Kilpatrick described them as "good enough to build for this agentic era." They seamlessly integrate various data types, handling everything from image detection to real-time processing.
Unlike previous approaches that used separate encoders for different modalities, Gemini processes all inputs through a unified transformer architecture, allowing cross-modal attention at every layer.
This deep integration enables more sophisticated reasoning across modalities. Gemini's training methodology breaks new ground by simultaneously training on aligned multimodal data at unprecedented scale, creating rich conceptual connections between what things look like, how they're described, and how they behave in videos.
The efficiency gains translate directly to practical benefits. Systems built on Gemini can process a customer's photographed product issue alongside their text description in a single inference pass, improving both accuracy and computational efficiency.
"These models are good enough to build for this agentic era," Kilpatrick asserts, highlighting how they seamlessly integrate various data types from image detection to real-time processing. By advancing multimodal AI, Gemini helps create systems that aren't just reactive but proactive, enhancing experiences across industries.
Future Directions for Multimodal AI
Multimodal AI continues to evolve, with key growth areas including scaling systems and improving infrastructure for advanced applications. Better infrastructure will make AI more efficient and cost-effective. Kilpatrick stressed the need for affordable AI: "The future requires models at the cost-effectiveness of Gemini's offerings."
Performance tracking will advance, helping AI integrate into various industries amid evolving regulations. It's essential to stay informed with insights on AI growth and regulation to navigate these changes.
The boundary between research and product development is fading, creating a synergy that propels innovation. To confidently explore these advancements, Galileo offers a platform for AI optimization and secure deployment.
The future of multimodal AI focuses not just on technological breakthroughs but on making AI accessible in everyday life. With Google and innovators like Galileo leading the way, AI promises to transform industries and empower people in unprecedented ways.
For more insights, listen to the rest of the conversation, where Bronsdon and Kilpatrick explore the story behind the making of Gemini 2.0. And check out other Chain of Thought episodes, where we break down complex Generative AI concepts into actionable strategies for software engineers and AI leaders.
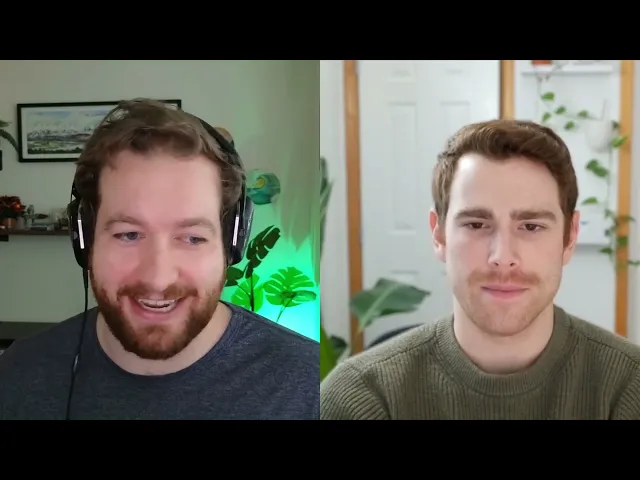
Multimodal AI combines text, images, audio, and video, giving AI multiple senses just like humans use sight, sound, and touch. In a Chain of Thought episode, Conor Bronsdon, Head of Developer Awareness at Galileo, explored the power of Multimodal AI with Logan Kilpatrick, a Senior Product Manager at Google DeepMind.
Google's Gemini was designed from scratch to handle multiple data types simultaneously, making machine intelligence more practical for real-world problems. This native multimodal design represents a significant shift in AI development.
The conversation explored how multimodal AI capabilities make AI smarter and more versatile, whether it's a virtual assistant that both sees and hears or healthcare systems analyzing medical texts alongside images.
Understanding Multimodal AI Capabilities
Multimodal AI functions like human intelligence by integrating multiple senses simultaneously. These systems combine different data forms to create a complete picture rather than fragmented insights, enabling better pattern recognition and problem-solving. To achieve this, teams employ evaluation techniques for multimodal AI to ensure optimal performance.
As Kilpatrick explained, "Think of multimodal AI as an intelligence that can see, listen, and read simultaneously." Just as we use multiple senses to understand our world, these AI systems combine different data forms for better decision-making.
Benefits of Integrating Multiple Modalities
Multimodal AI excels where single-mode systems fail by shifting seamlessly between different data formats. This approach reduces ambiguity in AI responses and provides contextual richness. When a system can simultaneously analyze a medical image and the patient's history, it delivers more nuanced, accurate insights than either modality could provide alone.
"It's about bringing intuitive and simplified user experiences into AI-enabled environments," Kilpatrick notes. By analyzing correlations across modalities, these systems can identify subtle patterns invisible within a single data type, thus enhancing performance and security.
However, teams must be cautious of phenomena like hallucinations in multimodal models, which can impact the reliability of AI outputs. In security applications, suspicious behavior might only become apparent when analyzing both visual footage and audio cues together.
Practical Applications of Multimodal AI
Multimodal AI is already transforming industries. Kilpatrick discusses how multimodal AI models excel in agentic AI systems that act on users' behalf, by processing multiple data types simultaneously. Their performance stands out on industry leaderboards for both capability and cost-efficiency.
In education, these systems create engaging environments where visuals, sounds, and narratives work together to boost understanding and retention.
Medical diagnostics has emerged as a particularly promising field. Radiologists using multimodal AI can simultaneously analyze X-ray images, patient medical histories, lab results, and related research papers, significantly improving diagnostic accuracy and treatment planning.
Customer service has been revolutionized by systems that understand both written complaints and uploaded images of defective products. This comprehensive understanding allows for more precise troubleshooting and faster resolution times.
E-commerce platforms now employ multimodal AI to enhance product discovery by understanding both text queries and visual preferences, creating more personalized shopping experiences that match functional needs and aesthetic preferences.
While these applications showcase the potential of multimodal AI, teams must navigate the challenges in building Multimodal LLMs to fully realize these benefits.
Democratizing AI with Multimodal Technology
Multimodal AI is breaking down barriers to entry. Traditional AI development required deep technical knowledge, but multimodal AI tools like Google's Gemini are changing that equation.
Reducing Dependence on Specialized ML Expertise
Building AI solutions previously demanded extensive machine learning expertise. Today's multimodal AI platforms simplify this process significantly, enabling developers to focus on solving business problems rather than wrestling with complex ML infrastructure.
"Gemini models enable developers and democratize access," Kilpatrick emphasizes. Google's integration of these tools into their platforms means small businesses can innovate without massive investments, fostering a broader range of AI applications.
The intuitive API design allows a restaurant owner with basic programming knowledge to build a system that analyzes food photos and reviews to identify trending preferences without hiring an ML specialist.
Pre-trained multimodal capabilities eliminate the need for extensive dataset collection and model training. Previously, creating even simple vision-language applications required separate datasets for each modality, specialized training workflows, and complex model fusion techniques—barriers that have largely disappeared with Gemini's unified approach.
Domain experts without AI backgrounds can now directly leverage their knowledge through these accessible tools, bringing specialized expertise to underserved communities without requiring machine learning skills.
Additionally, incorporating human-in-the-loop AI strategies further enhances the effectiveness and reliability of these AI systems, ensuring that human oversight complements automated processes.
Impact on Accessibility and Inclusivity in AI
Multimodal AI makes technology more accessible across different sectors and populations. By processing text, images, and audio, AI becomes more intuitive for diverse users. In education and healthcare, these systems can simplify complex data for non-specialists, expanding AI's reach to communities that can benefit from its capabilities.
For people with disabilities, multimodal systems offer transformative alternatives for interaction. Someone with limited mobility might use voice commands alongside simple gestures, while those with visual impairments can receive audio descriptions of images.
Global reach expands as these systems overcome language barriers through visual context understanding. A farmer in a developing region might photograph a crop disease and receive treatment advice in their local language, even if detailed agricultural resources aren't available in that language.
Cultural inclusivity improves as multimodal systems recognize diverse expressions and contexts without requiring users to adapt to Western-centric interaction patterns.
Google's Vision-First Strategy With Gemini Models and Multimodal Implications
Google is prioritizing a "vision-first" approach, focusing on how we interact with AI systems. This shift moves beyond text-based interactions to incorporate images and videos.
Explanation of the Vision-First Approach
This approach makes AI more like human perception by integrating visual data with text, making AI more context-aware and capable of offering richer responses. "The future is going to be a really interesting vision-first moment for AI agents," Kilpatrick predicts.
Vision-first represents a fundamental shift in how information flows through AI systems. Rather than treating visual data as supplementary to text, Gemini processes visual information as a primary channel. This prioritization reflects how humans typically perceive the world—we see first, then contextualize with language.
The architectural implications are significant. Google designed Gemini's internal representations to handle visual data natively rather than converting it to text-compatible formats. This enables richer visual reasoning capabilities, allowing the model to understand spatial relationships, color patterns, and visual semantics with unprecedented sophistication.
This approach also changes how users interact with AI systems. Rather than describing what they see, users can simply show the AI and have a conversation about what they're both observing—much like how humans naturally interact when sharing visual experiences.
Role of Gemini Models in Enhancing Multimodal Capabilities
Gemini models drive this transformation with their unified architecture. Kilpatrick described them as "good enough to build for this agentic era." They seamlessly integrate various data types, handling everything from image detection to real-time processing.
Unlike previous approaches that used separate encoders for different modalities, Gemini processes all inputs through a unified transformer architecture, allowing cross-modal attention at every layer.
This deep integration enables more sophisticated reasoning across modalities. Gemini's training methodology breaks new ground by simultaneously training on aligned multimodal data at unprecedented scale, creating rich conceptual connections between what things look like, how they're described, and how they behave in videos.
The efficiency gains translate directly to practical benefits. Systems built on Gemini can process a customer's photographed product issue alongside their text description in a single inference pass, improving both accuracy and computational efficiency.
"These models are good enough to build for this agentic era," Kilpatrick asserts, highlighting how they seamlessly integrate various data types from image detection to real-time processing. By advancing multimodal AI, Gemini helps create systems that aren't just reactive but proactive, enhancing experiences across industries.
Future Directions for Multimodal AI
Multimodal AI continues to evolve, with key growth areas including scaling systems and improving infrastructure for advanced applications. Better infrastructure will make AI more efficient and cost-effective. Kilpatrick stressed the need for affordable AI: "The future requires models at the cost-effectiveness of Gemini's offerings."
Performance tracking will advance, helping AI integrate into various industries amid evolving regulations. It's essential to stay informed with insights on AI growth and regulation to navigate these changes.
The boundary between research and product development is fading, creating a synergy that propels innovation. To confidently explore these advancements, Galileo offers a platform for AI optimization and secure deployment.
The future of multimodal AI focuses not just on technological breakthroughs but on making AI accessible in everyday life. With Google and innovators like Galileo leading the way, AI promises to transform industries and empower people in unprecedented ways.
For more insights, listen to the rest of the conversation, where Bronsdon and Kilpatrick explore the story behind the making of Gemini 2.0. And check out other Chain of Thought episodes, where we break down complex Generative AI concepts into actionable strategies for software engineers and AI leaders.